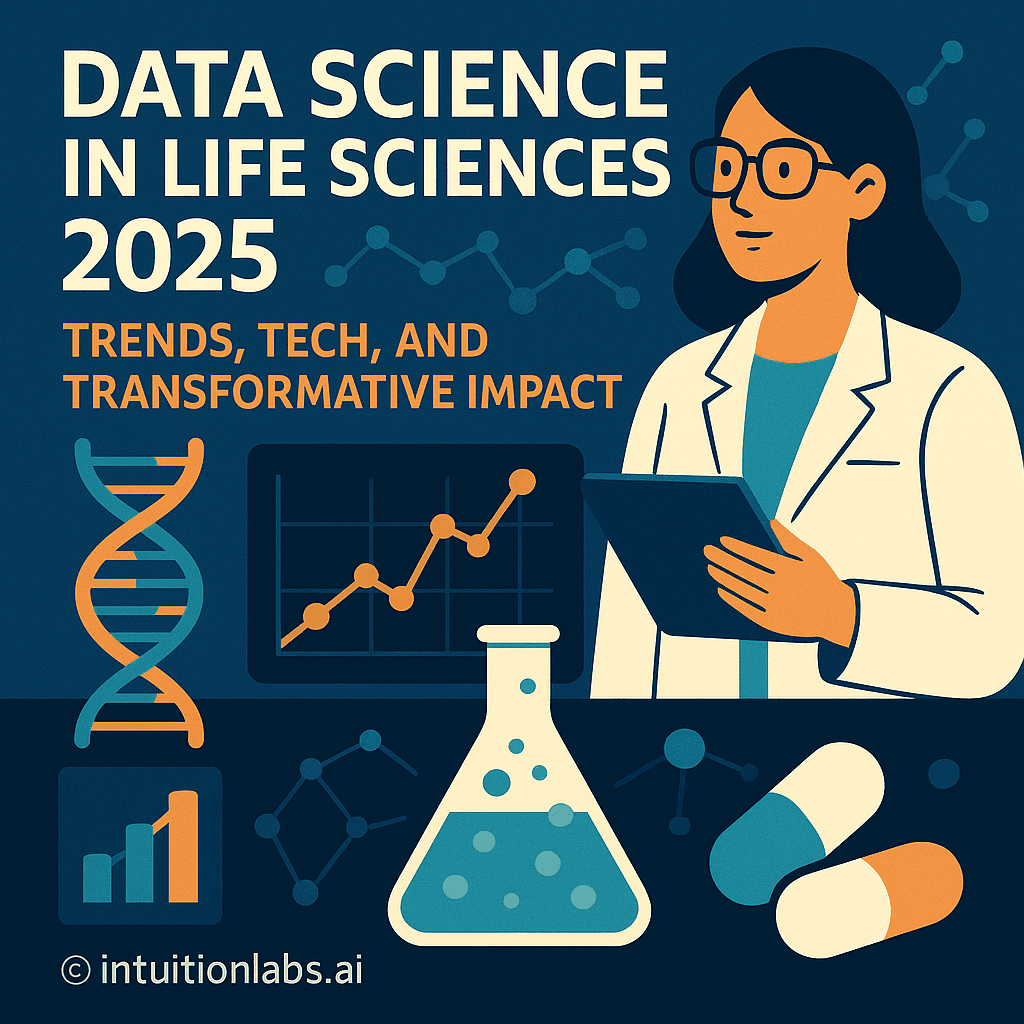
Data Science in Life Sciences 2025: Trends, Tech, and Transformative Impact
The life sciences industry – especially pharmaceutical research and development – is undergoing a data-driven transformation as of 2025. Advances in artificial intelligence (AI), big data analytics, natural language processing (NLP), and cloud computing are converging to reshape how new therapies are discovered, how clinical trials are run, and how personalized medicine is delivered. Companies across the U.S. pharma sector are heavily investing in data science capabilities: in one recent survey, 93% of life sciences executives plan to increase investments in data, digital, and AI in 2025 (2025 AI Trends: Life Sciences Leaders on Data, Digital and AI - ZS). This comprehensive overview will examine the current state of data science in life sciences, covering emerging technologies, real-world applications (from drug discovery and clinical trials to genomics and regulatory compliance), workforce and data governance trends, interoperability challenges, and evolving FDA/EMA expectations – with a focus on the U.S. context and the latest 2025 data and case studies.
Emerging Technologies Powering Data Science in Pharma
Modern pharmaceutical data science is fueled by a suite of powerful technologies. Key among them are AI and machine learning, which have matured from experimental pilot projects to mainstream tools across R&D and operations. Indeed, analysts estimate AI could create $350–$410 billion in annual value for the pharma industry by 2025 (What will be the key trends in AI innovation in the Pharmaceutical Industry in 2025?), driven by innovations in drug development, clinical trials, precision medicine, and even commercial operations. Unlike earlier decades where AI's impact was incremental, today's sophisticated algorithms – combined with large-scale data integration and cloud computing – are overcoming past limitations (such as fragmented data and slow processing) (How data science and AI will transform life sciences in 2025 - pharmaphorum). This means AI/ML is now hitting full stride in life sciences: companies can process massive datasets, detect hidden patterns, and make predictions at a speed and scale far beyond human capability (How data science and AI will transform life sciences in 2025 - pharmaphorum). For example, generative AI (which includes large language models and generative chemistry models) is seen as having transformative potential across the value chain (2025 life sciences outlook - Deloitte Insights). Life science organizations report that by embedding generative AI into workflows, they can reduce R&D costs, streamline operations, and boost productivity (2025 life sciences outlook - Deloitte Insights). One Deloitte analysis found that broad AI adoption could yield up to 11% of revenue in added value for biopharma companies and as much as 12% cost savings for medtech within a few years (2025 life sciences outlook - Deloitte Insights). These gains are motivating significant investment – about 60% of pharma executives are closely tracking AI-driven digital transformation and moving beyond pilots to scale these technologies enterprise-wide (2025 life sciences outlook - Deloitte Insights).
Another foundational technology is big data analytics. The pharma and biotech sector now collects unprecedented volumes of data from diverse sources: high-throughput screening assays, next-generation sequencing for genomics, electronic health records (EHRs) and real-world patient data, wearables and sensors, and more. Managing this deluge is non-trivial – the NIH estimates that storing all global genome sequencing data generated by 2025 will require on the order of exabytes of storage (Genomics Market Size To Hit USD 157.47 Billion By 2033 - BioSpace). In fact, over 100 million human genomes are expected to be sequenced by 2025 as part of genomic initiatives, each yielding hundreds of gigabytes of raw data (Genomics Market Size To Hit USD 157.47 Billion By 2033 - BioSpace). Pharma companies are leveraging advanced analytics and cloud-based data lakes to derive insights from this "data tsunami." By applying machine learning to massive datasets, researchers can spot patterns (for example, genetic variants associated with disease or biomarkers predictive of drug response) that would be impossible to discern manually. Real-world evidence (RWE) has become especially important – more than half of pharma organizations say they are prioritizing real-world and multimodal data capabilities (integrating clinical, genomic, and patient-reported data) (2025 life sciences outlook - Deloitte Insights). However, many still struggle to fully realize this vision; only ~21% consider RWE a "very important" priority, and capabilities gaps persist in analytics infrastructure and data science talent to gather, standardize, and make multi-source data accessible (2025 life sciences outlook - Deloitte Insights). Closing these gaps is a focus for 2025, as firms recognize that harnessing big data is critical for competitive advantage.
Natural language processing (NLP) – particularly the use of large language models (LLMs) – is another game-changer for pharma data science. A vast portion of biomedical information is stored in unstructured text: scientific publications, clinical trial protocols, regulatory filings, physician notes, adverse event reports, etc. In 2025, NLP tools are being deployed to unlock insights from this textual data and to automate labor-intensive documentation tasks. For instance, pharma organizations are using LLMs to draft and review clinical trial reports and regulatory submission documents, which speeds up processes while maintaining accuracy (How data science and AI will transform life sciences in 2025 - pharmaphorum). Large language models can now handle specialised medical terminology and perform compliance checks on documents, simplifying complex jargon into clearer language (How data science and AI will transform life sciences in 2025 - pharmaphorum). Industry giants like IBM and Microsoft have integrated LLMs into healthcare documentation workflows (How data science and AI will transform life sciences in 2025 - pharmaphorum), and companies are even automating authorship of clinical study reports by pulling data directly from clinical databases and statistical analysis systems (How data science and AI will transform life sciences in 2025 - pharmaphorum). This trend is expected to accelerate – by eliminating bottlenecks in report writing and data abstraction, NLP helps new therapies reach patients faster and reduces administrative burden. Beyond documentation, NLP algorithms are also scanning medical literature and real-world data sources to find signals (e.g. identifying new drug indications or flagging safety concerns from physician notes), tasks that would overwhelm human reviewers.
Finally, cloud computing and infrastructure underpins nearly all these data-driven advances. Pharma was once cautious about the cloud due to regulatory and security concerns, but by 2025 cloud adoption is mainstream. Modern drug discovery and development generate huge computational workloads – from simulating protein folding to analyzing multi-terabyte genomic cohorts – which cloud platforms can handle with scalable computing power. Nearly all companies (estimated 96% across industries) utilize public cloud services by 2025 (Cloud Computing Stats 2025 - NextWork), and pharma is no exception. Cloud-based data warehouses and high-performance computing environments allow global R&D teams to collaborate in real time, accessing shared datasets and AI tools regardless of location. Major cloud providers (AWS, Azure, Google Cloud) have created life science–specific services and compliance certifications to meet pharma needs (for example, GxP-qualified cloud environments for regulated data). The result is that even highly regulated workloads like clinical trial data management or pharmacovigilance are now often cloud-hosted. Cloud elasticity accelerates AI/ML experimentation – data scientists can train models on GPU clusters in hours instead of waiting in queue for limited on-premise servers. Moreover, cloud architectures facilitate data interoperability, enabling organizations to integrate data from various sources (internal silos, partner organizations, healthcare providers) into unified platforms. In sum, cloud computing provides the backbone for pharma's big data pipelines and AI initiatives, offering the storage, processing, and connectivity required to turn raw data into actionable knowledge.
Applications Across the Drug Lifecycle
Emerging technologies are not being adopted for their own sake – they are enabling real-world applications that are revolutionizing each stage of the drug lifecycle, from early discovery to post-market. Below we explore how data science is driving breakthroughs in key domains: drug discovery, clinical trials, genomics and personalized medicine, and regulatory compliance.
Accelerating Drug Discovery and Preclinical Research
Perhaps the most profound impact of AI and data science in pharma is in drug discovery. Traditional drug R&D is notoriously slow and costly – identifying a viable drug candidate can take years of lab experiments and screening thousands of compounds, with failure rates around 90%. In 2025, data science approaches are radically speeding up this process. Machine learning models can sift through enormous chemical libraries and predict which molecules are most likely to bind to a given biological target, focusing wet-lab efforts on the most promising candidates. In silico techniques now allow much of the design and testing of new compounds to occur virtually. As one industry expert put it, "the integration of data science in drug discovery is evolving beyond mere trial phases to practical uses – especially in the creation and testing of molecular compounds in silico." Algorithms simulating biological interactions can significantly accelerate drug discovery, improving the efficiency of initial testing and opening doors to novel therapies (How data science and AI will transform life sciences in 2025 - pharmaphorum).
A striking example is the advent of generative AI for drug design. Generative models (such as GANs and transformer-based models) are used to create new molecular structures with desired properties, essentially "imagining" new drug candidates. In 2024, biotech startup Insilico Medicine announced that its AI-designed drug for fibrosis (targeting a novel pathway) successfully advanced to human trials – the first AI-discovered and AI-generated drug to do so (Insilico Medicine unveils first AI-generated and AI-discovered drug in new paper - VentureBeat). Insilico's system used AI to identify a biological target and then generatively designed a molecule to hit that target; remarkably, the compound (INS018_055) reached Phase I clinical trials in under 30 months, compared to an estimated 6+ years and hundreds of millions of dollars via conventional approaches (Insilico Medicine unveils first AI-generated and AI-discovered drug in new paper - VentureBeat). By early 2024 it had even progressed into Phase II, demonstrating safety and preliminary efficacy (Insilico Medicine unveils first AI-generated and AI-discovered drug in new paper - VentureBeat). This proof-of-concept shows that AI can drastically compress early drug development timelines and costs. Other AI-driven drug discovery companies like Exscientia, Atomwise, and Recursion have similarly moved candidates into preclinical or clinical stages, often in partnership with major pharma firms. For instance, Exscientia (in collaboration with a large Japanese pharma) brought an AI-designed molecule for obsessive-compulsive disorder to Phase I trials in under 12 months back in 2020. As generative chemistry and deep learning models continue to improve, we can expect an expanding pipeline of AI-derived compounds tackling various diseases.
Beyond generating new molecules, data science is optimizing many facets of preclinical research. Computational biology and bioinformatics methods analyze vast genomic and proteomic datasets to uncover drug targets and disease mechanisms. High-dimensional data from omics experiments (genomics, transcriptomics, proteomics, metabolomics) can be mined with machine learning to identify patterns – for example, an algorithm might find a gene expression signature that differentiates patients who respond to a drug from those who don't, guiding the selection of a target or biomarker. Digital twin technology is also emerging: companies like Sanofi have begun using "virtual patients" or disease simulations as testing grounds for drug candidates (2025 life sciences outlook - Deloitte Insights). These digital twins are computational models that replicate human physiology or specific patient subpopulations, allowing researchers to experiment on the virtual twin to predict how a real patient might respond (2025 life sciences outlook - Deloitte Insights). Sanofi reported using digital twins in early development to test novel drug candidates, helping determine potential effectiveness before ever going into a real patient (2025 life sciences outlook - Deloitte Insights). Coupled with AI-driven predictive modeling, this has enabled R&D cycle times to shrink from weeks to hours in some cases (2025 life sciences outlook - Deloitte Insights). In summary, from target discovery (finding the right biology to hit) to lead optimization (tweaking chemical structures) and preclinical testing, data science techniques are injecting unprecedented speed and precision into drug discovery. This is critically important as pharma faces pressure to fill pipelines faster and more efficiently – such as the looming "patent cliff" of expiring drug patents by 2030 – and innovation through AI is a key strategy to boost R&D productivity (2025 life sciences outlook - Deloitte Insights).
Transforming Clinical Trials with Data Analytics and AI
The clinical trial phase of drug development – testing new treatments in human volunteers – is another area being transformed by data science in 2025. Clinical trials are expensive and time-consuming; recruiting patients, administering the study, collecting and analyzing data, and ensuring compliance can take several years for each phase. Now, AI and advanced analytics are helping to streamline clinical trials and improve their success rates.
One immediate impact is in patient recruitment and trial design. Finding enough eligible patients for a trial (who meet complex inclusion criteria) is often a bottleneck that leads to delays or even trial failure. Data science offers a solution: machine learning algorithms can mine electronic health records and other patient databases to identify candidates who match a trial's criteria in real-time. AI is expected to greatly accelerate trial recruitment in 2025 by intelligently matching patients to trials (How data science and AI will transform life sciences in 2025 - pharmaphorum). As an example, AI-powered platforms are already being used to scan hospital EHR systems for patients with specific genetic markers or disease characteristics, then alert physicians or patients about relevant trial opportunities. Pharmas report that such tools have significantly reduced recruitment timelines by increasing the precision of patient matching (How data science and AI will transform life sciences in 2025 - pharmaphorum). Over the next year, this trend is set to continue, enabling trials to enroll participants faster and get underway without the traditional months (or years) of site-by-site recruitment efforts. Faster recruitment not only cuts costs but also brings life-saving therapies to market sooner by eliminating needless waiting.
Data science is also optimizing trial design and execution. AI models can simulate trial outcomes under various scenarios (sometimes called in silico trials or trial digital twins) to choose optimal study parameters – such as determining the best inclusion criteria, endpoints, or dosing regimen that would demonstrate a drug's effect most efficiently. Adaptive trial designs, which adjust based on interim data, rely on real-time analytics to decide if a study arm should be modified or stopped early due to clear success or futility. Advanced analytics on streaming trial data (including data from remote wearables or biosensors in decentralized trials) can alert sponsors to safety signals or data quality issues earlier than traditional monitoring. In fact, the proliferation of decentralized clinical trials (DCTs) – where patients may participate from home using digital devices – has led to big data streams of continuous patient information (heart rate, activity, etc.) that require AI to interpret. Machine learning is employed to separate meaningful signals from noise in this deluge of patient data, helping researchers glean insights on drug efficacy or side effects in real time.
The benefits of AI in trials are potentially enormous. According to some research, AI-driven efficiencies could reduce clinical trial costs by up to 70% and shorten trial timelines by ~80% (What will be the key trends in AI innovation in the Pharmaceutical Industry in 2025?). Even if these figures are optimistic, they underscore how impactful AI can be: by reducing protocol amendments, avoiding unnecessary control patients, or predicting outcomes that allow early trial termination, huge savings in time and money are possible. Moreover, synthetic control arms and use of real-world data are emerging as supplements to traditional trials. For instance, instead of recruiting a full placebo group, a trial might use historical patient data (carefully matched via analytics) as an external control, thus needing fewer new participants. Regulators like the FDA have shown openness to such approaches in certain cases, especially for rare diseases or oncology when ethical or practical concerns make placebo trials difficult. All of this is enabled by robust data science techniques ensuring that real-world data is comparable and credible.
In practical terms, many pharma companies and research organizations are deploying AI solutions to manage trial operations. Examples include: natural language processing to automatically screen clinical notes or prior studies to inform trial design; ML-based tools to predict patient dropout risk so investigators can intervene and improve retention; and computer vision AI to analyze medical images (like tumor scans) as trial endpoints more objectively. Indeed, AI has demonstrated remarkable proficiency in medical image analysis, often exceeding human accuracy in detecting subtle changes (How data science and AI will transform life sciences in 2025 - pharmaphorum). In trials, this means faster and more consistent reading of outcomes such as tumor response or disease progression on imaging, which can be critical for oncology studies. All told, the clinical trials of 2025 are increasingly "digital" and data-driven, leveraging advanced analytics at every stage. Pharmaceutical companies that adopt these approaches can run leaner, more adaptive studies – potentially getting drugs approved with fewer hurdles. And for patients, this translates to faster access to innovative treatments and a trial experience that may be more efficient and personalized (for example, using data to ensure they're only in trials they are likely to benefit from).
Genomics and Personalized Medicine
Data science is also at the heart of the revolution in genomics and personalized medicine. In the two decades since the Human Genome Project, DNA sequencing has become exponentially cheaper and faster, leading to an explosion of genomic data. By 2025, genomic analysis is a routine part of both research and clinical care, and the integration of genomic data with other health data – powered by advanced analytics – is enabling truly personalized treatments.
The sheer scale of genomic data is staggering. As noted, tens of millions of human genomes have been sequenced globally, generating tens of billions of gigabytes of data (Genomics Market Size To Hit USD 157.47 Billion By 2033 - BioSpace). Within each genome lie millions of genetic variants; making sense of which variants are relevant to health and disease is a classic big data challenge. This is where data science comes in: AI and machine learning are invaluable for interpreting genomic data. For instance, ML models can be trained to predict whether a given DNA mutation is likely benign or disease-causing (a critical task in genetic diagnostics). In drug discovery, analyzing genomic and transcriptomic datasets with AI can reveal new drug targets – e.g., identifying a gene that is dysregulated in a disease but could be druggable.
One major use of genomic data is in precision medicine, which tailors medical treatment to the individual characteristics of each patient. AI tools can synthesize a patient's genetic profile, clinical history, and even environmental/lifestyle factors to recommend the optimal therapy. Oncology has been a pioneer in this area: cancer treatment now commonly involves genomic testing of the tumor to guide targeted therapies. By 2025, machine learning algorithms are often used to identify the specific DNA mutations or expression patterns in a tumor that indicate which drug will be most effective. For example, AI can help match cancer patients to clinical trials or approved therapies based on multi-gene biomarkers that would be impossible to evaluate manually. As a result, patients receive more effective, customized treatment plans, improving outcomes. In fact, AI in precision medicine is increasingly adept at handling this complexity – analysing a patient's genome alongside other clinical data to recommend personalized treatment – ensuring patients get the therapies most likely to benefit them while avoiding unnecessary treatments (What will be the key trends in AI innovation in the Pharmaceutical Industry in 2025?). In oncology specifically, AI has been used to optimize drug dosing and identify promising drug combinations tailored to a patient's unique genetic profile (What will be the key trends in AI innovation in the Pharmaceutical Industry in 2025?).
Beyond cancer, genomic data science is impacting a range of areas: from rare genetic disorders (where genome sequencing can pinpoint a causative mutation and suggest a targeted therapy or clinical trial) to pharmacogenomics (where AI helps predict how patients of different genotypes will metabolize a drug, allowing dose adjustments). Fields like gene therapy and CRISPR-based gene editing also rely on big data analytics – designing a CRISPR edit or evaluating off-target effects involve large-scale computations over genomic sequences. Multi-omics data integration is an emerging frontier: researchers are combining genomics with proteomics (proteins), metabolomics (metabolites), microbiome data, etc., to get a comprehensive view of human biology. Handling these rich datasets demands sophisticated data platforms and ML to find correlations (for example, linking a genetic variant to a change in a protein level that causes a disease phenotype).
Notably, national biobank projects and large population genomics initiatives are providing treasure troves of data for discovery. The U.S. All of Us Research Program, for instance, aims to sequence one million Americans and track their health data. Data scientists are mining these large cohorts to uncover new genotype–phenotype associations – such as genetic risk factors for common diseases and potential drug targets (some pharma companies have partnerships to access UK Biobank and similar resources). According to industry reports, five countries' biobanks will collectively cover ~15% of their populations by 2025 (Biobanks to reach 15% coverage in five nations by 2025), accelerating precision medicine research.
Yet, with great data comes great responsibility: handling sensitive genomic data raises privacy and data governance challenges (discussed later). Also, making genomic insights clinically actionable requires careful validation and often regulatory approval (e.g., companion diagnostics for drugs). Overall, data science is the engine that converts raw genomic data into medical breakthroughs – enabling early disease prediction (polygenic risk scores), individualized therapy selection, and a deeper understanding of human biology that will drive the next generation of treatments.
Enhancing Regulatory Compliance and Pharmacovigilance
For all the promise of data science, pharma is a heavily regulated industry – any use of AI or big data must ensure patient safety, data integrity, and compliance with stringent regulations. In 2025, we see data science being applied not only to scientific problems but also to regulatory and compliance challenges. From monitoring drug manufacturing processes to managing documentation and safety reporting, AI and analytics are helping companies stay compliant more efficiently, while regulators themselves are adapting their expectations to the new technological reality.
One notable trend is the rise of AI-powered compliance platforms. As operations generate ever more data (in manufacturing, quality control, clinical monitoring, etc.), maintaining compliance in real-time is daunting with manual methods. 2025 will see wide adoption of AI-based compliance monitoring solutions that can continuously track processes and data against regulatory requirements (How data science and AI will transform life sciences in 2025 - pharmaphorum). These platforms ingest streams of data – for example, from a manufacturing line's sensors or a clinical trial database – and automatically check for deviations or potential non-compliance. They can flag issues like out-of-specification results, protocol deviations, or missed reporting deadlines instantly for remediation. By providing real-time oversight of regulatory adherence across clinical trials, drug manufacturing, and other areas, such AI solutions help organizations maintain GxP (good practice) standards and operational integrity (How data science and AI will transform life sciences in 2025 - pharmaphorum). This is increasingly valuable as regulations grow more complex and data-intensive. In pharmacovigilance (drug safety monitoring), machine learning is being used to detect signals of adverse drug reactions from large datasets, including patient reports, EHRs, and even social media. Rather than relying on periodic manual review, AI algorithms can continuously scan for unusual patterns that might indicate a safety issue, enabling faster reporting to FDA and proactive risk management.
Another area of impact is regulatory documentation and submission preparation. We touched on NLP for authoring clinical study reports earlier – this directly feeds into regulatory workflows. Compiling a New Drug Application (NDA) or Biologics License Application (BLA) for FDA approval involves tens of thousands of pages of documentation. Large language models and automation tools are now assisting in preparing and QC'ing these submissions, ensuring that datasets, summaries, and reports are consistent and compliant with standards. By 2025, some companies are using AI to auto-generate first drafts of certain sections of regulatory filings (e.g., clinical summaries) based on the underlying trial data, which humans then refine. This speeds up the submission process and reduces transcription errors. Furthermore, intelligent search tools can quickly retrieve precedent language or relevant regulations for regulatory affairs teams, improving the quality of submissions.
Crucially, regulatory agencies themselves are evolving. The FDA and EMA have been actively engaging with AI/ML developments to update their guidance and expectations. In the U.S., the FDA in early 2025 issued its first draft guidance specifically on using AI in drug development and regulatory decision-making (FDA guidance on use of AI in drug development) (FDA guidance on use of AI in drug development). This guidance, titled "Considerations for the Use of Artificial Intelligence to Support Regulatory Decision-Making for Drug and Biological Products," lays out a risk-based framework for assessing the credibility of AI models when used in submissions (FDA guidance on use of AI in drug development). The FDA is essentially telling industry: if you want to use an AI model's output as evidence (say, to support approving a drug or selecting a dose), you need to demonstrate that the model is reliable, validated for its context of use, and appropriately risk-managed. FDA Commissioner Robert Califf highlighted that the agency seeks to promote innovation with AI while ensuring rigorous scientific and regulatory standards are upheld, likely through a risk-based oversight approach (FDA guidance on use of AI in drug development). Similarly, the European Medicines Agency finalized a reflection paper in late 2024 on AI in the medicinal product lifecycle, which mirrors many of the FDA's concerns and principles (FDA guidance on use of AI in drug development). Both regulators emphasize transparency, data quality, validation, and human oversight for AI algorithms.
As a result, life sciences companies must strengthen their data governance and validation practices for AI. FDA and EMA expect that any data used to train or run AI models is high quality (accurate, representative, and free of bias), and that companies can explain and justify their AI's decisions where they impact patient safety. Data integrity remains a watchword – agencies require that all data (whether used in an AI model or in traditional analysis) are reliable and accurate and that firms have strategies to mitigate data integrity risks (Using ALCOA to Ensure Data Integrity in the Age of AI - QAD Blog). Time-honored FDA principles like ALCOA (data should be Attributable, Legible, Contemporaneous, Original, Accurate) are as critical as ever in the digital age (Using ALCOA to Ensure Data Integrity in the Age of AI - QAD Blog). In fact, the ALCOA+ framework (expanded to include Complete, Consistent, Enduring, and Available) is being applied to modern data systems to ensure trustworthy datasets for AI and analytics (Using ALCOA to Ensure Data Integrity in the Age of AI - QAD Blog). Companies are training employees on data integrity practices, conducting regular audits, and using digital tools to enforce ALCOA compliance in data capture (Using ALCOA to Ensure Data Integrity in the Age of AI - QAD Blog). The goal is to prevent the classic "garbage in, garbage out" scenario – if an AI is fed flawed data, it will produce flawed results, which in pharma could have serious health consequences (Using ALCOA to Ensure Data Integrity in the Age of AI - QAD Blog). By shoring up data governance, firms both satisfy regulators and improve the performance of their data science initiatives.
It's worth noting that regulators aren't just issuing paper guidance; they are collaborating with industry and other stakeholders. The FDA has programs like the Emerging Technology Program and the Advanced Manufacturing Technologies initiative that engage companies to understand and oversee novel uses of AI in areas like drug manufacturing (Using ALCOA to Ensure Data Integrity in the Age of AI - QAD Blog). FDA centers (CDER, CBER, CDRH) along with the Digital Health Center of Excellence have been holding workshops and published discussion papers on AI/ML in drug development (Using ALCOA to Ensure Data Integrity in the Age of AI - QAD Blog). They are seeking a patient-centered approach to AI regulation, even soliciting input on issues like AI transparency, cybersecurity for AI in medical devices, and quality assurance (Using ALCOA to Ensure Data Integrity in the Age of AI - QAD Blog). The EMA, for its part, released an AI workplan and principles to guide AI usage in medicines regulation (Artificial intelligence - European Medicines Agency (EMA)), and is working on guidance that will likely become official policy in the coming years. All these developments signal that regulatory compliance in 2025 and beyond will explicitly include AI and data considerations. Pharma IT and data teams must therefore work hand-in-hand with regulatory affairs to ensure any advanced analytics are validated and documented in line with evolving FDA/EMA expectations.
Lastly, pharmacovigilance and post-market surveillance benefit from data science. With drugs on the market, monitoring their safety in large populations is crucial. AI algorithms now assist in combing through post-market data – including spontaneous adverse event reports, electronic health record data, and insurance claims – to detect rare side effects or efficacy issues faster. The FDA's Sentinel initiative, for example, uses big data from insurance databases to assess drug safety signals. In 2025, we can foresee NLP being used to process patient feedback or physician notes to catch early warning signs that might not be captured in structured data. Automation of compliance reporting is also more common; companies use analytics to automatically compile periodic safety update reports (PSURs) or other regulatory reports, ensuring nothing is missed. Even manufacturing compliance (e.g., ensuring ongoing Good Manufacturing Practice) is aided by data analytics that can detect subtle shifts in process control data that a human might overlook.
In summary, data science is not just accelerating R&D, it's also being applied to keep pharma compliant and patients safe, all while regulators update their frameworks in tandem. 2025 stands at a turning point where AI's potential is being embraced, but with careful attention to ethics, data privacy, and robust validation (How data science and AI will transform life sciences in 2025 - pharmaphorum). Those companies that manage to innovate responsibly will lead the industry forward.
Workforce and Talent Trends in Data-Driven Life Sciences
The surge of data science in pharma has profound implications for the workforce. In the United States, pharmaceutical companies are in a fierce race for talent who can bridge life sciences and data analytics. As the industry's focus shifts to digital innovation, a variety of data-centric roles are in high demand, including data scientists, data engineers, bioinformaticians, and computational biologists (Essential Data Science Roles in Life Sciences for 2024 - CSG Talent) (Essential Data Science Roles in Life Sciences for 2024 - CSG Talent). Life sciences organizations are building entire data science teams and even new departments to support these initiatives. A 2024 analysis noted that "data-driven roles are prevalent in life science due to the complex nature of biological systems and the importance of analyzing large amounts of data to advance personalized medicine, drug discovery, and regulatory compliance." (Essential Data Science Roles in Life Sciences for 2024 - CSG Talent) This trend has only intensified into 2025.
Key roles being hired or scaled up include:
- Data Scientists – experts in statistical modeling and machine learning who can uncover insights from complex datasets. In pharma, they tackle problems from optimizing clinical trial enrollment to predictive modeling of drug outcomes. Their work has a significant impact on improving products and processes, as they identify trends that might, for example, improve a formulation or pinpoint a subgroup of patients who benefit most from a therapy (Essential Data Science Roles in Life Sciences for 2024 - CSG Talent) (Essential Data Science Roles in Life Sciences for 2024 - CSG Talent).
- Data Engineers – professionals who build the data pipelines and infrastructure to gather, clean, and organize data for analysis. They ensure data from labs, trials, EHRs, etc., flows into analytics platforms in a usable, secure form (Essential Data Science Roles in Life Sciences for 2024 - CSG Talent). With pharma's huge data growth (clinical, genetic, sensor, imaging data, etc.), engineers are critical to manage and curate these troves (Essential Data Science Roles in Life Sciences for 2024 - CSG Talent).
- Bioinformaticians and Computational Biologists – specialists at the intersection of biology and computing. They apply algorithms to biological data, such as sequencing data, often working on genomics, proteomics, or systems biology problems (Essential Data Science Roles in Life Sciences for 2024 - CSG Talent). These roles are key for personalized medicine initiatives, where understanding genetic data is essential (Essential Data Science Roles in Life Sciences for 2024 - CSG Talent). Teams of bioinformatics scientists develop novel algorithms to interpret genomic data and discover new drug targets or biomarkers (Essential Data Science Roles in Life Sciences for 2024 - CSG Talent).
- Data Architects – responsible for the overall strategy of how data is stored, integrated, and governed in the organization (Essential Data Science Roles in Life Sciences for 2024 - CSG Talent). They design architectures that comply with regulations (ensuring data is secure and access-controlled) while enabling the analytical teams to easily find and use data.
- AI/ML Engineers – who develop and deploy AI models into production (for example, integrating an AI-driven decision support tool into a clinical workflow).
- Analytics Translators or Healthcare Data Analysts – roles that often serve to bridge domain experts (biologists, physicians) and data teams, ensuring the right questions are being asked and the results of analysis are interpretable in a medical context.
Moreover, companies are realizing they need leadership roles to champion data science. It's increasingly common to see titles like Chief Data Officer (CDO) or VP of Data Science at pharma companies, indicating a top-level commitment to data strategy (Essential Data Science Roles in Life Sciences for 2024 - CSG Talent). Indeed, many organizations have elevated technology leadership: over half of large life sciences companies have C-suite or executive roles (Chief Digital or Information Officers, etc.) driving these efforts (2025 AI Trends: Life Sciences Leaders on Data, Digital and AI - ZS) (2025 AI Trends: Life Sciences Leaders on Data, Digital and AI - ZS). These leaders focus on aligning data projects with business goals, measuring ROI, and fostering a data-driven culture. In 2025, survey data shows companies seek "boundary-spanning leaders" who can bridge tech and business and drive capability development (2025 AI Trends: Life Sciences Leaders on Data, Digital and AI - ZS).
A critical workforce trend is the emphasis on upskilling and digital fluency across the board. As advanced as data science tools are, they won't deliver value if the broader workforce can't use them or trust them. Many pharma companies are investing in training programs to raise the data literacy of their scientists, clinicians, and managers. According to a ZS survey, 69% of life science firms plan to invest in AI and digital upskilling for their employees in 2025 (up from 51% the year prior) (2025 AI Trends: Life Sciences Leaders on Data, Digital and AI - ZS). This includes training bench scientists in basic coding or statistics, educating clinicians on how to interpret AI outputs, and teaching all staff about data privacy and security practices. Alongside upskilling, attracting new digital talent is a priority – 56% of companies plan to change how they recruit tech talent (e.g. offering more flexible work arrangements, partnering with universities, or highlighting meaningful healthcare missions to lure data experts) (2025 AI Trends: Life Sciences Leaders on Data, Digital and AI - ZS).
Notably, the life sciences sector's job growth has been robust. Even in 2024, U.S. life sciences employment grew around 7%, outpacing many sectors, fueled partly by the demand for roles in biotech and data analytics (Year-End Wrap-Up: The State of Life Sciences Employment ClinLab Staffing). Specifically, data scientists in healthcare have become essential as organizations leverage big data to improve outcomes (Year-End Wrap-Up: The State of Life Sciences Employment ClinLab Staffing). The need for these roles is so acute that companies are sometimes competing with tech giants for the same talent pool. This has led pharma firms to increase collaboration with academia (sponsoring data science programs, offering internships and fellowships) and to leverage remote work to access talent beyond their geographic area. As one staffing report noted, ~68% of U.S. life science employers now offer some remote or flexible work options, partly to attract top professionals from across the country (Year-End Wrap-Up: The State of Life Sciences Employment ClinLab Staffing) – an important consideration for data science roles that may not need to be on-site in a lab every day.
The evolving nature of work is also prompting cultural shifts. Effective data science in pharma often requires interdisciplinary collaboration: bioinformaticians must work closely with biologists; data engineers must coordinate with clinical data managers; AI specialists need input from therapeutic area experts. Companies are fostering cross-functional teams, sometimes organized around specific goals (e.g., an "AI for Oncology" task force including oncologists, data scientists, and software developers). There is a push for teams oriented around outcomes rather than silos – for example, creating integrated teams to improve trial efficiency rather than each department doing its piece in isolation (2025 AI Trends: Life Sciences Leaders on Data, Digital and AI - ZS). This aligns with the finding that 72% of organizations plan to create teams focused on company-wide goals (rather than individual tech projects) to maximize impact (2025 AI Trends: Life Sciences Leaders on Data, Digital and AI - ZS).
In addition, soft skills are recognized as vital. Communication and the ability to translate between technical and clinical domains is highly valued (Essential Data Science Roles in Life Sciences for 2024 - CSG Talent). Data professionals in pharma must often explain complex models or analyses to decision-makers who aren't data experts, making clear communication a sought-after skill. Problem-solving and critical thinking are emphasized as well – data teams face novel problems (like cleaning a messy real-world dataset or figuring out how to validate an AI prediction in a clinical context) that require creativity and rigorous thinking (Essential Data Science Roles in Life Sciences for 2024 - CSG Talent).
To summarize, the life sciences workforce in 2025 is being reshaped to support a data-rich, AI-enabled industry. Companies are hiring aggressively for data talent and also reskilling existing employees. The competition for skilled data scientists, engineers, and bioinformaticians is intense, and organizations are adopting new strategies to build and retain these teams. Those that succeed will have a formidable edge, as they'll be able to fully leverage technology to drive innovation. As a senior recruiter put it, with the widespread adoption of AI and data in life sciences, "having a strong network of data-driven professionals has never been more important" (Essential Data Science Roles in Life Sciences for 2024 - CSG Talent). The human element – talent and culture – is ultimately the linchpin that will determine how well pharma realizes the potential of its data science investments.
Data Governance, Interoperability, and Security Challenges
With great volumes of data and advanced analytics come great challenges in data governance, interoperability, and security. The pharmaceutical industry in 2025 is grappling with how to effectively manage and share data while maintaining high standards of quality, privacy, and compliance. IT professionals in pharma must navigate these challenges to enable the promise of data science.
Data governance refers to the policies, processes, and standards that ensure data is managed properly across the enterprise. In a domain like pharma, data governance is absolutely critical: errors or mismanaged data can have regulatory ramifications and patient safety implications. Key aspects of governance include data quality, integrity, privacy, and lifecycle management. Companies are implementing comprehensive data governance frameworks to handle the flood of data from R&D and commercial activities, recognizing that this is not a "nice-to-have" but an absolute necessity.
One major challenge is ensuring data quality and integrity at scale. As data is collected from multiple sources (lab instruments, clinical forms, real-world databases, etc.), maintaining accuracy and consistency is difficult. Pharma companies adhere to FDA's data integrity guidelines – as mentioned, the ALCOA+ principles – to make sure data is trustworthy. This involves establishing controls such as audit trails on databases (so any change to data is recorded), standardized procedures for data entry and curation, and periodic audits for compliance (Using ALCOA to Ensure Data Integrity in the Age of AI - QAD Blog). Automation can help: companies use digital tools for data capture that enforce format and completeness rules, reducing manual errors (Using ALCOA to Ensure Data Integrity in the Age of AI - QAD Blog). Master data management systems are also used to keep reference data consistent (for example, ensuring that a clinical trial site or an investigator's name is recorded uniformly across systems). Despite these efforts, data silos and inconsistency remain pain points – many pharma organizations historically have had separate systems for research, clinical, manufacturing, etc., each with their own data conventions. Breaking down these silos to create a unified, high-quality data repository is a work in progress. It's encouraging that 77% of companies say they have adjusted or plan to overhaul their data strategies (governing how data is collected, managed and used) in light of AI opportunities (2025 AI Trends: Life Sciences Leaders on Data, Digital and AI - ZS).
Interoperability is closely related. It's the ability of different IT systems and datasets to connect and exchange information in a usable way. Interoperability is crucial for life sciences because insights often emerge from linking data across domains – for example, connecting clinical trial data with real-world patient outcomes, or combining genomic data with electronic health record data. However, technical and semantic incompatibilities can make such integration challenging. To improve interoperability, the industry and regulators have been pushing data standards: for instance, the FDA mandates the use of CDISC standardized data formats (SDTM, ADaM) for clinical trial submissions, ensuring that submitted trial data is in a consistent structure. In the healthcare domain, standards like HL7 FHIR are enabling the exchange of electronic health records in a structured format, which is very useful when pharma wants to ingest EHR data for research or pharmacovigilance. By 2025, we also see efforts to standardize genomic data formats and use common ontologies for biomedical concepts, so that datasets from different studies can be more easily aggregated.
Another aspect is building the IT infrastructure that supports interoperability. Many companies are investing in enterprise data platforms or data lakes that consolidate data from various sources. For example, a pharma might create a cloud-based data lake where preclinical data, clinical trial databases, manufacturing batch records, and commercial analytics data all reside (with appropriate access controls). On top of this, they implement an API layer or integration middleware that allows different applications to query and update this data. Modern data catalogs are deployed so that data scientists can discover what data exists and understand its provenance and definitions. The technical heavy lifting here is substantial, but the payoff is that once data is integrated, AI and analytics can draw from a rich, interconnected data foundation.
One real-world driver of interoperability in the U.S. has been regulatory compliance in the supply chain: the Drug Supply Chain Security Act (DSCSA), for instance, requires an interoperable system to trace prescription drugs through the supply chain by 2023-2025 (Top 4 trends shaping life sciences operations in 2025 - European Pharmaceutical Manufacturer). This has pushed companies to adopt standards for exchanging data with partners and regulators (like EPCIS for serialization data). While supply chain track-and-trace is tangential to R&D, it underscores a broader theme – cross-industry and cross-company data sharing is increasingly important in life sciences. Partnerships between pharma companies, contract research organizations (CROs), academic centers, and tech companies mean data is moving across organizational boundaries. Ensuring interoperability (technically and legally) in these collaborations is a challenge that requires agreed data standards, secure exchange mechanisms, and clear data ownership/use policies.
Privacy and security are paramount concerns overlaying all data activities. Pharma deals with highly sensitive data: patient health information (which is protected under HIPAA and other privacy laws), confidential R&D data (which is intellectual property), and personal data of trial participants (subject to regulations like GDPR if in Europe). As the volume of data grows and it's used in more ways (e.g., AI modeling), protecting this data from breaches or misuse is a top priority.
Cybersecurity, in particular, has become a board-level issue. In 2025, cyber attacks on pharmaceutical companies are expected to make headlines, especially multi-company breaches that exploit the interconnected nature of modern systems (Top 4 trends shaping life sciences operations in 2025 - European Pharmaceutical Manufacturer). With remote monitoring, IoT devices, and cloud systems expanding the attack surface, vulnerabilities have increased. A recent example was a cyberattack on Cencora (AmerisourceBergen) in 2024 that disrupted operations at multiple pharma companies down the supply chain (Top 4 trends shaping life sciences operations in 2025 - European Pharmaceutical Manufacturer). In response, regulators and industry groups are tightening cybersecurity requirements. The FDA has issued guidance on cybersecurity for medical devices and has an eye on manufacturing system security as well. In the EU, the NIS2 directive now subjects pharmaceutical manufacturers to strict cybersecurity mandates (Top 4 trends shaping life sciences operations in 2025 - European Pharmaceutical Manufacturer). Pharma companies are bolstering their defenses: conducting thorough risk assessments, patching legacy systems, segmenting networks, and improving incident response plans. However, gaps remain – a European analysis found about 10% of pharma/health companies lacked a proper vulnerability management plan as of 2024 (Top 4 trends shaping life sciences operations in 2025 - European Pharmaceutical Manufacturer). Bridging these gaps by 2025 is critical, as any significant data breach could not only compromise patient data but also shake public trust in how companies handle sensitive health information.
On the privacy front, compliance with data protection regulations is non-negotiable. In the U.S., while there's no unified federal privacy law for all health data beyond HIPAA, companies must adhere to various state laws (like CCPA in California) and ensure any patient data from clinical trials is used only with consent and for approved purposes. For global trials, GDPR in Europe imposes strict requirements on processing personal data, including genetic data (which is considered highly sensitive). Data governance teams work to pseudonymize or anonymize data where possible for secondary use, and legal teams craft data sharing agreements that define how partners can use shared data. We also see "privacy by design" principles being incorporated into data platforms – e.g., role-based access controls to ensure only authorized personnel can see identifiable data, and audit logs to track data access.
Another facet of governance is ethical AI and algorithm accountability. As AI models make inroads in decision-making, companies are forming internal AI ethics committees or guidelines to oversee fair and appropriate use of AI. This might cover ensuring that AI models do not inadvertently discriminate against certain patient groups, especially in areas like patient recruitment or treatment recommendations. It also covers being transparent (where appropriate) that AI is being used – for example, informing trial participants if an AI was used to screen their eligibility, or doctors if an AI is assisting in diagnostic decisions.
Finally, there's the challenge of change management – implementing governance and interoperability solutions requires cultural and procedural change. Scientists and analysts may need to adapt to using centralized systems or following new standards, which can meet resistance if not handled well. Strong executive support and clear communication about the value of these governance measures (e.g., "by standardizing our data, we can get results X times faster" or "by following these privacy steps, we protect our patients and our reputation") are necessary to get buy-in across the organization.
In essence, data governance and interoperability efforts are the unsung heroes enabling data science success. They may not be as glamorous as AI algorithms, but without well-governed, high-quality data that flows where it's needed, even the best algorithms will flounder. The U.S. pharma industry in 2025 is investing heavily in these backbone areas – building the data foundations, establishing trust and security, and aligning with regulatory expectations – to ensure that the vast potential of data science can be realized responsibly and effectively.
Evolving Regulatory Expectations (FDA/EMA) in the Data Science Era
As we've weaved throughout the discussion, regulatory bodies like the U.S. Food and Drug Administration (FDA) and the European Medicines Agency (EMA) are actively modernizing their frameworks in response to data science advancements. IT professionals in pharma must stay attuned to these evolving regulatory expectations, as they shape what is required to successfully develop and gain approval for new therapies in this data-rich era.
A headline development is the FDA's focus on AI/ML in drug development. In January 2025, the FDA released a landmark draft guidance on the use of AI for drug and biologic development – the agency's first formal guidance in this area (FDA guidance on use of AI in drug development). This guidance doesn't give hard-and-fast rules (it's still draft), but it provides insight into the FDA's thinking. The FDA advocates a risk-based approach to AI, meaning the level of scrutiny on an AI tool should correspond to the impact that tool's output has on decision-making (FDA guidance on use of AI in drug development). If a machine learning model is being used to decide critical trial aspects (like determining the dose for first-in-human trials, or identifying which patients to enroll), the FDA will expect rigorous validation and evidence of the model's credibility. The guidance introduces concepts like "context of use" for AI models and a credibility assessment framework (FDA guidance on use of AI in drug development). In practice, sponsors will need to provide documentation to FDA on how an AI model was developed (what data, what algorithm), its performance (accuracy, error rates, generalizability), and what controls are in place to ensure it doesn't output something misleading. Essentially, if companies want to submit AI-derived insights as part of an approval package, they must establish the reliability of those insights to the same degree as any lab assay or clinical measurement.
The EMA has paralleled these moves. In late 2024, EMA published a Reflection Paper on AI in the medicinal product lifecycle, covering AI use from drug discovery to clinical trials and post-market (EMA adopts reflection paper on the use of Artificial Intelligence (AI)). While not legally binding, it reflects EMA's views and will inform future guidelines. EMA's principles stress patient safety, data transparency, and the importance of human oversight of AI. For instance, EMA suggests that companies using AI in clinical trials should pre-specify that in the trial protocol and discuss how it might affect trial conduct or data integrity. The EMA is also watching developments around the broader EU AI Act – a proposed regulation classifying AI systems by risk. Certain AI uses in pharma (like those influencing treatment decisions) might be deemed "high-risk" and subject to requirements such as conformity assessments or post-market monitoring under this law (EMA's AI Workplan: Integrating Artificial Intelligence into Healthcare). By 2025, while the AI Act isn't finalized, pharma companies operating in Europe are already considering its potential impact in their AI deployment strategies (AI in Pharma: Key Regulatory Developments - LinkedIn).
Both FDA and EMA place huge emphasis on data quality and integrity in the context of AI. As noted earlier, regulators expect that any data feeding AI models meets the same high standards as data in a clinical trial submission. Regulatory guidelines on Computerized Systems and Data Integrity (FDA's 21 CFR Part 11, EMA's Annex 11, etc.) apply to AI systems too – for example, ensuring an AI software that processes clinical data is validated and has audit trails. Regulators also encourage sponsors to engage in dialogue when using novel data approaches. The FDA, for example, has an Emerging Technology Team (ETT) for drug manufacturing that companies can consult if they want to use an innovative AI-based process so that regulatory considerations are addressed early (Using ALCOA to Ensure Data Integrity in the Age of AI - QAD Blog). For clinical development, FDA has been hosting public meetings and issuing discussion papers to get feedback on how to regulate AI without stifling innovation (Using ALCOA to Ensure Data Integrity in the Age of AI - QAD Blog). Collaboration and transparency are the themes – FDA is working with industry, patients, and international counterparts to figure out frameworks that assure safety and efficacy in the age of AI (Using ALCOA to Ensure Data Integrity in the Age of AI - QAD Blog).
Regulatory expectations are also evolving in terms of data submissions. The FDA and EMA are modernizing how data is submitted and reviewed. FDA's Center for Drug Evaluation and Research (CDER) has been expanding its capability to review real-world evidence in submissions, as enabled by the 21st Century Cures Act and PDUFA VI commitments. We've seen the FDA approve new indications for drugs partly based on RWE analyses (for instance, using real-world data as supportive evidence of a drug's effectiveness in a subpopulation). By 2025, sponsors know that if they plan to use RWE or novel data sources to support a label claim, they should discuss it with the FDA early and ensure their methodologies are sound. The agencies have also released guidance on topics like the use of digital health technologies in clinical trials (e.g., wearables data), decentralized trial conduct, and using EHR and claims data for regulatory decisions. All these point to regulators encouraging innovation in trial design and evidence generation, but reminding sponsors that methodological rigor is key. For instance, an FDA guidance on RWE (December 2021) outlines how to design observational studies that could be submitted for approval, emphasizing things like data provenance and bias reduction – principles that data scientists must incorporate when analyzing real-world datasets for regulatory use.
Another area is regulatory operations efficiency: agencies themselves are leveraging data science to enhance their review processes. The FDA has been investing in technology to handle the growing volume of data in submissions – including possibly using AI to sort through thousands of pages of applications to find key information or to check datasets for anomalies. The EMA's workplan on AI includes improving personal productivity of regulators with AI tools (Artificial intelligence - European Medicines Agency (EMA)). This means that down the line, sponsors might interact with AI-assisted regulators – for example, an AI might flag to a reviewer that a submitted trial dataset has discrepancies versus the protocol, or suggest questions to ask the sponsor. While this is mostly internal to agencies, it reflects that regulators are not just passively overseeing industry's use of AI; they are adopting data science themselves. This may lead to changes in what they ask from companies. For instance, if the FDA uses an AI to re-analyze a clinical dataset and finds a different result, they will certainly query the sponsor. As such, alignment on analytical methods could become part of the sponsor-regulator dialogue.
In the realm of manufacturing and quality, the FDA's Center for Drug Evaluation and Research and Center for Biologics (CBER) have been looking at AI in biomanufacturing (for process monitoring, predictive maintenance, etc.). They've indicated that they plan to integrate considerations for AI into their existing frameworks for process validation and quality systems. We may see guidance on AI in Good Manufacturing Practice (GMP) down the road. The key expectation is that if companies use AI to control or monitor manufacturing, they must ensure the AI is validated and doesn't compromise product quality – essentially treating it like any other piece of production equipment that needs qualification.
One more noteworthy regulatory trend is around data transparency and sharing. Regulatory bodies are increasingly pushing for the sharing of clinical trial data (anonymized) for scientific and public health purposes. FDA has programs for observational RWD submissions where they require the datasets and code be made available for audit. EMA has its clinical data publication policy (currently paused due to resource issues, but philosophically in favor of transparency). What this means for data science is more external scrutiny and collaboration: companies might have to provide data to regulators in analysis-ready formats and perhaps even share AI algorithms or validation study results. Being prepared to explain one's data science methodology in a transparent way is likely to become part of regulatory submissions. For example, if a model was used to adjudicate ambiguous endpoints in a trial, the sponsor might need to share the model or at least its performance characteristics.
In conclusion, FDA and EMA are embracing the data science revolution, but with careful guardrails. The expectations in 2025 are that pharma companies using AI and advanced analytics must do so with robust scientific discipline and documentation. The agencies are keen to see innovation that can benefit patients – FDA's Robert Califf himself said AI has "transformative potential to advance clinical research and accelerate product development to improve patient care, with appropriate safeguards in place." (FDA guidance on use of AI in drug development). So the onus is on industry to implement those safeguards: ensure high-quality data, validate models, maintain transparency, and keep humans in the loop for critical decisions. Regulators will likely reward companies that do this by showing flexibility and openness to novel approaches. Those that cut corners, however, could face setbacks (e.g., FDA refusing to consider an analysis because the model was a "black box" with unexplained behavior). For IT and data professionals in pharma, staying ahead means continuously monitoring guidance updates, participating in industry forums about standards (like TransCelerate or PHUSE working groups on data science), and baking compliance into the design of data systems from the start. The regulatory landscape is evolving in tandem with technology – and in 2025, it's clear that successful pharma data science strategies will be those aligned with this evolving landscape.
Leading Companies and Case Studies
The push for data science in life sciences is evident across big pharma, biotech startups, and tech companies entering the healthcare space. It's worth highlighting a few key players and initiatives that exemplify the trends discussed:
-
Pfizer, Merck, Johnson & Johnson, and other Big Pharmas: Large U.S. pharma companies have heavily invested in AI and analytics programs. For example, Pfizer has used machine learning models to analyze immuno-oncology data and famously partnered with IBM Watson in the past for immune-oncology research (an effort that laid groundwork for later AI projects). Pfizer also leveraged real-world data during the COVID-19 vaccine rollout to monitor safety and effectiveness at scale, demonstrating data agility. Merck has a longstanding biostatistics and ML group; it has collaborated with AI firms (like collaborating with Accenture on a cloud data platform, or investing in startup projects for drug discovery). J&J's data science teams have worked on everything from surgical robotics AI (through their Ethicon division) to using AI in clinical trial recruitment and analysis.
-
Novartis and Microsoft Alliance: Although Novartis is Swiss-based, its collaboration with Microsoft has global impact, including in the U.S. The Novartis AI Innovation Lab, founded in 2019 with Microsoft as a strategic partner, is a multi-year effort to infuse AI across Novartis' R&D. They've worked on projects such as using Azure AI services to analyze imaging data for ophthalmology (personalized therapies for macular degeneration) (Novartis, Microsoft Announce Artificial Intelligence Collaboration), and to generate molecules for complex targets using generative models. By 2025, Novartis reports that this alliance has accelerated some discovery programs and improved scientists' productivity by providing self-service AI tools for routine tasks. This is a case of a pharma partnering with a tech giant to get access to cloud and AI expertise at scale.
-
Roche/Genentech and Real-World Data: Roche, through its Genentech arm in the U.S., has been a leader in using real-world evidence. Roche's 2018 acquisition of Flatiron Health (an oncology EHR data company) was a bold move to integrate real-world patient data into its development and regulatory process. By 2025, Roche uses real-world oncology data to support label extensions and to design smarter post-market studies. A notable example was FDA's approval of Roche's drug Alecensa in a new lung cancer indication partly supported by Flatiron-derived RWE. Roche also has a Personalized Healthcare division that employs data scientists to build algorithms predicting disease progression in multiple sclerosis and other diseases using combined clinical and imaging data.
-
Sanofi's Digital Accelerator: Sanofi (France-based, but with a big U.S. presence) launched a "Digital Accelerator" in 2022 to fast-track data projects. We saw earlier how Sanofi uses digital twins and AI to shrink R&D timelines (2025 life sciences outlook - Deloitte Insights). They have also partnered with AI startups (like Exscientia for oncology drug discovery, and Insilico Medicine for new target discovery). Sanofi's example shows how a traditional pharma is reorienting its research approach around in silico methods, with tangible time savings.
-
AstraZeneca and AI Partnerships: AstraZeneca (UK/Sweden-based, large U.S. operations in oncology) has multiple AI collaborations. They partnered with BenevolentAI, a notable AI biotech, to identify new drug targets using knowledge graphs and machine learning. This partnership reportedly yielded a novel target for idiopathic pulmonary fibrosis which AstraZeneca moved into internal development. AstraZeneca also works with Schrödinger (a computational chemistry company) on physics-based and machine learning methods to design drugs for challenging targets. By 2025, AstraZeneca has integrated AI as a core component of its research, evidenced by a number of publications and conference presentations showing AI-assisted discoveries.
-
AI-Driven Biotechs: A wave of startups specialized in AI for life sciences emerged in the last 5-10 years, and by 2025 some have reached maturity:
- Insilico Medicine (as discussed) – with an AI-discovered drug in clinical trials, it's a poster child for AI in drug discovery.
- Recursion Pharmaceuticals – a U.S. biotech that built an automated high-content screening platform using AI to analyze cellular images. Recursion has amassed one of the largest biological image datasets and uses it to identify drug repurposing opportunities. They attracted partnerships with Bayer and others, and by 2025 have multiple compounds in trials found via their platform.
- Exscientia – a UK AI drug design company, but active in the U.S. market (Nasdaq-listed). They delivered AI-designed molecules to partners like Sumitomo Dainippon and Sanofi, some reaching clinical stages. Exscientia also implemented AI-driven patient selection in oncology trials (via AI-guided biomarkers).
- Tempus – a Chicago-based company focusing on AI and precision medicine, which has built a huge clinicogenomic database (especially in oncology) and provides AI-driven insights for treatment decisions. Tempus collaborates with both academic centers and pharma on trials where their analytics identify which patients might benefit from experimental therapies.
- IBM Watson Health (now Merative) – While IBM's grand vision for Watson in healthcare didn't fully materialize as initially hyped, the spin-off Merative still offers AI analytics for clinical development and real-world data management. Some pharma companies use these tools for trial design optimization or real-world study analysis.
- NVIDIA and BioNTech – an interesting partnership is between AI hardware giant NVIDIA and BioNTech (of mRNA vaccine fame) announced in 2022 to build a "BioNTainer," essentially an AI-powered platform in a container for rapid mRNA vaccine production design. This kind of cross-industry collaboration shows how AI and advanced computing are crossing into novel biotech manufacturing.
-
Case Study – AI in Action for Drug Repurposing: During the early COVID-19 pandemic, data science proved its worth when researchers used AI algorithms on large compound databases to suggest existing drugs that might work against the virus. For instance, BenevolentAI's platform identified the rheumatoid arthritis drug baricitinib as a potential COVID-19 treatment by analyzing connections in the scientific literature, which subsequently led to clinical trials and an eventual emergency use authorization. This highlighted how AI can rapidly generate hypotheses by reading and linking disparate biomedical data – essentially doing in seconds what would take human experts months to piece together from thousands of papers.
-
Case Study – Clinical Trial Efficiency: Bristol Myers Squibb (BMS) implemented an analytics program to improve trial operations. By leveraging predictive models on historical trial data, they developed an AI that forecasts enrollment rates at each trial site and identifies when a site is lagging. Using this, BMS can proactively allocate resources or open new sites to stay on enrollment targets. They reported significantly reduced enrollment variance and avoided delays, saving both time and cost. Many big pharmas have similar "trial analytics" dashboards powered by data science.
-
Regulatory Innovation – FDA's Project Frontiers: The FDA itself has been running programs like Project Frontiers to use advanced analytics for pharmacovigilance. One notable success was using natural language processing on FDA's own adverse event databases to more quickly detect safety signals (like detecting patterns of a certain side effect across multiple drugs). The FDA's Center for Biologics (CBER) also uses machine learning to help review gene therapy trials – e.g., screening the submissions for any inconsistencies or scanning the literature to verify sponsor claims. Collaboration between FDA and industry on data (like the IMI's EHDEN project in EU or Sentinel in US) means regulators and companies often analyze the same datasets, ensuring consistency in interpretation.
These examples illustrate a broader point: the companies that embrace data science and form the right partnerships are making notable strides. It's a synergistic ecosystem – big pharmas bring domain expertise and data, tech firms bring cutting-edge AI tools, and startups bring agility and innovation. In the U.S., there's also significant government and academic collaboration. The NIH has invested in programs like Bridge2AI to drive AI in biomedical research, and the NSF funds advanced computing for health projects.
For IT professionals, studying these case studies shows what success looks like: strong executive sponsorship of data initiatives (e.g., Novartis' CEO backing the AI Lab), integrating new tech with legacy processes (e.g., trial analytics at BMS blending with traditional operations), and maintaining a patient-centered focus (e.g., Tempus using AI to match patients to best therapies). The U.S. context also offers unique opportunities such as large, diverse datasets (from big healthcare systems or claims databases) and a vibrant startup scene to collaborate with.
In conclusion, data science in the life sciences industry as of 2025 stands at an exciting and pivotal point. The current trends – from AI-driven R&D to real-world evidence generation – are converging to make drug discovery faster, clinical trials smarter, and treatments more personalized than ever before. Emerging technologies like machine learning, NLP, and cloud computing are no longer experimental side projects; they are central to strategy, with companies investing heavily across all business domains to scale these tools (2025 AI Trends: Life Sciences Leaders on Data, Digital and AI - ZS). We see their real-world impact in initiatives improving every stage of the pipeline: AI is designing molecules and predicting trial outcomes, big data analytics is unlocking insights from genomes and patient records, and automation is streamlining compliance and documentation tasks.
This progress comes with new challenges. Organizations must cultivate the right workforce, blending data expertise with life science know-how, and foster a culture of continuous learning and collaboration. They must implement robust data governance practices to ensure that quality, privacy, and security keep pace with the data deluge. And they must heed evolving regulatory guidance, building systems that not only comply with today's rules but are agile enough to adapt to tomorrow's standards around AI and data usage. The FDA and EMA are effectively raising the bar: to use advanced analytics in pharma, one must demonstrate responsibility, transparency, and reliability.
Overall, the U.S. pharmaceutical landscape in 2025 is one of dynamic transformation, driven by necessity and enabled by technology. Companies that successfully integrate data science into their DNA – and do so responsibly – are likely to lead in innovation, efficiency, and ultimately in bringing better therapies to patients. Those that lag may find themselves disrupted or outpaced. For IT and data professionals in pharma, it's a time of great opportunity: their skills are more valued than ever, and they sit at the forefront of scientific and operational breakthroughs. The industry mantra could well be "in data we trust" – with data science techniques proving instrumental in enhancing lives and advancing healthcare on a global scale (How data science and AI will transform life sciences in 2025 - pharmaphorum). The journey is ongoing, but 2025 is undeniably a milestone year where the vision of a data-driven life sciences sector is truly coming into fruition.
Sources: The information in this article is derived from a range of 2024–2025 industry reports, expert analyses, and case studies, including Deloitte's 2025 life sciences outlook (2025 life sciences outlook - Deloitte Insights) (2025 life sciences outlook - Deloitte Insights), pharmaphorum's predictions for AI in 2025 (How data science and AI will transform life sciences in 2025 - pharmaphorum) (How data science and AI will transform life sciences in 2025 - pharmaphorum), ZS Associates' life sciences tech survey (2025 AI Trends: Life Sciences Leaders on Data, Digital and AI - ZS) (2025 AI Trends: Life Sciences Leaders on Data, Digital and AI - ZS), and recent FDA/EMA regulatory publications (FDA guidance on use of AI in drug development) (Using ALCOA to Ensure Data Integrity in the Age of AI - QAD Blog), among other sources. These illustrate the current state and trajectory of data science in the pharmaceutical and biotech arena as we head through 2025 and beyond.
DISCLAIMER
The information contained in this document is provided for educational and informational purposes only. We make no representations or warranties of any kind, express or implied, about the completeness, accuracy, reliability, suitability, or availability of the information contained herein. Any reliance you place on such information is strictly at your own risk. In no event will IntuitionLabs.ai or its representatives be liable for any loss or damage including without limitation, indirect or consequential loss or damage, or any loss or damage whatsoever arising from the use of information presented in this document. This document may contain content generated with the assistance of artificial intelligence technologies. AI-generated content may contain errors, omissions, or inaccuracies. Readers are advised to independently verify any critical information before acting upon it. All product names, logos, brands, trademarks, and registered trademarks mentioned in this document are the property of their respective owners. All company, product, and service names used in this document are for identification purposes only. Use of these names, logos, trademarks, and brands does not imply endorsement by the respective trademark holders. IntuitionLabs.ai is an AI software development company specializing in helping life-science companies implement and leverage artificial intelligence solutions. Founded in 2023 by Adrien Laurent and based in San Jose, California. This document does not constitute professional or legal advice. For specific guidance related to your business needs, please consult with appropriate qualified professionals.