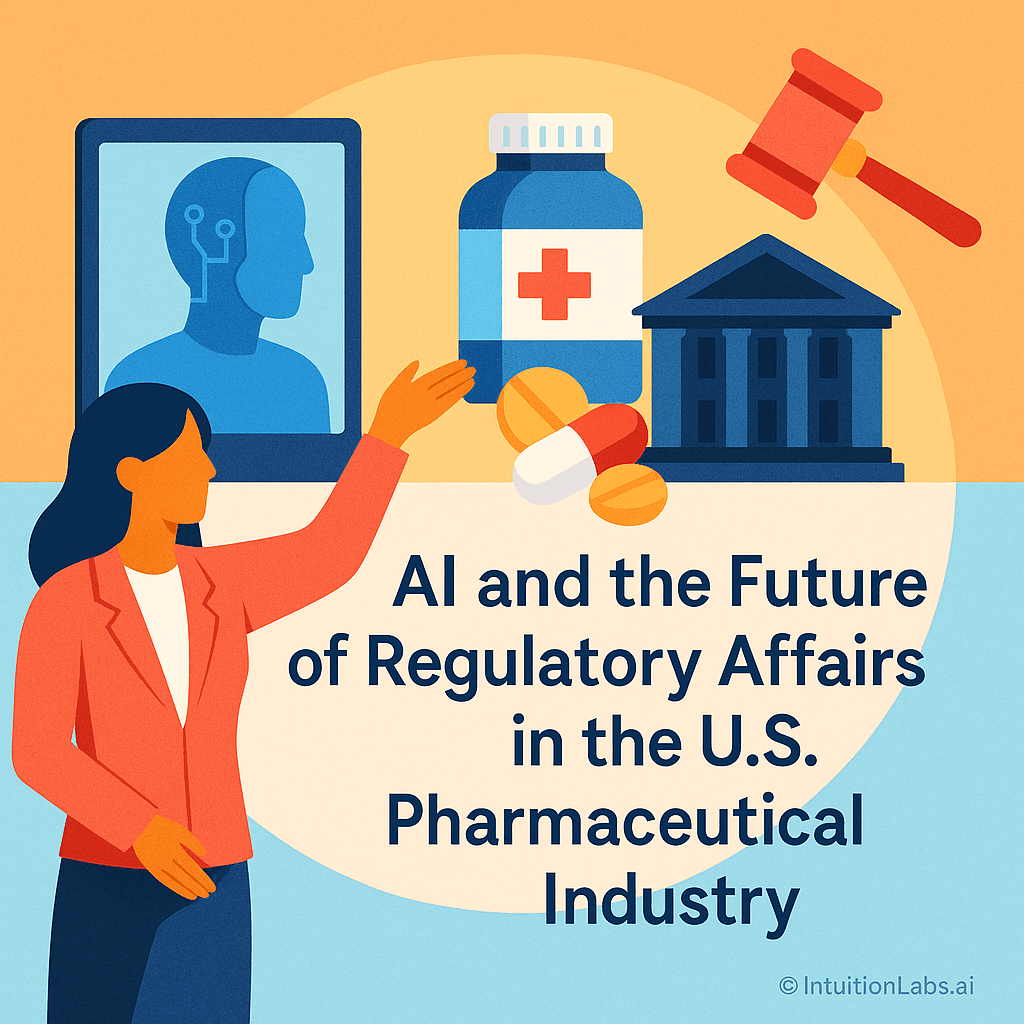
AI and the Future of Regulatory Affairs in the U.S. Pharmaceutical Industry
Introduction
Artificial Intelligence (AI) is poised to redefine regulatory affairs in the pharmaceutical sector. Regulatory affairs encompass the end-to-end process of drug approvals and oversight – from preparing submissions to post-market safety monitoring – all under stringent regulations. In recent years, pharma companies have widely adopted AI and machine learning (ML) tools to streamline these processes (Regulatory Concerns for AI: 2024 Trends) (Using AI to Boost Efficiency and Ensure Regulatory Compliance - Experic CDMO). This report explores current and emerging AI applications in regulatory submissions, compliance monitoring, labeling, pharmacovigilance, and quality assurance. It also analyzes how AI is transforming interactions with the U.S. Food and Drug Administration (FDA) and accelerating the drug approval pipeline. We review regulatory guidance on AI/ML in pharma, including FDA’s recent draft guidelines for AI in drug development and medical devices. Real-world examples, industry statistics, and forecasts illustrate both the benefits of AI (efficiency, speed, and data-driven insights) and the risks/limitations (bias, transparency, and ethical considerations) of AI adoption in regulatory affairs.
AI Applications in Regulatory Affairs
AI-Powered Regulatory Submissions
Regulatory submission preparation is a labor-intensive process requiring meticulous compilation of data (preclinical, clinical, manufacturing, etc.) into formats like the electronic Common Technical Document (eCTD). AI is now helping automate and optimize this process. For example, natural language processing (NLP) can review draft submissions to flag errors or inconsistencies that might delay approval (Revolutionizing Compliance: Regulatory Affairs Automation & AI - DDi). Machine learning algorithms can cross-check new drug applications against past approvals to ensure all required data and justifications are included (How AI Is Accelerating Drug Approvals-). In practice, AI tools are being used to generate and validate submission documents, reducing human error and administrative burden (How AI Is Accelerating Drug Approvals-). Early experiments with generative AI show promise in drafting portions of regulatory documents: one analysis found that large language model–based tools could auto-generate a first draft of a clinical study report in minutes, cutting writing time almost in half (Generative AI in the pharmaceutical industry-McKinsey) (Generative AI in the pharmaceutical industry-McKinsey). These AI-generated drafts (often “80% right”) still require human medical writers for polishing and complex clinical interpretation, but they significantly speed up the submission process (Generative AI in the pharmaceutical industry-McKinsey). According to McKinsey, such approaches could make regulatory submissions ~40% faster, halve the cost, and cut document quality issues by two-fold (Generative AI in the pharmaceutical industry-McKinsey). Regulators are beginning to embrace these efficiencies – the FDA and EMA have signaled openness to “AI-enhanced” submissions that can increase efficiency without compromising safety (How AI Is Accelerating Drug Approvals-). Notably, predictive AI is also being applied to anticipate regulators’ questions during review. AI-driven “regulatory intelligence engines” can analyze past FDA queries (so-called health authority queries, HAQs) to predict likely questions for a given submission and even help craft responses (Generative AI in the pharmaceutical industry-McKinsey) (Generative AI in the pharmaceutical industry-McKinsey). In simulations, this has led to ~30% faster response times and 50% fewer follow-up questions during FDA review (Generative AI in the pharmaceutical industry-McKinsey). By proactively addressing anticipated concerns, companies can potentially shorten review cycles and accelerate approvals. Overall, AI in regulatory submissions augments human teams by ensuring greater accuracy, consistency, and preparedness, ultimately aiming to speed new therapies to patients.
AI for Compliance Monitoring and Regulatory Intelligence
Staying compliant with constantly evolving regulations is a core challenge in pharma. AI has emerged as a game-changer for regulatory intelligence – the tracking of global regulatory changes, guidance, inspection findings, and industry trends to inform compliance. Many pharmaceutical companies now leverage NLP and data mining to monitor FDA databases, guidelines, and even publicly available documents for any changes that could impact their products (How Pharma Companies Are Solving Regulatory Challenges with AI-based Technology-American Pharmaceutical Review - The Review of American Pharmaceutical Business & Technology) (How Pharma Companies Are Solving Regulatory Challenges with AI-based Technology-American Pharmaceutical Review - The Review of American Pharmaceutical Business & Technology). Instead of staff manually checking various agency websites and email alerts, AI systems can ingest millions of data points from sources like FDA warning letters, new regulatory guidances, meeting minutes, and published white papers (How Pharma Companies Are Solving Regulatory Challenges with AI-based Technology-American Pharmaceutical Review - The Review of American Pharmaceutical Business & Technology). One large biopharma’s regulatory team built a centralized “data lake” integrating internal quality data (deviations, CAPAs, risks) with external regulatory data (FDA letters, biologics license review reports, etc.) (How Pharma Companies Are Solving Regulatory Challenges with AI-based Technology-American Pharmaceutical Review - The Review of American Pharmaceutical Business & Technology). They applied NLP to this data lake to extract critical concepts, relationships, and even sentiment (e.g. detecting negative regulatory trends) (How Pharma Companies Are Solving Regulatory Challenges with AI-based Technology-American Pharmaceutical Review - The Review of American Pharmaceutical Business & Technology). The result was a dashboard with real-time compliance risk indicators and recommendations, updated automatically as new data flows in (How Pharma Companies Are Solving Regulatory Challenges with AI-based Technology-American Pharmaceutical Review - The Review of American Pharmaceutical Business & Technology). This enabled proactive risk management across product formulation, supply chain, and regulatory compliance activities – essentially an AI-driven early warning system for compliance issues. Another pharma company created a regulatory Q&A chatbot by combining NLP with large language models (LLMs) (How Pharma Companies Are Solving Regulatory Challenges with AI-based Technology-American Pharmaceutical Review - The Review of American Pharmaceutical Business & Technology) (How Pharma Companies Are Solving Regulatory Challenges with AI-based Technology-American Pharmaceutical Review - The Review of American Pharmaceutical Business & Technology). This “regulatory intelligence assistant” lets team members ask questions in natural language (e.g. “What new FDA safety alerts came out this month for ingredient X?”) and get summarized answers drawing from up-to-date sources (How Pharma Companies Are Solving Regulatory Challenges with AI-based Technology-American Pharmaceutical Review - The Review of American Pharmaceutical Business & Technology). It can also categorize regulatory changes by risk level and highlight relevant requirements for specific compounds (How Pharma Companies Are Solving Regulatory Challenges with AI-based Technology-American Pharmaceutical Review - The Review of American Pharmaceutical Business & Technology). Such semi-automated monitoring has become mainstream – an estimated 90% of leading pharma and medtech companies use AI to analyze regulatory and inspection trends, reportedly saving hundreds of staff hours per month on compliance monitoring tasks (Using AI to Boost Efficiency and Ensure Regulatory Compliance - Experic CDMO). These tools help companies keep pace with FDA rule changes and guidance updates, ensuring they remain in continuous compliance. Importantly, AI is also being applied to internal compliance processes. Pattern-recognition algorithms can sift through manufacturing and audit data to spot deviations or documentation errors that humans might miss. GlaxoSmithKline (GSK), for instance, implemented an AI-powered compliance system that significantly reduced documentation errors during internal audits, thereby minimizing the risk of regulatory citations (Using AI to Boost Efficiency and Ensure Regulatory Compliance - Experic CDMO). By improving data accuracy and catching issues early, AI strengthens companies’ inspection readiness. Overall, AI-driven regulatory intelligence and compliance monitoring allow regulatory affairs teams to move from reactive to proactive mode – instead of scrambling to interpret new rules or fix audit findings after the fact, they can anticipate and address compliance requirements in near real-time.
AI in Pharmaceutical Labeling
Regulatory labeling – creating and updating the official prescribing information for drugs – is a complex process requiring absolute accuracy. Labels must reflect the latest safety, efficacy, and usage information, and even small errors or delays can trigger regulatory action. AI is now helping to streamline labeling processes while maintaining high precision. Generative AI and NLP tools can assist in drafting and reviewing label content. For example, an AI “copilot” can be fed a core data sheet or clinical study results and then generate draft text for sections of the label (such as indications, adverse effects, or dosing) (Implementing AI in Pharmaceutical Labeling Processes). One industry solution uses generative AI to propose label wording, then automatically notifies human label experts for review and approval – this human-in-the-loop approach ensures oversight of the AI’s suggestions (Implementing AI in Pharmaceutical Labeling Processes). Such tools can cut down the time spent writing or revising label sections, while still relying on experts to validate accuracy and tone. AI is also excellent at comparing and analyzing labeling documents. Large pharma companies operate in multiple markets and must synchronize US FDA labels with those in other regions (EMEA, etc.). AI-powered labeling platforms now aggregate drug label information from various regulatory authorities (FDA, EMA, etc.) into a searchable hub (How Pharma Companies Are Solving Regulatory Challenges with AI-based Technology-American Pharmaceutical Review - The Review of American Pharmaceutical Business & Technology). A leading drug developer built an “NLP-powered labeling intelligence hub” that lets its regulatory team quickly search and cross-compare labels across different countries and products (How Pharma Companies Are Solving Regulatory Challenges with AI-based Technology-American Pharmaceutical Review - The Review of American Pharmaceutical Business & Technology). This tool can, for instance, find how a particular adverse event is described in all versions of a label globally, or highlight differences in contraindications between the FDA-approved label and the European label (How Pharma Companies Are Solving Regulatory Challenges with AI-based Technology-American Pharmaceutical Review - The Review of American Pharmaceutical Business & Technology). By synthesizing disparate label sources, the AI hub helped the team identify needed updates and ensure consistency, significantly speeding up label revisions and approvals (How Pharma Companies Are Solving Regulatory Challenges with AI-based Technology-American Pharmaceutical Review - The Review of American Pharmaceutical Business & Technology). Another emerging use is AI-driven change impact analysis for labeling. When new data (like a safety signal or study result) emerges, AI can rapidly scan the existing label text to pinpoint sections that would be affected by that change (Implementing AI in Pharmaceutical Labeling Processes). For example, if an FDA alert adds a new contraindication, an AI tool could locate all relevant mentions in the current label (and related documents) that need updating. This ensures that critical updates (e.g. a newly identified risk or ingredient change) are quickly propagated across all labeling materials (Implementing AI in Pharmaceutical Labeling Processes). Furthermore, intelligent document processing algorithms are extracting key data from unstructured label documents (like PDFs of old package inserts or global Company Core Data Sheets). By structuring this information, companies can more easily populate databases and map label content to standard data models (for example, conforming to Identification of Medicinal Products standards) (How Pharma Companies Are Solving Regulatory Challenges with AI-based Technology-American Pharmaceutical Review - The Review of American Pharmaceutical Business & Technology) (How Pharma Companies Are Solving Regulatory Challenges with AI-based Technology-American Pharmaceutical Review - The Review of American Pharmaceutical Business & Technology). Overall, AI in labeling augments human expertise by handling the heavy data crunching – generating draft text, checking for consistency, translating and comparing multi-region labels – thereby allowing regulatory teams to focus on the judgment calls around phrasing and clinical implications. The end result is faster label updates, more consistent labeling worldwide, and reduced risk of compliance errors in drug packaging and documentation.
AI in Pharmacovigilance and Drug Safety
Pharmacovigilance (PV) – the monitoring of drug safety and adverse events post-market – was one of the earliest areas to embrace AI in pharma. The volume of safety data has exploded (tens of millions of adverse event reports, scientific literature, and even social media posts each year) making it impossible to manage with manual methods alone. Since 2014, Pfizer has been pioneering the use of AI to handle the influx of adverse event case reports (The Future of Pharmacovigilance: Applying AI and Other Tech to Monitor Medicine and Vaccine Safety-Pfizer). The company’s AI system automatically sorts and categorizes incoming case reports, extracting key information about the patient, drug, and event (The Future of Pharmacovigilance: Applying AI and Other Tech to Monitor Medicine and Vaccine Safety-Pfizer). Mundane tasks like data entry, coding of medical terms, and initial assessment are offloaded to the AI, which can process vast amounts of data without fatigue (The Future of Pharmacovigilance: Applying AI and Other Tech to Monitor Medicine and Vaccine Safety-Pfizer). Pfizer credits this AI-driven case processing for allowing them to cope with surges in reports (for example, during the COVID-19 vaccine rollout) while maintaining regulatory compliance on reporting timelines (The Future of Pharmacovigilance: Applying AI and Other Tech to Monitor Medicine and Vaccine Safety-Pfizer) (The Future of Pharmacovigilance: Applying AI and Other Tech to Monitor Medicine and Vaccine Safety-Pfizer). By automating repetitive work, Pfizer’s safety scientists are freed to focus on the more complex aspects of safety analysis and patient risk management (The Future of Pharmacovigilance: Applying AI and Other Tech to Monitor Medicine and Vaccine Safety-Pfizer). Many other pharma companies have adopted similar PV automation. For instance, Roche employs NLP to scan scientific literature and real-world data for adverse event signals (7 AI Tools Transforming Pharmacovigilance - Pharma Now). AI-based systems like VigiLanz, TCS ADD, and Oracle Empirica are widely used to detect safety signals from large databases (7 AI Tools Transforming Pharmacovigilance - Pharma Now). Common PV tasks now augmented by AI include: duplicate case detection (identifying if two reports refer to the same case), triage prioritization (predicting which cases are serious vs. minor), and even causality assessment suggestions for clinicians (Looking Ahead: Safety & Regulatory Compliance Trends in 2024 - IQVIA) (Looking Ahead: Safety & Regulatory Compliance Trends in 2024 - IQVIA). Notably, AI’s pattern recognition can sometimes flag subtle safety trends faster than traditional methods. The FDA itself has leveraged big data and algorithmic tools for post-market surveillance – the FDA’s Sentinel System uses automated algorithms to analyze electronic health records and insurance claims data for safety signals (What is the Role of AI in Pharmacovigilance? - Daffodil Software). This kind of AI-enabled surveillance can quickly check if an adverse event reported in the FDA database is also showing up at higher rates in broader healthcare data, strengthening signal detection. Apart from efficiency, a key benefit of AI in PV is consistency. AI systems apply the same criteria to every case, reducing variability in how different staff might interpret reports. This consistency improves the quality of safety data submitted to regulators. Machine translation is another AI tool in PV – it allows companies to instantly translate foreign adverse event reports into English for evaluation, which is critical for global companies monitoring safety across markets. Looking forward, “intelligent” PV systems are being designed to not just process cases but also to contextualize them. For example, experimental AI models combine adverse event reports with knowledge graphs of drug mechanisms and patient characteristics to help identify high-risk patient subsets or potential risk factors that merit label changes (Artificial Intelligence is Changing the Face of Pharmacovigilance) (AI-Driven Pharmacovigilance: Ensuring Safety Tomorrow). All these applications aim to bolster drug safety monitoring by making it more real-time, comprehensive, and predictive. Regulators are supportive – FDA officials have spoken about the need for AI in pharmacovigilance to manage growing data volumes and identify rare signals that manual review might miss (The Need for Artificial Intelligence in Pharmacovigilance - FDA). Ultimately, AI-enhanced PV should lead to earlier detection of safety issues and faster interventions (like safety communications or product label updates), improving patient protection.
AI in Quality Assurance and Manufacturing
Quality assurance (QA) in pharma – ensuring that drugs are consistently produced and tested to meet quality standards – is another domain where AI is leaving a mark. In manufacturing, AI-driven analytics enable real-time monitoring of production processes, helping catch deviations before they become quality defects (The Impact of AI on Pharmaceutical Quality Assurance). For example, pharmaceutical production lines now use machine vision systems powered by AI to inspect tablets or vials for defects. These systems can detect tiny cracks, discoloration, or packaging errors far more reliably than the human eye (How AI is Revolutionizing Quality Control in Pharma Manufacturing). By automating visual inspection, companies improve detection accuracy and speed, preventing faulty products from reaching patients. Merck & Co. recently applied a generative AI approach to its visual inspection process. By training on images of both good products and known defects, the AI could better distinguish true defects from false alarms. This led to a 50% reduction in “false rejects” on the production line (i.e. fewer good products were mistakenly thrown out as defective) (The Transformative Impact of Generative AI in Manufacturing ... - AWS) (Shwen Gwee on LinkedIn: Transforming Patient Care: Generative AI ...). Reducing false rejects not only cuts waste but also improves supply availability – more safe medicine makes it to the market rather than being discarded due to inspection errors.
AI is also used for process optimization and predictive quality. Advanced ML models can monitor process parameters (pressure, temperature, mixing times, etc.) and predict quality outcomes in real-time ([PDF] Use of AI in Pharmaceutical Quality and Operations). If the model detects a drift that correlates with out-of-specification results, it can alert operators to intervene or adjust parameters. Over time, such systems continuously learn and adjust, leading to ongoing process optimization and fewer batch failures (Using AI to Boost Efficiency and Ensure Regulatory Compliance - Experic CDMO). One review noted that AI in pharma manufacturing has delivered benefits like enhanced monitoring of products for consistent quality, reduced errors and waste, and ongoing process improvements (Using AI to Boost Efficiency and Ensure Regulatory Compliance - Experic CDMO). These improvements ultimately simplify adherence to Good Manufacturing Practice (GMP) standards (Using AI to Boost Efficiency and Ensure Regulatory Compliance - Experic CDMO). In quality control labs, AI-based software can automate analysis of test results, flag anomalies, and even suggest likely root causes by comparing against large datasets of historical batches. This speeds up investigations and corrective actions when a quality issue is encountered.
Beyond manufacturing, quality assurance of data and documentation is critical in regulatory affairs. Here too, AI assists by checking datasets for integrity and compliance. For instance, AI can reconcile clinical data points across various submissions or detect if any values look out of range (possibly indicating a data transcription error). Some companies are deploying AI “audit assistants” that review documents (SOPs, batch records, validation protocols) to ensure they meet regulatory formatting and content requirements, highlighting sections that might be non-compliant. By catching these issues before official audits, firms have fewer observations from regulators.
In summary, AI is elevating quality assurance by making monitoring more sensitive and predictive. Problems can be identified earlier – sometimes even before they occur (via prediction) – allowing proactive adjustments and reducing the risk of defective products or data integrity lapses. The pharmaceutical industry’s commitment to quality is seeing a boost from these “smart” systems that work alongside QA professionals to maintain the highest standards of product safety and efficacy.
Transforming FDA Interactions and the Drug Approval Pipeline
The infusion of AI into regulatory affairs is beginning to transform how pharmaceutical companies interact with the FDA and how efficiently new drugs move through the approval pipeline. One major impact is the potential for shorter review times and more streamlined communication with regulators. When regulatory submissions are prepared with AI support (leading to more complete dossiers and anticipating questions), the regulatory review process becomes smoother. As noted earlier, AI tools that predict and address likely FDA questions can reduce back-and-forth inquiries (Generative AI in the pharmaceutical industry-McKinsey). Fewer clarification questions mean the FDA can reach a decision sooner. Additionally, high-quality, error-free submissions (ensured by AI validation checks) require less time for FDA reviewers to scrutinize and request corrections, thereby accelerating the pipeline. Industry experts predict that widespread use of AI could significantly cut down drug development timelines – not only in R&D but also in the approval phase. Kinetica reports that AI-driven process improvements across trials and submissions have the potential to trim overall development time by up to 30% in some cases (How AI Is Accelerating Drug Approvals-) (How AI Is Accelerating Drug Approvals-). This translates to new medicines getting to patients faster than before.
AI is also changing how companies communicate data to the FDA. We are seeing the rise of dynamic, digital submission components, such as interactive datasets or AI-generated analyses included in applications. For example, a company might include an AI-powered analysis of real-world data as supplementary evidence of a drug’s safety. The FDA, in turn, is adapting to evaluate these novel data submissions. In 2023, FDA’s Center for Drug Evaluation and Research (CDER) stated it is increasingly open to sponsors using AI/ML in drug development and in submissions, provided the use is well-documented (How AI Is Accelerating Drug Approvals-). FDA review divisions have been training staff and developing capacity to assess AI-derived evidence. In some cases, companies have engaged in “FDA early engagement” when using novel AI approaches – proactively discussing their AI methodology with the agency before submission. This helps FDA reviewers become comfortable with the model and its validation, fostering a collaborative approach to oversight (FDA unveils long-awaited guidance on AI use to support drug and biologic development) (FDA unveils long-awaited guidance on AI use to support drug and biologic development).
On the FDA side, the agency itself is exploring AI tools to enhance its review and oversight duties. FDA researchers have worked on AI models (like AskFDA for querying drug labels) to help reviewers quickly find pertinent information in huge submissions (Leveraging FDA Labeling Documents and Large Language Model ...) (A framework enabling LLMs into regulatory environment for ...). In 2020, the FDA began developing an AI-based system to let reviewers query labeling documents using a custom language model, aiming to retrieve answers from thousands of pages of prior labels and submissions more efficiently (FDALabel: Full-Text Search of Drug Product Labeling-FDA). The FDA’s Office of Surveillance and Epidemiology is also known to employ data mining algorithms to analyze adverse event report trends (detecting safety signals that might prompt regulatory action). Such initiatives indicate that FDA reviewers might soon routinely use AI assistants to handle the growing volume and complexity of data. The result could be faster, more informed regulatory decisions.
Moreover, AI might transform post-approval interactions between industry and FDA. Pharma companies use AI to continuously monitor real-world evidence, and when signals arise, they can more rapidly report insights to the FDA (for instance, flagging an unexpected safety issue). This could lead to a more agile post-market surveillance system in partnership with regulators. The concept of “algorithmovigilance” has even been proposed, borrowing from pharmacovigilance – it refers to monitoring AI systems themselves for performance issues or errors once they’re deployed (Algorithmovigilance, lessons from pharmacovigilance-npj Digital Medicine) (Algorithmovigilance, lessons from pharmacovigilance-npj Digital Medicine). In the future, companies might be required to report on the performance and updates of critical AI models (e.g. those used in clinical trial monitoring or safety decision-making) as part of regulatory compliance, ensuring regulators stay informed about how AI is influencing drug development and safety.
In summary, AI is fostering a more data-rich and proactive engagement with the FDA. Companies armed with AI insights can engage regulators with better evidence and preparedness, while the FDA is modernizing its own toolset to keep pace. The drug approval pipeline stands to benefit through greater efficiency – possibly achieving approvals in a shorter time with maintained (or improved) rigor. Both sides – industry and FDA – will need to maintain close collaboration to ensure these technologies are used responsibly to serve public health.
Regulatory Guidance and Oversight for AI/ML in Pharma
As AI becomes integral to drug development and regulatory processes, regulatory bodies are issuing guidance to govern its use. The FDA has been actively developing a framework to ensure AI/ML tools are used safely and effectively in the pharmaceutical context. In early 2025, the FDA published its first draft guidance focused on the use of AI in drug development and regulatory decision-making (FDA unveils long-awaited guidance on AI use to support drug and biologic development). This draft guidance, titled “Considerations for the Use of Artificial Intelligence and Machine Learning in the Development of Drug and Biological Products,” provides recommendations to sponsors leveraging AI in various phases of the product lifecycle. Notably, the FDA made clear that the guidance applies to nonclinical, clinical, manufacturing, and post-marketing phases, but excludes drug discovery research and other internal uses not directly impacting product safety or study results (FDA unveils long-awaited guidance on AI use to support drug and biologic development). In other words, if a company uses AI to analyze clinical trial data, support efficacy/safety analysis, or control a manufacturing process – those uses fall under FDA’s purview, whereas using AI to find new drug targets does not (at least for now).
A centerpiece of FDA’s draft guidance is a 7-step risk-based framework for establishing the credibility of AI/ML models used in drug development (FDA unveils long-awaited guidance on AI use to support drug and biologic development). The FDA expects companies to rigorously assess and document that their AI tool is “fit for purpose” for its intended regulatory use. The steps include: (1) Defining the Question of Interest – clearly state what decision or task the AI model will inform (e.g. dose selection in a trial) (FDA unveils long-awaited guidance on AI use to support drug and biologic development); (2) Defining the Context of Use (COU) – outline exactly how and where the model will be applied in the development process and its scope (FDA unveils long-awaited guidance on AI use to support drug and biologic development); (3) Assessing Model Risk – evaluate how much influence the model’s output has on decisions and the potential consequences if the model is wrong (FDA unveils long-awaited guidance on AI use to support drug and biologic development). If an AI’s output could significantly impact patient safety or trial outcomes, it’s considered high-risk and demands more stringent validation (FDA unveils long-awaited guidance on AI use to support drug and biologic development). Steps 4 and 5 involve developing and executing a “Credibility Assessment Plan” (FDA unveils long-awaited guidance on AI use to support drug and biologic development). This is essentially a detailed validation plan covering the model’s design, data used, performance metrics, and risk mitigation strategies. The FDA encourages sponsors to engage early and even present this plan to the agency for feedback (FDA unveils long-awaited guidance on AI use to support drug and biologic development). The guidance outlines what to include: a thorough description of the model (inputs, architecture, algorithms), the data used for training and testing (with justification that the data is adequate and representative), and how biases or limitations in data have been addressed (FDA unveils long-awaited guidance on AI use to support drug and biologic development) (FDA unveils long-awaited guidance on AI use to support drug and biologic development). For instance, sponsors should discuss if certain patient groups were underrepresented in training data and how that is mitigated (FDA unveils long-awaited guidance on AI use to support drug and biologic development). They must also detail the model’s performance criteria and what level of error is acceptable given the context (tied back to the risk assessment). Steps 6 and 7 involve implementing the model and monitoring it throughout its lifecycle. The FDA expects a lifecycle management plan for the AI model, meaning even after the model is used to support an approval, it should be monitored and maintained if it will continue to be used (e.g. in post-market analyses) (FDA unveils long-awaited guidance on AI use to support drug and biologic development). Any updates to the model or degradation in performance over time should be managed under this plan (FDA unveils long-awaited guidance on AI use to support drug and biologic development). The overall goal of this framework is to ensure that AI models influencing regulatory decisions are transparent, well-validated, and reliable, much like any lab test or statistical method would need to be. By following these steps and documenting them in a “Credibility Assessment Report,” sponsors can give FDA confidence in their AI’s results (FDA unveils long-awaited guidance on AI use to support drug and biologic development). The guidance explicitly emphasizes addressing algorithmic bias, data quality, and explaining the model’s outputs in a way regulators can understand (FDA unveils long-awaited guidance on AI use to support drug and biologic development) (FDA unveils long-awaited guidance on AI use to support drug and biologic development). This reflects FDA’s broader commitment to trustworthy AI, aligning with principles like transparency, explainability, and robustness.
When it comes to AI/ML-enabled medical devices, the FDA has also been proactive. In January 2025, the FDA released a draft guidance on “Artificial Intelligence-Enabled Device Software Functions” to guide submissions for AI-based devices (FDA Releases Draft Guidance on Submission Recommendations for ...). This is particularly relevant for software as a medical device (SaMD) that utilizes machine learning (for example, an AI diagnostic app). The FDA recommends that sponsors of AI-based devices include detailed documentation in their marketing submissions about the model’s training, testing, and performance. They even provide an example “model card” format in the guidance, which standardizes how to report an AI model’s details and intended use (FDA Releases Draft Guidance on Submission Recommendations for AI-Enabled Device Software Functions - King & Spalding) (FDA Releases Draft Guidance on Submission Recommendations for AI-Enabled Device Software Functions - King & Spalding). Transparency is key – FDA wants a clear description of the algorithm, the dataset it was developed on, and its limitations (FDA Releases Draft Guidance on Submission Recommendations for AI-Enabled Device Software Functions - King & Spalding). Specifically, they highlight the importance of addressing risks related to users understanding and interpreting AI outputs (FDA Releases Draft Guidance on Submission Recommendations for AI-Enabled Device Software Functions - King & Spalding). For medical devices, if an AI provides a prediction or result, the labeling (user interface) should help the healthcare provider or user grasp the level of certainty and any caveats. The guidance also pushes for considerations of “performance drift” – AI models might perform well initially but could degrade as data in the real world evolves. Sponsors are encouraged to have a post-market monitoring plan to detect and correct any drift in an AI device’s performance (FDA Releases Draft Guidance on Submission Recommendations for AI-Enabled Device Software Functions - King & Spalding). This might involve periodic re-validation or updates. Additionally, the FDA has introduced the concept of a “predetermined change control plan” for AI devices – allowing manufacturers to get advance FDA approval for how their model can learn and update over time within set boundaries, rather than needing a new submission for every minor model change. Although our focus is pharma (drug development), these device guidelines reflect the FDA’s general approach to AI: requiring thorough documentation, risk management, and plans for ongoing oversight.
Beyond FDA, other bodies and international regulators are also shaping AI governance in pharma. The FDA has collaborated with Health Canada and the UK’s MHRA on guiding principles for Good Machine Learning Practice (GMLP) in medical technology, emphasizing things like algorithm transparency and robustness (Healthcare Industry News Weekly Wrap-Up: June 20, 2024-Vault Bioventures). In the EU, the Artificial Intelligence Act (the first broad AI law) will impose requirements from 2024/2025 on AI used in high-risk domains including healthcare (Using AI to Boost Efficiency and Ensure Regulatory Compliance - Experic CDMO) (Using AI to Boost Efficiency and Ensure Regulatory Compliance - Experic CDMO). While the AI Act is EU-focused, multinational pharma companies will likely align with its standards (such as risk assessment, documentation, and human oversight for AI systems). This global regulatory convergence means U.S. companies should anticipate more formal regulations on AI in the near future. As one pharma regulatory expert noted, comprehensive AI regulations in the U.S. are expected – focusing on limitations for AI in clinical decision-making, transparency, and cybersecurity/privacy protections (Regulatory Concerns for AI: 2024 Trends) (Regulatory Concerns for AI: 2024 Trends). In anticipation, companies are already adopting best practices frameworks (like the NIST AI Risk Management Framework and ISO AI standards) to guide responsible AI use (Healthcare Industry News Weekly Wrap-Up: June 20, 2024-Vault Bioventures). The FDA’s draft guidances are currently recommendations, but they signal the direction of formal policy. Industry stakeholders are closely watching these developments to ensure their AI deployments in regulatory affairs will meet the forthcoming expectations. In summary, the regulatory oversight is evolving to keep AI on a leash: encouraging innovation that can speed drug development, but under a structure that assures quality, accountability, and ethics in AI outputs.
Real-World Use Cases and Industry Examples
To illustrate the impact of AI in regulatory affairs, the table below summarizes key use cases, companies implementing them, and reported outcomes:
AI Use Case | Company / Implementation | Outcomes / Benefits |
---|---|---|
Regulatory Submission Automation | Multiple pharma (pilot programs) – Using generative AI to draft clinical study reports and summarize data for submissions (Generative AI in the pharmaceutical industry-McKinsey) (Generative AI in the pharmaceutical industry-McKinsey). McKinsey analysis of industry adoption. | – Drafting time for certain submission documents cut ~50% (Generative AI in the pharmaceutical industry-McKinsey). – Overall regulatory submission timelines potentially 40% faster (Generative AI in the pharmaceutical industry-McKinsey). – 2× reduction in document quality issues through AI QC checks (Generative AI in the pharmaceutical industry-McKinsey). |
Regulatory Intelligence & Compliance | Large Biopharma (unidentified via IQVIA) – Integrated data lake of internal (CAPAs, deviations) & external (FDA letters, guidelines) data with NLP analytics (How Pharma Companies Are Solving Regulatory Challenges with AI-based Technology-American Pharmaceutical Review - The Review of American Pharmaceutical Business & Technology) (How Pharma Companies Are Solving Regulatory Challenges with AI-based Technology-American Pharmaceutical Review - The Review of American Pharmaceutical Business & Technology). Top 10 Pharmas (90% of industry) – Semi-automated monitoring of regulatory changes using AI (Using AI to Boost Efficiency and Ensure Regulatory Compliance - Experic CDMO). | – Proactive risk identification from combined data sources, providing “actionable intelligence” to teams (Using AI to Boost Efficiency and Ensure Regulatory Compliance - Experic CDMO) (Using AI to Boost Efficiency and Ensure Regulatory Compliance - Experic CDMO). – Hundreds of hours saved monthly per company in manual monitoring efforts (Using AI to Boost Efficiency and Ensure Regulatory Compliance - Experic CDMO). – Fewer compliance gaps: e.g. GSK’s AI system cut documentation errors in audits, reducing regulatory findings (Using AI to Boost Efficiency and Ensure Regulatory Compliance - Experic CDMO). |
Labeling and Document Management | Leading Drug Developer (via IQVIA) – NLP-powered labeling content hub aggregating FDA/EMA labels for comparison (How Pharma Companies Are Solving Regulatory Challenges with AI-based Technology-American Pharmaceutical Review - The Review of American Pharmaceutical Business & Technology). Appian AI Labeling Suite – GenAI for label text generation with human review (Implementing AI in Pharmaceutical Labeling Processes). | – Streamlined label updates: faster reference checking across markets, expediting new label creation and updates (How Pharma Companies Are Solving Regulatory Challenges with AI-based Technology-American Pharmaceutical Review - The Review of American Pharmaceutical Business & Technology). – Improved consistency across global product labels, aiding faster approvals of changes (How Pharma Companies Are Solving Regulatory Challenges with AI-based Technology-American Pharmaceutical Review - The Review of American Pharmaceutical Business & Technology). – Draft label sections produced in minutes, freeing experts to focus on verification (Implementing AI in Pharmaceutical Labeling Processes). |
Pharmacovigilance (Adverse Event Processing) | Pfizer – In-house AI since 2014 for adverse event case intake and triage (The Future of Pharmacovigilance: Applying AI and Other Tech to Monitor Medicine and Vaccine Safety-Pfizer). Roche – NLP to scan literature and social media for safety signals (7 AI Tools Transforming Pharmacovigilance - Pharma Now). Multiple (industry tools: VigiLenz, ArisGlobal LifeSphere, etc.) (7 AI Tools Transforming Pharmacovigilance - Pharma Now). | – Scaled to handle surges (e.g. COVID-19 vaccine reports) without proportional staff increase, while meeting reporting compliance (The Future of Pharmacovigilance: Applying AI and Other Tech to Monitor Medicine and Vaccine Safety-Pfizer) (The Future of Pharmacovigilance: Applying AI and Other Tech to Monitor Medicine and Vaccine Safety-Pfizer). – Faster case processing and initial assessment, focusing human experts on serious cases (improved productivity) (The Future of Pharmacovigilance: Applying AI and Other Tech to Monitor Medicine and Vaccine Safety-Pfizer). – Earlier detection of safety trends from real-world data, enabling prompt regulatory safety communications. |
Quality Assurance & Manufacturing QA | Merck & Co. – Vision AI to inspect products, using generative AI to reduce false rejects (The Transformative Impact of Generative AI in Manufacturing ... - AWS). Novartis (and peers) – AI to monitor production parameters and predict quality issues (various pilot projects). GSK – Automated QA documentation checks (internal compliance AI system) (Using AI to Boost Efficiency and Ensure Regulatory Compliance - Experic CDMO). | – 50%+ reduction in false rejection of good products, improving yield and supply availability (The Transformative Impact of Generative AI in Manufacturing ... - AWS) (Shwen Gwee on LinkedIn: Transforming Patient Care: Generative AI ...). – Real-time quality monitoring leading to fewer batch failures and reduced waste (Using AI to Boost Efficiency and Ensure Regulatory Compliance - Experic CDMO). – Enhanced cGMP compliance: consistent oversight of processes and documents, resulting in smoother FDA inspections and approvals. |
Table: Key AI Use Cases in Regulatory Affairs – Examples of Implementation by Pharma Companies and Outcomes.
These examples demonstrate tangible improvements. For instance, Pfizer’s case shows how AI helps manage pharmacovigilance at scale, and Merck’s example highlights quality gains in manufacturing. Many companies initially start with narrow AI pilots (like automating one step of submissions or one aspect of PV) and then expand once benefits are proven. Importantly, the outcomes are measured not just in efficiency, but in better compliance (fewer errors, faster detection of issues) which has direct regulatory impact. While results vary by organization, common trends are emerging: significant time savings, reduction in manual workload, improved accuracy, and data-driven insights that were not previously possible. These outcomes collectively strengthen a company’s regulatory position – submissions are more robust, compliance is demonstrable, and safety monitoring is rigorous – all of which can increase trust with regulators and expedite regulatory processes.
Risks, Limitations, and Ethical Considerations
Despite the optimism around AI in regulatory affairs, it is crucial to acknowledge the risks, limitations, and ethical issues involved. AI systems are only as good as the data and design behind them, and in a high-stakes domain like pharma, a flawed AI output can have serious consequences. One major concern is bias and fairness. AI algorithms trained on historical data may inadvertently learn biases. In healthcare, there have been instances of AI models exhibiting racial or gender bias in diagnostics (Regulatory Hurdles and Ethical Concerns in FDA Oversight of AI/ML Medical Devices). In regulatory affairs, bias might mean an AI tool that under-prioritizes safety cases from certain demographics because of underrepresentation in training data – a dangerous blind spot. Recognizing this, the FDA’s guidance explicitly calls for sponsors to address potential algorithmic bias and data representativeness when using AI (FDA unveils long-awaited guidance on AI use to support drug and biologic development) (FDA unveils long-awaited guidance on AI use to support drug and biologic development). Ethical use of AI demands that companies ensure their training datasets are diverse and that they test models for biased outcomes.
Another concern is transparency (“explainability”). Many AI models, especially deep learning, act as “black boxes” that output decisions without clear rationale. In regulatory contexts, lack of explainability is problematic – regulators and industry professionals need to understand why an AI flagged a submission section as non-compliant or how an AI decided a case was non-serious. Without transparency, trust in AI outputs is low. The FDA has emphasized transparency in AI-enabled device guidance, suggesting use of model “factsheets” or cards to clearly explain an AI’s function and limitations (FDA Releases Draft Guidance on Submission Recommendations for AI-Enabled Device Software Functions - King & Spalding) (FDA Releases Draft Guidance on Submission Recommendations for AI-Enabled Device Software Functions - King & Spalding). Similarly, pharma companies are adopting “explainable AI” techniques so that any recommendation the AI makes (e.g. identifying a risk in a data set) can be traced to supporting evidence or logic. A related issue is validation – in regulated industries, any software used in processes must be validated for its intended use. AI’s probabilistic nature makes validation challenging. It’s not enough for an AI to work on average; companies must show it meets consistent performance standards and define its acceptable error rates. If an AI fails to flag a critical issue in a submission, the company remains accountable. Thus, rigorous testing (including worst-case scenarios) is needed before trusting AI in regulatory decisions. FDA’s draft framework essentially pushes companies to do just that – treat AI models with the same rigor as lab assays, including continuous monitoring for performance drift (FDA Releases Draft Guidance on Submission Recommendations for AI-Enabled Device Software Functions - King & Spalding).
Ethical considerations also extend to privacy and data protection. AI often requires large datasets, which in pharma may include sensitive patient information (clinical trial data, medical records, etc.). Ensuring compliance with privacy laws (HIPAA, GDPR) while using AI is mandatory. There is a risk that AI systems, if not secure, could be hacked or could inadvertently expose sensitive data. The pharmaceutical industry is aware of these cybersecurity risks – any AI system connected to the internet or cloud must have robust protections, and FDA has indicated it will scrutinize cybersecurity, especially for AI in devices (Regulatory Concerns for AI: 2024 Trends). Patient consent and awareness is another facet: if patient data is used to train AI, patients might have an ethical right to know and consent to that use.
Another risk is over-reliance on AI or “automation complacency.” Regulatory professionals bring critical judgment that an AI cannot replicate. For example, an AI might miss a context that a human would catch (such as a subtle implication of a regulation on a unique product scenario). If teams blindly trust AI outputs without cross-checking, errors could slip through. AI should thus be used as an assistant, not a replacement, for expert judgment. Leading companies have stressed that human oversight is essential – AI outputs in regulatory affairs are typically reviewed by subject matter experts before decisions are made (Looking Ahead: Safety & Regulatory Compliance Trends in 2024 - IQVIA) (Looking Ahead: Safety & Regulatory Compliance Trends in 2024 - IQVIA). The ideal is a human-AI collaboration where AI handles grunt work and humans do the critical thinking. This balance mitigates the risk of AI mistakes causing harm.
There are also broader ethical debates: For instance, if AI helps a company get a drug approved faster, did it also ensure that all safety considerations were as thoroughly vetted? Speed should not come at the cost of diligence. Regulators will be watching that AI isn’t used to game the system or gloss over issues. Another topic is employment and skills – as AI automates certain regulatory tasks, companies need to manage the workforce transition ethically, upskilling regulatory professionals to work alongside AI rather than rendering roles obsolete. In reality, the role of regulatory professionals is evolving (focus on higher-level strategy while AI does routine tasks), and demand for AI-fluent talent in regulatory affairs is growing (How AI Is Accelerating Drug Approvals-).
Finally, the concept of “algorithmic accountability” is emerging: if an AI causes an error (say, an important warning was left out of a label because AI summary missed it), who is accountable? The company cannot blame the machine – regulators will hold the company responsible. Thus, firms must implement strict quality controls and possibly “AI audit trails” to document how AI tools are used in each decision. Some have suggested adopting practices akin to pharmacovigilance for AI itself (“algorithmovigilance”) – continuous monitoring and reporting of AI performance issues (Algorithmovigilance, lessons from pharmacovigilance-npj Digital Medicine) (Algorithmovigilance, lessons from pharmacovigilance-npj Digital Medicine). In the event of an AI-related mishap, having audit logs and monitoring could help identify and correct the issue, and demonstrate to regulators that the company manages its AI responsibly.
In summary, while AI offers powerful benefits, the pharmaceutical industry and regulators are keenly aware of the pitfalls. Ensuring fairness, maintaining transparency, validating performance, and keeping humans in the loop are key strategies to mitigate AI risks. Ethical use of AI in regulatory affairs is not just a nice-to-have; it’s necessary to maintain the trust of regulators, healthcare providers, and patients. The technologies may be cutting-edge, but the age-old principles of patient safety and data integrity remain paramount.
Conclusion and Outlook
AI’s impact on regulatory affairs in the U.S. pharma industry is profound and growing. What started as experimental pilots a few years ago is now moving into the mainstream of how companies handle regulatory submissions, compliance, labeling, pharmacovigilance, and quality. Current applications show that AI can take on laborious tasks – compiling documents, monitoring endless data streams, writing initial drafts – with speed and accuracy that significantly augment human capabilities. This augmentation is already yielding faster submissions, more robust compliance oversight, and more vigilant safety monitoring. Emerging applications promise even more transformation: from AI copilots that help write entire sections of an NDA/BLA, to predictive models that map out the most efficient approval strategy or highlight which real-world evidence could strengthen a filing.
The FDA and other regulators are generally receptive to AI innovation, seeing its potential to improve efficiencies, but they are rightly cautious. Through draft guidances and inter-agency collaborations, regulators are staking expectations that AI be used responsibly – validating models, controlling risks, and ensuring transparency. As these guidances solidify, we can expect clearer rules of the road. Companies that invest now in strong AI governance (data governance, model validation, and ethical AI practices) will be well-positioned to meet future regulatory requirements and even shape industry standards. On the flip side, companies that deploy AI without proper controls may face regulatory setbacks if an AI-driven error occurs or if they cannot satisfy FDA’s documentation requests about their AI tools.
Looking ahead, industry analysts foresee that by the end of this decade AI could become embedded across the drug regulatory lifecycle. Routine regulatory interactions might involve AI – for example, companies could submit data in new AI-readable formats, and FDA reviewers might use AI to assist in reviewing. The review process might also shorten as certain checks become automated. There’s even a vision of real-time regulatory oversight: continuous data flow from manufacturing or post-market monitoring to regulators via AI analytics, moving away from periodic submissions toward a more ongoing assurance of compliance. While such a paradigm is still evolving, it underscores a future where AI enables a more dynamic, data-driven regulatory environment.
The benefits will need to be balanced with vigilance on the risks. Stakeholders must continue to collaborate – pharma companies, FDA, technology providers, and standards organizations – to ensure that AI tools are thoroughly evaluated and fit-for-purpose. Ethical frameworks and possibly new regulations (e.g., an FDA guidance that becomes a rule, or legislation on AI in healthcare) will likely solidify. IT professionals in pharma will play a crucial role: they are the bridge between data science and regulatory teams, tasked with implementing AI solutions that comply with GxP requirements and are audit-ready. This means incorporating features like audit logs, access controls, model version control, and validation documentation into AI platforms used for regulatory work.
In conclusion, AI is not replacing the need for regulatory expertise – rather, it is amplifying it. By handling the heavy data lifting and routine analyses, AI allows regulatory professionals to focus on strategy, decision-making, and ensuring that the spirit of regulations (protecting patients and ensuring drug efficacy/quality) is upheld. The U.S. regulatory landscape is evolving alongside AI advancements: cautious but optimistic. With robust implementation, AI can be the catalyst for a more efficient regulatory process that brings medicines to market faster without compromising safety or compliance. As one industry attorney observed, the adoption of AI in pharma is only accelerating, and we can expect greater investment and guidance in the coming years to fully capture its benefits while safeguarding public health (Regulatory Concerns for AI: 2024 Trends) (Regulatory Concerns for AI: 2024 Trends). The journey is ongoing, but the trajectory is clear – AI will be an indispensable component of regulatory affairs, shaping a future where innovation and regulation advance hand in hand.
Sources: Reliable sources such as regulatory agency publications, peer-reviewed journals, and leading industry analysis were referenced in this report. Key references include FDA draft guidances on AI (FDA unveils long-awaited guidance on AI use to support drug and biologic development) (FDA unveils long-awaited guidance on AI use to support drug and biologic development), insights from industry experts and surveys (IQVIA, McKinsey) (Looking Ahead: Safety & Regulatory Compliance Trends in 2024 - IQVIA) (Generative AI in the pharmaceutical industry-McKinsey), and case studies reported in pharmaceutical literature (How Pharma Companies Are Solving Regulatory Challenges with AI-based Technology-American Pharmaceutical Review - The Review of American Pharmaceutical Business & Technology) (The Future of Pharmacovigilance: Applying AI and Other Tech to Monitor Medicine and Vaccine Safety-Pfizer). These citations highlight both the current state and the forward-looking expert consensus on AI in regulatory affairs. All evidence points to a future where AI is integral to regulatory functions – provided we implement it responsibly – ultimately strengthening the pharma industry’s ability to deliver safe, effective therapies to the public efficiently.
DISCLAIMER
The information contained in this document is provided for educational and informational purposes only. We make no representations or warranties of any kind, express or implied, about the completeness, accuracy, reliability, suitability, or availability of the information contained herein. Any reliance you place on such information is strictly at your own risk. In no event will IntuitionLabs.ai or its representatives be liable for any loss or damage including without limitation, indirect or consequential loss or damage, or any loss or damage whatsoever arising from the use of information presented in this document. This document may contain content generated with the assistance of artificial intelligence technologies. AI-generated content may contain errors, omissions, or inaccuracies. Readers are advised to independently verify any critical information before acting upon it. All product names, logos, brands, trademarks, and registered trademarks mentioned in this document are the property of their respective owners. All company, product, and service names used in this document are for identification purposes only. Use of these names, logos, trademarks, and brands does not imply endorsement by the respective trademark holders. IntuitionLabs.ai is an AI software development company specializing in helping life-science companies implement and leverage artificial intelligence solutions. Founded in 2023 by Adrien Laurent and based in San Jose, California. This document does not constitute professional or legal advice. For specific guidance related to your business needs, please consult with appropriate qualified professionals.