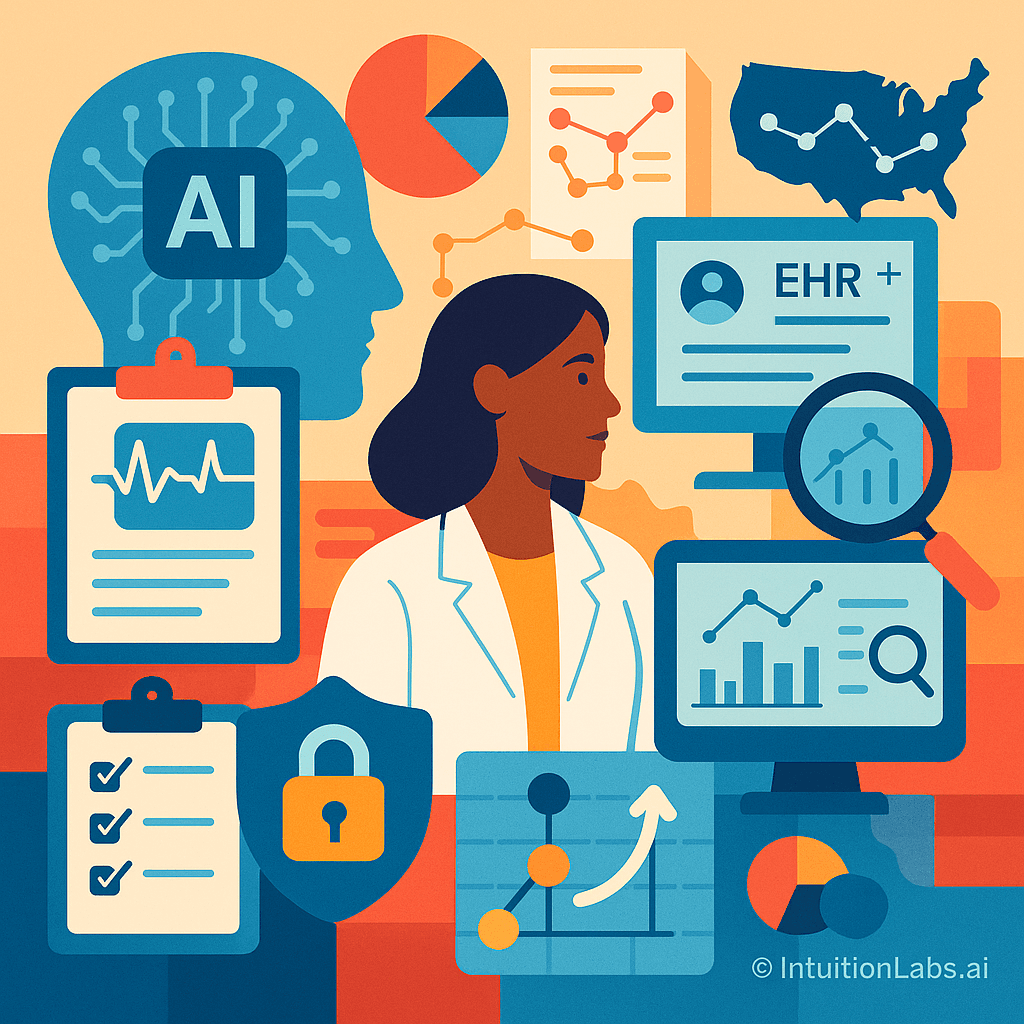
Impact of Artificial Intelligence on Clinical Data Management in the United States
Introduction
Artificial intelligence (AI) is increasingly transforming how clinical data is collected, managed, and utilized across U.S. healthcare and pharmaceutical sectors. From automating electronic health record (EHR) documentation to optimizing clinical trial operations, AI-driven tools are being adopted at a rapid pace. In 2024, roughly 66% of U.S. physicians reported using some form of health AI, a dramatic rise from 38% the year before (2 in 3 physicians are using health AI—up 78% from 2023-American Medical Association). Health systems likewise are embracing AI – a recent survey found 86% of U.S. healthcare organizations leverage AI in their operations (HIMSS and Medscape Unveil Groundbreaking Report on AI ...). This report provides an in-depth look at current AI applications in clinical data management (with a focus on EHRs, clinical trials, real-world evidence, data standardization, and predictive analytics), examines the benefits and challenges, and discusses U.S.-specific regulatory and ethical considerations (FDA guidelines, HIPAA compliance, etc.). We also highlight leading AI tools and vendors in this space. Short data-driven case studies and statistics are included to illustrate AI’s impact on efficiency, data quality, and compliance.
AI in Electronic Health Records and Clinical Documentation
One of the most mature applications of AI in clinical data management is within electronic health record (EHR) systems. Hospitals and clinics are using AI to streamline documentation, coding, and information retrieval in EHRs:
- Automated Clinical Documentation: Speech recognition and natural language processing (NLP) “scribes” now capture physicians’ notes and integrate them into EHRs. For example, ambient voice assistants (like Nuance DAX and Suki) listen to doctor-patient conversations and generate structured visit notes automatically. Rush University Medical Center found that deploying an AI assistant for documentation cut time spent on writing clinical notes by 72% (RUSH Deepens its AI Efforts By Teaming Up with Suki to Tackle Clinician Burnout - Suki AI), dramatically reducing physicians’ administrative burden. At Northwestern Medicine, an ambient AI documentation tool enabled doctors to see ~11 more patients per month by saving 24% of time on note-taking (A year of DAX Copilot: Healthcare innovation that refocuses on the clinician-patient connection - The Official Microsoft Blog). These tools can also improve quality – in one rollout, 77% of clinicians said AI improved their documentation quality (A year of DAX Copilot: Healthcare innovation that refocuses on the clinician-patient connection - The Official Microsoft Blog). As a result, many providers report lower burnout and “pajama time” (after-hours charting) when using AI-driven documentation aids.
- Clinical Data Coding and Entry: AI systems help populate structured fields and code clinical data. Machine learning models can auto-suggest billing codes or problem list entries from a doctor’s free-text note. For instance, an AI medical coding system integrated with EHRs achieved 96% accuracy in auto-assigning standard diagnostic codes (MedDRA) when set to high-confidence mode (AI in Clinical Data Management: Coding & Reconciliation). This kind of accuracy significantly reduces manual coding effort while maintaining data quality. By one estimate, automating code assignment and data entry saves tens of hours per clinician each month (AI in Clinical Data Management: Coding & Reconciliation).
- Information Retrieval and Decision Support: AI also enhances how clinicians retrieve and use EHR data. NLP-driven search tools can pull up relevant patient history or lab results with simple queries, saving time navigating complex records. Some EHRs embed predictive analytics that alert providers to at-risk patients (for example, early sepsis warning scores or readmission risk predictions). Notably, Epic Systems (the largest U.S. EHR vendor) has a built-in sepsis prediction model deployed at hundreds of hospitals nationwide (Epic's widely used sepsis prediction model falls short among Michigan Medicine patients-Fierce Healthcare) (Epic's widely used sepsis prediction model falls short among Michigan Medicine patients-Fierce Healthcare). This model scans patient vitals and notes to flag possible sepsis cases for clinicians. While such AI-driven decision support can improve outcomes (early intervention for sepsis can save lives), it also illustrates challenges – an independent study found Epic’s model had only ~33% sensitivity in practice (Epic's widely used sepsis prediction model falls short among Michigan Medicine patients-Fierce Healthcare), missing many true sepsis cases and highlighting the need for careful validation. Still, when properly tuned, AI-based risk scores and recommendations in EHRs have helped some hospitals reduce adverse events and even cut hospital length of stay by ~6.5% in certain implementations (AI for EHR: A Transformation in the Making).
Overall, AI in the EHR context is improving efficiency and data accuracy. Physicians increasingly use AI for routine tasks like documentation, coding, and translating clinical notes – in 2024, 21% of physicians said they use AI for documentation and billing code capture (up from 13% a year prior) (2 in 3 physicians are using health AI—up 78% from 2023-American Medical Association). This automation not only saves time but also standardizes data, which helps with downstream analytics and billing compliance. The immediate benefits in U.S. healthcare settings include more face time with patients (as doctors spend less time typing), more complete records, and fewer documentation errors. However, healthcare providers must ensure these AI tools are integrated seamlessly into workflows and that clinicians trust the AI outputs.
AI in Clinical Trials Data Management
Pharmaceutical companies and clinical research organizations are leveraging AI to modernize clinical trial data management, aiming to speed up trials and improve data quality. Key applications include:
- Patient Recruitment and Trial Design: Recruiting eligible patients is often a bottleneck in trials. AI algorithms can mine EHR databases and real-world data to find patients who match complex trial criteria, potentially improving enrollment speed. Machine learning models can also predict which trial sites will enroll patients fastest. According to a McKinsey analysis, using AI to select optimal trial sites and focus recruitment efforts has boosted enrollment rates by 10–15% on average for sponsors (Performance in clinical development with AI & ML-McKinsey). AI-driven site selection can identify top-performing hospitals/clinics 30–50% more effectively than traditional methods (Performance in clinical development with AI & ML-McKinsey), helping trials get fully enrolled months faster. These gains translate to significant time and cost savings – compressing the clinical timeline by even 6 months per drug can add on the order of $400 million in value for a sponsor (Performance in clinical development with AI & ML-McKinsey) (by getting a new therapy to market sooner).
- Automated Data Cleaning and Monitoring: During trials, huge volumes of data (patient visits, labs, adverse events, etc.) are collected in electronic data capture (EDC) systems. AI is now used to continuously clean and audit trial data. For example, AI-based anomaly detection can flag outlier data points or errors in real-time, reducing the number of manual queries that data managers must issue to investigative sites. In medical coding of trial data (e.g. coding reported adverse events to standardized terms), AI is already highly effective: one platform reported that AI auto-coding can save ~69 hours per 1,000 medical terms coded by performing the task in seconds rather than minutes per item (AI in Clinical Data Management: Coding & Reconciliation). The auto-coding suggestions are accurate enough that they often need no correction – in fact, with a human-in-the-loop review, high-confidence AI code assignments matched expert human coders 96% of the time (AI in Clinical Data Management: Coding & Reconciliation). Similarly, AI-powered data reconciliation tools automatically cross-check different data sources (e.g. comparing an adverse event log vs. medication log to ensure consistency) and flag discrepancies. This has been shown to save many hours of manual review and catch issues that might be overlooked otherwise (AI in Clinical Data Management: Coding & Reconciliation). Overall, AI is improving the completeness and integrity of clinical trial datasets while reducing labor for data management teams.
- AI “Copilots” for Trial Operations: Leading companies are implementing AI copilots or “control towers” to assist trial managers in overseeing ongoing studies. These AI agents aggregate real-time trial metrics (enrollment, data entry status, query resolution, etc.) and can prioritize which sites or patients need attention. For example, a generative AI–driven trial management assistant can draft targeted emails to sites that are lagging and even learn which interventions (a phone call, an extra training, etc.) tend to resolve delays (Performance in clinical development with AI & ML-McKinsey). Such tools help trial managers focus on what matters, potentially preventing delays. AI can also forecast problems – one system can predict, mid-trial, if enrollment will fall behind or if certain data quality issues will occur, allowing proactive corrective action (Performance in clinical development with AI & ML-McKinsey).
- Document Generation and Analysis: Another impactful use of AI in trials is automating the creation of clinical documents required for regulatory submissions. Writing Clinical Study Reports (CSRs), which summarize trial results, is traditionally a tedious process taking weeks. Generative AI has sped this up dramatically. Pharma companies have used genAI to produce first-draft CSRs 40% faster, cutting the writing process from 8–14 weeks down to ~5 weeks (Performance in clinical development with AI & ML-McKinsey). Impressively, the AI-generated drafts reached 98% accuracy compared to human-written reports, with fewer errors noted (Performance in clinical development with AI & ML-McKinsey). In one case, a company reduced the initial drafting time from 3 weeks to just 3 days by using an AI writer (Performance in clinical development with AI & ML-McKinsey). This high degree of accuracy and time savings allows human medical writers to focus on fine-tuning and interpreting results rather than rote documentation. Encouraged by this success, firms are now extending genAI to draft informed consent forms, safety update reports, and other trial documents (Performance in clinical development with AI & ML-McKinsey). AI is thus accelerating the generation of critical trial documentation while maintaining compliance with regulatory standards for clarity and accuracy.
Real-world impact: The integration of AI in clinical trials is already shaving significant time off drug development. By one estimate, combining various AI/ML techniques in trial operations (from site selection to data cleaning to document drafting) has shortened trial timelines by about 6 months per asset on average (Performance in clinical development with AI & ML-McKinsey). Faster trials not only reduce costs but deliver new treatments to patients sooner. Additionally, AI improves data quality – for instance, AI-assisted monitoring can increase protocol compliance and even enhance patient diversity in trials by identifying underrepresented groups and optimizing eligibility criteria (Performance in clinical development with AI & ML-McKinsey). The FDA has recognized these trends, noting that AI has “transformative potential to advance clinical research and accelerate product development” when proper safeguards are in place (FDA Issues Draft Guidance on the Role of Artificial Intelligence in Supporting Regulatory Decisions). The U.S. regulatory environment (discussed later) is evolving to accommodate such AI-driven innovations in the clinical trial process.
AI in Real-World Evidence (RWE) Generation
Outside of controlled trials, real-world data (RWD) from electronic health records, insurance claims, patient registries, and wearable devices are increasingly mined to generate real-world evidence (RWE) about treatments. AI is a crucial enabler in this arena, helping to make sense of vast, messy healthcare datasets:
- Extracting Outcomes from EHRs: Much of RWD is locked in unstructured formats (free-text physician notes, PDF reports, etc.). AI, particularly NLP, is being used to curate and structure this data at scale. For example, Flatiron Health – known for its oncology RWE datasets – employs machine learning and NLP to comb through oncology clinic EHRs and pull out critical details like tumor progression dates, biomarker results, or treatment responses (Flatiron Health’s AI Breakthrough: Accurate, Analyzable EHR Data Extraction-Inside Precision Medicine). In a recent study, Flatiron researchers fine-tuned a large language model to identify a specific biomarker (PD-L1 status) from pathology reports and saw it achieve accuracy surpassing earlier rule-based methods (Flatiron Health’s AI Breakthrough: Accurate, Analyzable EHR Data Extraction-Inside Precision Medicine). This indicates that modern AI can reach human-level abstraction capabilities in parsing medical text. Such capabilities dramatically improve the accuracy and efficiency of RWE generation, as AI can process millions of clinical documents far faster than manual review, ensuring key endpoints and outcomes are not missed.
- Analyzing Real-World Outcomes and Patterns: Once real-world datasets are structured, AI techniques (like predictive modeling) help find meaningful patterns. For instance, AI can analyze longitudinal patient records to detect treatment benefit or safety signals in populations not well studied in trials. Machine learning models have been used to predict disease progression or hospital admission risk using real-world patient characteristics, aiding in outcome research. In one use case, researchers used an AI model on EHR data to predict flares in inflammatory bowel disease and identify patients likely to need intervention (Artificial Intelligence and Real-World Data: Speeding Up Drug Development Like Never Before - TriNetX) – insights that help tailor early treatment strategies. AI’s ability to sift through high-dimensional data can uncover subtle correlations (e.g. how a diabetes drug performs in patients with certain comorbidities) that traditional analytic methods might miss. This strengthens the evidence base for clinical and regulatory decisions.
- Scaling Pharmacovigilance and Safety Monitoring: Pharmaceutical companies are also applying AI to post-market surveillance data – scanning physician reports, social media, and insurance claims to detect adverse events or product issues sooner. Natural language processing can automatically classify and triage reports of drug side effects. By automating signal detection, AI tools help meet FDA’s safety monitoring requirements more efficiently, alerting manufacturers to potential risks earlier than manual review alone.
- Data Linkage and Standardization: To generate robust RWE, data often must be integrated from multiple sources (EHRs, claims, labs). AI assists in matching and linking records that belong to the same patient and mapping data to common standards. For example, AI can reconcile medication names or map diagnosis descriptions to standardized codes (like ICD or SNOMED). This data harmonization is crucial for creating large, analyzable real-world datasets. One systematic review noted that while AI greatly enhances RWE generation, data integration challenges between healthcare systems remain an issue, pointing to the need for better interoperability (Presentación de PowerPoint). AI is starting to tackle this by intelligently merging datasets and filling in gaps where possible.
Impact: The use of AI in real-world evidence is still emerging, but it is improving the accuracy and speed of evidence generation. The FDA and other stakeholders have keen interest in this – real-world evidence is increasingly used for regulatory approvals and coverage decisions, and AI can strengthen the reliability of such evidence (Presentación de PowerPoint). By automating data extraction, AI reduces human error and bias in RWE studies. It also makes previously infeasible analyses possible (e.g. sifting through millions of records for outcomes). However, as noted in studies, ensuring data quality and validating AI findings with clinical experts is vital (Presentación de PowerPoint). In the U.S., collaboration between technology firms and healthcare providers (sometimes via public-private initiatives) is accelerating RWE capabilities. For example, the federal government launched the “Stargate AI” initiative in 2025 to boost AI’s role in healthcare research (Artificial Intelligence and Real-World Data: Speeding Up Drug Development Like Never Before - TriNetX), reflecting a national interest in leveraging AI for real-world data insights.
AI for Data Standardization and Integration
Clinical data is often siloed and stored in various formats across systems. AI is helping standardize and integrate these data, which is foundational for any advanced analytics or sharing:
- Data Mapping and Harmonization: Hospitals and pharma companies face the task of converting disparate datasets into common formats (for example, mapping an EHR’s internal lab codes to standard LOINC codes, or aligning a trial’s data to the FDA’s CDISC standards). Machine learning can learn from prior mappings to suggest how new data elements should be standardized. AI tools now exist that automatically match local terminologies to universal ones with high accuracy by understanding context. This reduces a labor-intensive process. One application is using NLP to read clinical text and output structured data in FHIR (Fast Healthcare Interoperability Resources) format (A Standardized Clinical Data Harmonization Pipeline for Scalable AI ...) – effectively turning unstructured notes into standardized data points that can be exchanged or analyzed consistently.
- Master Patient Index & Record Linkage: AI-based probabilistic matching algorithms can improve how patient records are linked across systems. By analyzing names, addresses, and other identifiers (even if slightly different or containing typos), an AI can decide if two records refer to the same individual with greater accuracy than simple rules. This is crucial when merging datasets for research or coordinating care between providers. Improved patient matching reduces duplicate records and ensures longitudinal data is consolidated, which in turn makes downstream data management more reliable.
- Cleaning and De-duplication: Data standardization also involves cleaning errors and duplicates. AI can continuously scan databases to spot anomalies – for instance, two entries for the same lab test on the same day, likely an accidental duplicate. Or values that are out of normal range (possibly a unit entry error). By flagging these, AI supports data managers in maintaining high data quality. In EHR systems, studies show that AI can detect data errors or irregular patterns that humans might miss (Effective Strategies for Tackling Data Quality Issues in Healthcare), thereby preventing flawed data from propagating into analytics or patient care decisions.
- Interoperability Between Legacy Systems: Many U.S. healthcare organizations struggle with legacy software that doesn’t easily share data. AI is facilitating interoperability by acting as a smart intermediary. For example, an AI middleware can take data from an older HL7v2 interface and convert it on-the-fly into modern FHIR resources for a new system, “translating” between old and new standards. This use of AI preserves information fidelity while enabling legacy systems to participate in integrated data workflows. Given that an estimated 72% of U.S. hospitals have experienced patient data gaps because systems wouldn’t talk to each other (AI for EHR: A Transformation in the Making), these AI-driven translation and integration solutions address a very real pain point. Over time, better integration means more complete patient records and more efficient care coordination.
Impact: By automating data standardization, AI improves data usability and comparability. Clean, standardized data is a prerequisite for any large-scale analytics, AI, or sharing between stakeholders. For instance, when a clinical trial can automatically map its data to submission standards, it speeds regulatory review; when a health system’s data is uniform, population health analytics and quality reporting become easier and more accurate. AI’s ability to learn from vast examples (e.g. past mapping decisions or common error patterns) makes it an ideal tool for this domain. Early deployments indicate significant time savings – what used to take teams of data specialists weeks can sometimes be done in days with AI assistance. It also boosts regulatory compliance by ensuring data meets required formats. As one example, an AI system that standardizes and reconciles trial data ensures that audit trails and data logs are consistent and complete, which is essential for FDA 21 CFR Part 11 compliance (AI in Clinical Data Management: Coding & Reconciliation). Organizations that invest in AI for data standardization find it de-risks their data handling and yields high-quality datasets ready for analysis. The main challenge is training these AI tools on enough high-quality mappings or examples, but this barrier is rapidly diminishing as the industry accumulates experience.
AI in Predictive Analytics and Clinical Decision Support
AI’s pattern-recognition prowess makes it a powerful engine for predictive analytics on clinical data. In the U.S., predictive models are being deployed to support both front-line care decisions and high-level planning:
- Patient Risk Prediction: Health systems use AI models to predict which patients are at risk for outcomes like readmission, emergency deterioration, or treatment complications. These models analyze historical EHR data to find patterns (e.g. vital sign trends, lab results, comorbidities) that precede an event. For example, hospitals have implemented AI predictors for early sepsis detection, ICU transfer risk, or likely 30-day readmission. When effective, such models let clinicians intervene proactively – one case study reported a modest but meaningful 2.1% reduction in hospital readmission rates after deploying AI-based risk scoring (AI for EHR: A Transformation in the Making). Similarly, machine learning–based clinical decision support for inpatient care has been associated with shorter average lengths of stay and fewer adverse events (AI for EHR: A Transformation in the Making).
- Population Health and Resource Planning: At a broader level, predictive analytics help health administrators manage patient populations and resources. AI can forecast surges in hospital admissions (useful for staffing and bed management) or identify which chronic disease patients in a health plan are likely to incur high costs, enabling targeted preventive care. In the pharmaceutical domain, companies use AI to predict how a drug will perform in a real-world population or to identify which subgroups benefit most, thereby informing post-market strategies.
- Clinical Decision Support Systems (CDSS): Beyond risk scores, AI is being embedded in clinical decision support tools that guide diagnosis and treatment. For instance, some emergency departments use AI to analyze EHR data and suggest potential diagnoses or flag overlooked abnormal results to physicians. There are FDA-cleared AI systems for imaging and pathology that integrate with clinical workflows – e.g. an AI that reads radiology images for signs of cancer and reports findings to the radiologist. While imaging AI is a bit tangential to “data management,” it ultimately contributes to the clinical data by adding structured findings to the patient record. Diagnostic assistance AI can reduce diagnostic errors and ensure more consistent care by providing a second look or recommendation (The role of artificial intelligence for the application of integrating ...).
- Real-Time Clinical Analytics: With advancements in streaming data (like continuous vital monitors), AI can analyze data in real-time to predict events like cardiac arrest or sepsis hours in advance. These streaming analytics, often powered by deep learning, represent the cutting edge of predictive medicine. A well-known example is the Epic Sepsis Model described earlier, as well as proprietary models developed by institutions like Mayo Clinic or Kaiser Permanente for in-hospital deterioration. The benefit is earlier warning and triage. The drawback, as seen, is potential false alarms or bias if models are not perfectly calibrated to the patient population (Epic's widely used sepsis prediction model falls short among Michigan Medicine patients-Fierce Healthcare). Addressing these issues is an active area of improvement.
Impact: When implemented thoughtfully, predictive analytics improve clinical outcomes and operational efficiency. Patients receive more personalized, proactive care, and providers can allocate resources more effectively. For example, knowing which discharged patients are at high risk of readmission allows a hospital to arrange follow-up calls or home visits, preventing costly readmissions. Predictive models have shown value in specific areas like reducing sepsis mortality by prompting earlier antibiotics administration. In one large healthcare system, adopting a suite of AI-driven prediction tools was associated with a drop in complication rates and a net savings by avoiding unnecessary ICU days (AI for EHR: A Transformation in the Making). However, these benefits depend on model accuracy and clinician trust. A key challenge has been algorithmic bias – if a model is trained on data that underrepresents certain groups, its predictions may be less accurate for those patients. A notorious 2019 study revealed that a widely used commercial risk algorithm systematically underestimated risk for Black patients, resulting in Black patients having 26% more chronic illnesses on average at the same algorithmic risk score as white patients (Racial Bias Found in a Major Health Care Risk Algorithm-Scientific American). Such biases led to fewer Black patients being flagged for high-risk care management (Racial Bias Found in a Major Health Care Risk Algorithm-Scientific American). This example underscores that predictive analytics must be continuously monitored and validated to ensure equity and safety. U.S. institutions are now implementing checks (like bias assessments and periodic re-training with diverse data) as part of their AI governance, as discussed below.
The table below summarizes key AI applications in clinical data management and their impacts:
Application Area | Example AI Uses | Notable Impact (U.S. examples) |
---|---|---|
Electronic Health Records (EHR) | NLP transcription of clinical notes; AI-assisted coding; Voice assistants for documentation; EHR-integrated decision support (risk alerts) | Efficiency: Physicians save significant time – e.g. 72% less time on documentation at Rush with an AI scribe (RUSH Deepens its AI Efforts By Teaming Up with Suki to Tackle Clinician Burnout - Suki AI). Accuracy: More consistent coding and note quality (AI coding 96% accurate vs. human benchmark (AI in Clinical Data Management: Coding & Reconciliation)). Adoption: ~66% of U.S. doctors now use some AI for EHR tasks (documentation, order entry, etc.) (2 in 3 physicians are using health AI—up 78% from 2023-American Medical Association). |
Clinical Trials Data | AI site selection and patient matching; Automated data cleaning & query resolution; AI-generated clinical reports (CSR drafting); Predictive trial monitoring | Speed: AI optimizes operations, cutting trial durations – site AI improved enrollment ~15% and shaved ~6 months off development timelines (Performance in clinical development with AI & ML-McKinsey) (Performance in clinical development with AI & ML-McKinsey). Efficiency: Auto-coding and cleaning tools save hundreds of hours (e.g. 69+ hours per 1,000 data points coded) (AI in Clinical Data Management: Coding & Reconciliation). Quality: AI document drafting achieves ~98% accuracy (Performance in clinical development with AI & ML-McKinsey), reducing errors in regulatory submissions. |
Real-World Evidence (RWE) | NLP extraction from EHR/free text; ML analysis of large observational datasets; AI for pharmacovigilance signal detection | Insights: AI enables use of real-world patient data at scale – patterns and treatment outcomes identified that inform care beyond clinical trials (Presentación de PowerPoint). Accuracy: Improved data extraction leads to more reliable RWE; AI systems can match or exceed human abstraction accuracy in parsing records (Flatiron Health’s AI Breakthrough: Accurate, Analyzable EHR Data Extraction-Inside Precision Medicine). Regulatory: Strong RWE (powered by AI) is supporting FDA decisions for drug safety and new indications, although data integration issues remain a challenge (Presentación de PowerPoint). |
Data Standardization | ML mapping of codes and terminologies; Intelligent data integration across systems; Automated de-identification of PHI for compliance | Interoperability: AI reduces data silos – e.g. aligning 72% of hospitals’ disparate data to common standards to close information gaps (AI for EHR: A Transformation in the Making). Productivity: Tasks like medical term coding are done in seconds by AI (versus minutes by humans), greatly accelerating data preparation (AI in Clinical Data Management: Coding & Reconciliation). Compliance: AI-driven de-identification tools (like AWS Comprehend Medical) can automatically redact HIPAA identifiers (Amazon Comprehend Medical - Healthcare NLP - AWS), enabling safer data sharing for research. |
Predictive Analytics | Predictive models for patient risk (sepsis, readmission, etc.); Population health predictions; AI-driven clinical decision support alerts | Patient Outcomes: Early warning models can improve outcomes (e.g. lower sepsis mortality with timely alerts), and some hospitals saw measurable reductions in length of stay and readmissions (AI for EHR: A Transformation in the Making). Efficiency: Better resource allocation (predicting ICU needs, etc.) saves costs. Caution: Bias and false alarms are challenges – e.g. one widely used sepsis model missed ~67% of true sepsis cases (Epic's widely used sepsis prediction model falls short among Michigan Medicine patients-Fierce Healthcare), highlighting the need for ongoing model oversight. |
Benefits of AI in Clinical Data Management
The adoption of AI in managing clinical data is yielding numerous benefits for healthcare and pharma organizations in the U.S. Key advantages include:
- Improved Efficiency and Productivity: AI automates labor-intensive tasks – from data entry to report generation – which saves time and operational costs. This efficiency is evident in both clinical practice and research settings. For instance, by using AI documentation tools, physicians can reduce documentation time by 50–70%, freeing them to see more patients or focus on care (RUSH Deepens its AI Efforts By Teaming Up with Suki to Tackle Clinician Burnout - Suki AI) (A year of DAX Copilot: Healthcare innovation that refocuses on the clinician-patient connection - The Official Microsoft Blog). In clinical trials, AI automation of data cleaning and monitoring allows studies to run faster with leaner teams, potentially shortening development timelines (a 2025 industry survey found 53% of trial leaders believe AI/ML will have the greatest impact on improving efficiency and outcomes in the near term (New Research Reveals Status of AI/ML Adoption Amid Surging ...)). Ultimately, AI-driven efficiency can lower administrative costs in healthcare – Deloitte has projected billions in savings industry-wide as AI scales routine workflows.
- Higher Data Quality and Accuracy: AI systems excel at detecting patterns and anomalies, which helps catch errors in clinical data that humans might overlook. They enforce consistency in how data is recorded. For example, NLP-based transcription greatly reduces documentation errors compared to hurried manual typing (Enhancing Clinical Documentation with AI: Reducing Errors ...), and AI coding tools ensure standardized terminology usage. In trials, automated edit checks by AI mean cleaner datasets (fewer missing values, implausible entries, etc.). Some organizations report significant improvements in data completeness after implementing AI-assisted data capture (AI in Clinical Data Management: Coding & Reconciliation). Moreover, AI’s ability to aggregate data across sources provides a more comprehensive and accurate view of patient information (no more isolated data silos). This improved data fidelity supports better clinical decisions and analyses.
- Enhanced Regulatory Compliance: AI is helping organizations remain compliant with complex healthcare regulations. In the U.S., HIPAA requires strict protection of patient privacy – AI tools can automatically identify and redact protected health information (PHI) from documents, aiding de-identification efforts so data can be used in research without breaching privacy (Amazon Comprehend Medical - Healthcare NLP - AWS). AI can also continuously monitor access logs and user behavior in data systems to flag suspicious activity, strengthening security compliance. When it comes to FDA regulations, AI assists with 21 CFR Part 11 compliance by maintaining thorough, searchable audit trails and ensuring data integrity in electronic records (AI in Clinical Data Management: Coding & Reconciliation). Some AI platforms are designed with audit logs and traceability to satisfy inspectors that processes are validated and reliable. Additionally, AI can help with quality control and reporting requirements – for example, automatically compiling clinical quality measures or pharmacovigilance reports within required timelines. By standardizing and checking data against expected ranges, AI reduces the risk of non-compliance due to data errors. In sum, AI acts as a tireless assistant that can enforce rules and spot issues, which is invaluable for meeting U.S. healthcare’s stringent regulatory and quality standards.
- Faster Insights and Decision-Making: With AI handling data processing at scale, healthcare organizations can derive insights much more quickly. What used to take analysts weeks to crunch (if at all possible) might be done in hours by AI. This accelerates research discoveries (e.g. identifying a drug’s off-label benefits via RWE analysis) and clinical decisions (e.g. an AI early warning saves a critical hour in starting sepsis treatment). Quicker turnaround from data to action can improve patient outcomes and give companies a competitive edge in drug development.
- Strategic Advantages: For pharmaceutical companies, leveraging AI in data management contributes to shorter development cycles, potentially bringing therapies to market faster and extending patent-effective sales time. For provider organizations, AI can improve patient satisfaction and outcomes, which are tied to reimbursement (value-based care models reward lower readmissions, better preventive care, etc., all of which AI can facilitate). Moreover, embracing AI can position organizations as innovative leaders, attracting partnerships and talent. Many U.S. hospitals now highlight their AI initiatives (e.g. Mayo Clinic’s AI programs) as a mark of prestige and forward-thinking care.
In summary, AI offers tangible improvements in how clinical data is handled – making processes more efficient (saving time/cost), data more accurate (reducing errors), and operations more compliant and intelligence-driven. These benefits ultimately translate into better patient care and scientific innovation. As one health IT expert observed, AI in healthcare “can decrease medical errors and reduce clerical burdens for physicians” when properly implemented (Learning from the EHR to implement AI in healthcare - Nature), aligning technology with the prime directive of improving care.
Challenges and Risks of AI in Clinical Data Management
Despite its promise, the integration of AI into clinical data workflows comes with significant challenges and risks that organizations must address:
- Data Privacy and Security Concerns: Clinical data is highly sensitive, and using AI often requires aggregating large datasets (potentially including patient identifiers) or relying on cloud-based services. This raises concerns about HIPAA compliance and patient privacy. If AI models are trained on identifiable data, there is a risk (however small) of re-identification or data leakage. Any breach or improper handling can lead to severe legal penalties and loss of trust. For example, using an AI chatbot on real patient data without proper safeguards could inadvertently expose PHI. Ensuring robust encryption, access controls, and that AI vendors sign Business Associate Agreements (making them accountable under HIPAA) is essential (A Roadmap for Thinking about Health Data, AI and HIPAA Applicability). Additionally, cybersecurity of AI systems is critical – attackers might target AI pipelines (poisoning the training data or model) to exfiltrate information or cause harm. The U.S. Department of Health and Human Services (HHS) has been directed to regulate AI’s impact on healthcare data privacy and security (AI explained: AI and recent HHS activity with HIPAA considerations-Perspectives-Reed Smith LLP), highlighting that this is a top-tier concern. In practice, organizations often mitigate this by de-identifying data before AI processing and thoroughly vetting any third-party AI tools for security compliance.
- Algorithmic Bias and Fairness: AI models can inadvertently perpetuate or even amplify biases present in historical data. In healthcare, this can translate to disparities in care. A notable real-world example was the algorithm that under-referred Black patients to high-risk care programs because it used healthcare cost as a proxy for need – since Black patients historically had lower expenditures (due to access issues), the algorithm concluded they were “healthier” than they truly were (Racial Bias Found in a Major Health Care Risk Algorithm-Scientific American). This kind of bias led to unequal care recommendations until it was uncovered. Similarly, some AI-driven diagnostic tools have shown differing accuracy by race or gender if those groups were underrepresented in the training data. Bias in AI is a serious risk because it can systematically disadvantage certain populations, exacerbating health disparities (AI in medicine need to counter bias, and not entrench it more - NPR). It can also erode clinician trust in the tools. Addressing this requires careful dataset curation (ensuring diversity), bias testing of models, and possibly algorithmic adjustments to correct identified biases (The role of health care algorithms in racial bias, inequity). U.S. regulators are aware – the White House’s 2023 AI Bill of Rights blueprint emphasizes algorithmic fairness in healthcare, and HHS is investigating whether AI contributes to medical errors or inequities (AI explained: AI and recent HHS activity with HIPAA considerations-Perspectives-Reed Smith LLP). Ensuring AI outputs are not discriminatory is both an ethical mandate and increasingly a regulatory expectation.
- Integration with Legacy Systems: Many healthcare organizations operate on legacy IT systems and fragmented data silos. Integrating cutting-edge AI solutions into these environments can be very challenging. AI tools often require large volumes of accessible, high-quality data, but legacy systems might not easily supply that (due to outdated data formats or lack of interoperability). Additionally, real-time AI support (like an alert system) demands interfaces with EHRs or devices that older systems weren’t designed to provide. The result can be slow or costly integration projects. In some cases, hospitals have AI pilots that work in concept but struggle to implement system-wide due to infrastructure constraints. Moreover, clinicians already complain about EHR usability – adding AI on top should ideally simplify their workflow, not complicate it. If integration is clumsy (e.g. requiring using a separate application or producing an overload of alerts), users may resist adoption. Basically, legacy tech debt and siloed data can limit the seamless deployment of AI, requiring investment in data engineering and interoperability. On the positive side, standards like FHIR are easing integration, and AI middleware can bridge some old-new gaps, but this remains a practical hurdle for many.
- Transparency and Validation: Many AI models (especially deep learning ones) are “black boxes” that don’t explain their reasoning. In healthcare, this opacity can be problematic – clinicians and regulators want to know why a model made a certain prediction (e.g. why did the AI flag this patient as high-risk?). Lack of transparency can hinder trust. If an AI system cannot provide understandable rationale, providers might be hesitant to act on its recommendations, particularly for high-stakes decisions. Furthermore, thorough validation of AI tools in clinical settings is necessary to ensure they actually work as intended on the target population. As seen with Epic’s sepsis model, vendor-provided performance claims may not hold in independent real-world testing (Epic's widely used sepsis prediction model falls short among Michigan Medicine patients-Fierce Healthcare) (Epic's widely used sepsis prediction model falls short among Michigan Medicine patients-Fierce Healthcare). This “proof gap” is risky – deploying unvalidated or overly hyped AI could lead to errors or patient harm. Organizations need processes for pilot testing AI on their own data and continuously monitoring performance metrics (sensitivity, specificity, error rates, etc.). The FDA’s new draft guidance explicitly asks for demonstrating “credibility” of AI models for their context of use (FDA Issues Draft Guidance on the Role of Artificial Intelligence in Supporting Regulatory Decisions), reflecting the importance of validation.
- Workforce and Workflow Adaptation: Introducing AI requires change management. Clinicians and data staff must be trained to use these tools and interpret their outputs. There can be resistance due to fear of technology replacing jobs or simply added complexity. If workflows aren’t redesigned to integrate AI smoothly, the promised efficiency gains might not materialize. For example, if a doctor has to click through an extra AI interface during a patient visit, it might annoy them rather than help. Human factors design is crucial so that AI augments rather than obstructs. There’s also the issue of who is liable if AI makes a mistake – this is legally uncharted territory. Providers might fear that relying on AI could open them to liability, so some default to “ignore the AI and do things the old way,” negating its benefit. Building confidence through training, pilot success stories, and having clear policies (e.g. AI suggests, but clinician always has final judgment) can mitigate these issues.
- Cost and ROI Considerations: While AI can save money in the long run, the upfront investment in technology, talent (data scientists, AI engineers), and process overhaul can be substantial. Healthcare budgets are often tight, and making the case for AI projects requires demonstrating return on investment. Not all AI implementations yield immediate financial returns – some are strategic or quality-focused. Smaller clinics or organizations might lack the resources to implement AI, widening the gap between large, well-funded systems and others. However, with more off-the-shelf AI services becoming available (from cloud vendors, etc.), the cost barrier is slowly lowering.
Addressing these challenges is critical to realizing AI’s full potential in clinical data management. Encouragingly, awareness of these issues is high in 2025: professional societies, regulators, and industry groups are actively publishing guidelines for “Responsible AI” in healthcare to ensure safety, fairness, and efficacy. For example, the National Minority Health Council has issued principles to address bias in healthcare algorithms (Shedding Light on Healthcare Algorithmic and Artificial Intelligence ...), and the FDA is working on a risk-based framework for AI validation (FDA Issues Draft Guidance on the Role of Artificial Intelligence in Supporting Regulatory Decisions). Healthcare CIOs and CMIOs now often include AI governance committees in their organizations to oversee these aspects. In summary, while AI brings powerful tools, vigilance and governance are needed to mitigate risks and ensure that AI in healthcare augments care without unintended harm.
Regulatory and Ethical Considerations in the U.S.
The U.S. regulatory and ethical landscape for AI in clinical data management is rapidly evolving to keep pace with technology. Stakeholders must navigate existing healthcare regulations (like FDA laws and HIPAA privacy rules) as well as emerging AI-specific guidance:
- FDA Guidance on AI/ML in Healthcare: The Food and Drug Administration (FDA) regulates many products of AI, especially when they are used for diagnosis or treatment (which can qualify them as medical devices). For AI used in clinical data management and drug development, the FDA has begun issuing guidance. In January 2025, the FDA released its first draft guidance on the use of AI to support regulatory decision-making for drugs and biologics (FDA Issues Draft Guidance on the Role of Artificial Intelligence in Supporting Regulatory Decisions). This guidance outlines a risk-based framework for sponsors to establish the “credibility” of AI models they use in submissions (FDA Issues Draft Guidance on the Role of Artificial Intelligence in Supporting Regulatory Decisions). In practice, that means if a pharma company uses an AI analysis as evidence (say an AI that analyzed EHR data to conclude a drug is effective in some subpopulation), they need to demonstrate the model’s reliability, explainability, and validation to the FDA. Commissioner Robert Califf emphasized that the FDA supports AI innovation but wants to ensure “robust scientific and regulatory standards are met” (FDA Issues Draft Guidance on the Role of Artificial Intelligence in Supporting Regulatory Decisions). This implies rigorous validation and transparency will be expected. On the medical device side, the FDA’s Digital Health Center has already been evaluating AI-based software (like imaging analysis tools) and in 2023 finalized guidance on managing AI algorithms that can evolve (the “Predetermined Change Control Plan” for learning algorithms) (FDA Finalizes Guidance for AI/ML Medical Devices - Exponent). For AI in EHRs and hospital workflows (which may not be regulated devices), the FDA generally doesn’t intervene unless the AI is making clinical diagnoses or treatment recommendations. However, if an AI tool is marketed with claims of improving clinical outcomes, it might trigger oversight. Overall, FDA is signaling a collaborative stance: enabling AI’s benefits (faster drug development, personalized medicine) while ensuring patient safety and efficacy are not compromised. Organizations deploying AI should stay abreast of FDA guidances and be prepared to provide documentation on algorithm performance, data provenance, and any risk mitigation for bias or errors.
- HIPAA and Data Privacy Laws: HIPAA (Health Insurance Portability and Accountability Act) remains a cornerstone of U.S. health data privacy. Any AI system dealing with identifiable patient data must comply with HIPAA’s privacy and security rules. This means covered entities (providers, payers) must only use patient data for permitted uses (treatment, payment, operations) or research with proper authorization/de-identification. If an AI vendor (e.g. a cloud AI service) is processing PHI on behalf of a hospital, a Business Associate Agreement is required, binding the vendor to HIPAA rules (A Roadmap for Thinking about Health Data, AI and HIPAA Applicability). One ethical consideration is patient consent – should patients be informed or consent to their data being used to train AI? Currently, if it’s for healthcare operations (e.g. improving data management processes within the covered entity), HIPAA permits it without individual consent. But for secondary use, like developing a general AI product, consent or at least de-identification is needed. Regulators are also wary of new risks: AI models sometimes can memorize aspects of their training data, so there’s ongoing research on preventing AI from regurgitating sensitive info. HHS has been tasked (via a 2023 Executive Order) with evaluating whether AI use is consistent with privacy and security standards (AI explained: AI and recent HHS activity with HIPAA considerations-Perspectives-Reed Smith LLP). Another law, the 21st Century Cures Act, promotes data interoperability but also safeguards patient access and control over their records, which intersects with AI that may use broad data sharing. Additionally, state laws like California’s Consumer Privacy Act (CCPA) might come into play if AI involves consumer health apps outside of HIPAA’s umbrella. Ethically, “data stewardship” is crucial – organizations should minimize data used, anonymize when possible, and be transparent. For example, if a hospital uses AI to analyze patient data for research, an ethical approach is to inform patients through notices and give opt-out options if feasible. In summary, compliance with privacy regulations is non-negotiable: AI projects must bake in data security, access controls, and privacy-by-design (like using synthetic data for development when possible).
- Ethical Use and Accountability: Beyond formal regulations, there are broad ethical principles being emphasized for AI in healthcare. The AMA (American Medical Association) has published guidelines for augmented intelligence, stressing that AI should enhance patient care, be evidence-based, and not override clinician judgment ([PDF] Future of Health: The Emerging Landscape of Augmented | AMA). Ensuring transparency (to the extent possible) and the ability to explain AI-driven decisions is considered ethical best practice – especially if an AI influences a life-or-death decision, the patient deserves to know the rationale in understandable terms. There is also the principle of accountability: who is responsible if AI causes harm? Legally, today it would likely be the provider or manufacturer, but this is a gray area. Ethically, organizations shouldn’t use AI as a scapegoat; they must take responsibility for outcomes and have humans in the loop for critical decisions. Informed consent might evolve to cover AI – e.g. should trial participants be told that AI will be used to monitor their data or adjudicate an endpoint? These questions are under discussion. The FDA’s stance on transparency for AI/ML-enabled devices (from an April 2021 action plan) also encourages that users are made aware that a given tool is AI-driven and of its known limitations. Another ethical consideration is bias mitigation (touched above) – healthcare AI should be designed and monitored to promote equity. The Office of Minority Health at HHS has launched initiatives to study and reduce algorithmic bias (Shedding Light on Healthcare Algorithmic and Artificial Intelligence ...), reflecting an ethical commitment to “do no harm” across all patient groups.
- Emerging Oversight Mechanisms: In Washington, there is increasing attention on AI oversight in healthcare. Apart from FDA’s efforts, we see bodies like the FTC (Federal Trade Commission) warning against misleading claims for AI health products (truth-in-advertising applies – an AI can’t be marketed as “98% accurate” without evidence). Congress has held hearings on AI in health, though no sector-specific AI law exists yet. However, the emphasis is that existing laws (HIPAA, FDA’s FD&C Act, product liability, anti-discrimination laws like the Civil Rights Act) all still apply to AI. If an AI tool leads to discriminatory practices, it could violate civil rights law. If an AI component of an EHR malfunctions and causes an adverse event, it could fall under healthcare malpractice or product liability. We may see more formal requirements soon: for example, the FDA might require algorithm developers to implement Good Machine Learning Practice (GMLP) in development, similar to how pharma follows Good Clinical Practice. Professional accreditation bodies might also include AI competency – e.g. clinical trial auditors might start asking how AI outputs were verified.
- Human Oversight and the “Human-in-the-Loop” Requirement: Ethically and per draft guidance, AI in clinical scenarios should have appropriate human oversight. The FDA has hinted that even as automation increases, a qualified professional should remain the final decision-maker for patient care decisions. In clinical trials, sponsors using AI for data management are advised to keep data managers/statisticians involved to review AI findings. Completely “hands-off” automation in regulated processes is risky; agencies will ask how humans oversee or validate the AI’s work. For instance, an AI that auto-adjudicates data mismatches in a trial should log its actions and allow human review of a sample to ensure it’s doing it correctly. This human-in-the-loop approach is seen as the safe path forward, and likely to be encoded in guidance.
In summary, the U.S. environment is supportive but cautious: regulators encourage leveraging AI’s benefits for efficiency and insight, yet they are simultaneously crafting frameworks to ensure this is done safely, ethically, and in compliance with longstanding health protections. Organizations using AI for clinical data must stay informed of these evolving guidelines. By following best practices (transparency, validation, bias mitigation, privacy protection) and documenting their AI processes, they can not only satisfy regulators but also build trust with clinicians and patients. As Dr. Robert Califf of FDA noted, with appropriate safeguards, AI can truly accelerate research and improve care (FDA Issues Draft Guidance on the Role of Artificial Intelligence in Supporting Regulatory Decisions) – the task at hand is putting those safeguards firmly in place.
Leading AI Tools and Vendors in Clinical Data Management
The rapid growth of AI in healthcare has given rise to a variety of tools and vendors catering to different aspects of clinical data management. Below is an overview of some leading AI tools and vendors in the U.S. market, and how they are being used:
- Nuance DAX (Microsoft): Nuance Dragon Ambient eXperience (DAX) is an AI-powered ambient clinical documentation tool. It automatically transcribes and summarizes patient visits through voice conversation capture. Now part of Microsoft (acquired in 2022), Nuance DAX is widely integrated with EHRs like Epic. Over 400 healthcare organizations in the U.S. have deployed DAX in the past year (A year of DAX Copilot: Healthcare innovation that refocuses on the clinician-patient connection - The Official Microsoft Blog). Clinicians report that it cuts documentation time ~50% per encounter, on average saving 7 minutes of charting for every patient visit (Nuance DAX: How AI Can Transform Your Clinical Documentation). By reducing clerical work, DAX aims to combat physician burnout and improve patient eye-contact time. Leading academic centers (e.g. Northwestern Medicine, Atrium Health) have documented increased physician satisfaction and improved note quality with DAX (A year of DAX Copilot: Healthcare innovation that refocuses on the clinician-patient connection - The Official Microsoft Blog) (A year of DAX Copilot: Healthcare innovation that refocuses on the clinician-patient connection - The Official Microsoft Blog). This tool represents the state-of-the-art in ambient documentation AI.
- Suki AI Assistant: Suki is another popular voice AI for clinical documentation, often used in ambulatory settings. It functions like a digital medical scribe, allowing physicians to dictate notes in a conversational manner. Suki integrates with EHRs and can handle commands (e.g. “pull in last lab results”). At Rush University Health, Suki’s deployment led to the 72% reduction in documentation time mentioned earlier (RUSH Deepens its AI Efforts By Teaming Up with Suki to Tackle Clinician Burnout - Suki AI). Users also saw fewer coding errors and faster chart completion. Suki and Nuance are somewhat competitors in this “AI scribe” space, both targeting the U.S. market where documentation burden is high.
- Epic Cognitive Computing (Epic Systems): Epic, the largest EHR vendor in the U.S., has been embedding AI features directly into its platform. The Epic Sepsis Model is one example of a predictive algorithm available to Epic clients, used in hundreds of hospitals (Epic's widely used sepsis prediction model falls short among Michigan Medicine patients-Fierce Healthcare). Epic also offers predictive models for patient deterioration, no-show appointments, and other use cases, trained on its massive de-identified data set (Cosmos). Additionally, Epic has incorporated NLP for chart review (e.g. an “NoteReader” that can suggest missing diagnoses by reading notes). While Epic’s AI tools have broad reach due to the company’s market share, their performance varies and hospitals often supplement with third-party AI or their own models. Still, Epic is a key “vendor” to mention because its built-in AI capabilities influence how many U.S. health systems experience clinical AI (often as part of their core EHR).
- Medidata (Dassault Systèmes): Medidata is a dominant vendor in clinical trial data management (providing EDC systems used in many trials). They have introduced AI features in their Rave platform. For instance, Medidata Rave Coder uses AI to auto-suggest standard codes for adverse events and medications. As noted, it achieves ~96% accuracy for high-confidence suggestions (AI in Clinical Data Management: Coding & Reconciliation), greatly accelerating the coding process. Medidata also developed an AI-based data reconciliation engine and is piloting generative AI for trial audit trail analysis (AI in Clinical Data Management: Coding & Reconciliation) (AI in Clinical Data Management: Coding & Reconciliation). Many pharma companies and contract research organizations are adopting these tools to streamline trial workflows. Medidata’s competitors (like Oracle Health Sciences’ InForm and IQVIA’s platforms) are also adding AI, but Medidata is seen as an early mover with concrete AI products in use.
- Flatiron Health: Flatiron is known for its real-world oncology data platform. It employs a hybrid human+AI approach to curate oncology EHR data. Their internally developed NLP and machine learning tools assist human abstractors by highlighting relevant info in charts. Recently, Flatiron explored LLMs to automate data extraction and found promising results in capturing complex oncology data points with high accuracy (Flatiron Health’s AI Breakthrough: Accurate, Analyzable EHR Data Extraction-Inside Precision Medicine). Flatiron’s datasets (used by academia, FDA, and pharma) are a prime example of AI enabling RWE – they claim some data processing tasks that once took weeks can be done in hours with AI assistance. Flatiron (acquired by Roche) also partners with others on AI in clinical decision support for oncology.
- Tempus: Another notable player, Tempus, focuses on AI and precision medicine. They generate genomic sequencing data and integrate it with clinical data to help guide oncology treatment. Tempus uses AI models to identify which cancer patients might benefit from certain therapies or trials, analyzing both molecular and clinical variables. They also have an AI-driven diagnostic tests (like for AI pathology). In data management, Tempus curates a vast library of cancer patient data that can serve as RWE for drug development or comparative effectiveness research.
- IQVIA: IQVIA (a major CRO and health data analytics company) has an “Orchestrated Clinical Trials” platform that leverages AI for trial planning and execution. For example, IQVIA’s algorithms predict optimal trial sites globally and identify patients from their network of EHR and claims data. IQVIA reports that its AI-driven approach can boost enrollment and even simulate control arms with RWD, potentially reducing the need for placebo arms in some studies. They also offer AI tools for pharmacovigilance (finding safety signals in real-world data) and for adherence programs (identifying patients likely to drop off therapy). As a vendor, IQVIA often partners with pharma to embed these AI solutions in development programs.
- Google Cloud & AWS (Healthcare AI Services): The big cloud providers offer toolkits that many health IT teams use to build AI solutions. Google Cloud’s Healthcare API and AutoML provide NLP that can extract medical concepts from text, identify medication names, procedures, and more. Google’s tools have been used by groups like Mayo Clinic to annotate years of clinical notes and improve their searchability. Amazon Web Services (AWS) offers Comprehend Medical, an NLP service that is HIPAA-eligible and can detect PHI and medical terms in text (Amazon Comprehend Medical - Healthcare NLP - AWS). Hospitals have used it to de-identify notes at scale or pull structured data for analysis. AWS also has AI services for medical image analysis and Amazon HealthLake for aggregating health data. Microsoft’s Azure likewise has a suite of healthcare ML tools (and given it owns Nuance, it’s heavily invested in this space). These cloud AI services are not end-user applications but they empower healthcare organizations and startups to create AI-enhanced data management pipelines without building models from scratch.
- Emerging Startups and Tools: There are numerous startups targeting niches: e.g. H2O.ai provides an AI platform some hospitals use for developing custom models on their data; Truveta is a health system-led data platform using AI to harmonize EHR data from multiple providers for research; Jvion offers an “AI clinical success platform” with pre-built predictive models for various use cases (like identifying high-risk patients for specific conditions). In clinical trials, startups like Saama Technologies and eClinical Solutions have AI-driven trial data platforms (with eClinical’s “Elluminate” platform emphasizing AI in data cleaning). Even consulting firms (Deloitte, Accenture) have developed AI analytics solutions they implement for clients (such as Deloitte’s Cortex AI for RWE). The ecosystem is vibrant, and many tools are being refined through real-world deployments.
The following table provides a summary of selected AI tools/vendors, their focus areas, and reported impacts in U.S. clinical data management:
AI Tool / Vendor | Focus Area | Reported Impact / Use in U.S. |
---|---|---|
Nuance DAX (Microsoft) | Ambient clinical documentation (EHR) | Used by 400+ U.S. health orgs; reduces physician documentation time ~50%, enabling 5–11 more patients/week per doctor (A year of DAX Copilot: Healthcare innovation that refocuses on the clinician-patient connection - The Official Microsoft Blog). 75% of doctors felt it decreased burnout ([PDF] Improving financial performance with the Nuance Dragon Ambient ...). |
Suki AI Assistant | Voice-based EHR documentation | Deployed in large health systems (e.g. Rush); achieved 72% less time on notes and 48% fewer chart corrections (RUSH Deepens its AI Efforts By Teaming Up with Suki to Tackle Clinician Burnout - Suki AI) (RUSH Deepens its AI Efforts By Teaming Up with Suki to Tackle Clinician Burnout - Suki AI). Integrates with Epic and Cerner for seamless workflow. |
Epic Systems (Cognitive Computing) | EHR-integrated AI (predictive models, NLP) | Epic’s sepsis model in hundreds of hospitals (Epic's widely used sepsis prediction model falls short among Michigan Medicine patients-Fierce Healthcare) (improves early sepsis identification, though needs tuning). Epic’s tools for predicting no-shows and readmissions are widely used in ambulatory settings to allocate resources. |
Medidata (Rave AI) | Clinical trial data platform | AI auto-coding (96% accuracy) and reconciliation in use by top pharma, saving 60+ hours per 1,000 data points coded (AI in Clinical Data Management: Coding & Reconciliation). Piloting generative AI for instant audit trail summaries to speed up regulatory responses. |
Flatiron Health | Real-world oncology data (RWE) | Uses ML/NLP to abstract EHR data from 280+ clinics, creating research-grade datasets. Demonstrated LLMs can accurately extract complex oncology data, improving throughput of RWE generation (Flatiron Health’s AI Breakthrough: Accurate, Analyzable EHR Data Extraction-Inside Precision Medicine). Data used in FDA submissions and comparative studies. |
IQVIA | Trial planning & RWD analytics | AI-driven trial site selection and patient finding (claimed 10–20% faster enrollment) (Performance in clinical development with AI & ML-McKinsey). Offers safety signal detection AI scanning millions of records (used in post-market surveillance by pharma). Provides custom AI solutions to life sciences companies. |
Google Cloud Healthcare AI | NLP and data interoperability services | NLP API that hospitals use to structure text data (e.g. extracting problem lists from notes). Mayo Clinic used Google AI to index 10+ years of records for research queries. Google’s tools also help map data to FHIR standard, easing interoperability (Artificial Intelligence (AI) Focus Team - What is the optimum role for ...). |
AWS Comprehend Medical | NLP for text & PHI de-identification | HIPAA-compliant service; used by insurers and providers to auto-extract medical conditions, medications, and redact identifiers (Amazon Comprehend Medical - Healthcare NLP - AWS). For example, Humana used it to process millions of call transcripts to identify social determinants of health notes. |
Truveta | Aggregated EHR data platform (multi-system) | Uses AI to normalize and de-identify data from 20+ health systems, creating a unified dataset of tens of millions of patients. Aids public health and pharma research by providing harmonized real-world data (e.g. COVID-19 outcomes research). Relies on ML for data cleaning across sources. |
(Sources: vendor case studies and reports; e.g. Microsoft Blog on DAX (A year of DAX Copilot: Healthcare innovation that refocuses on the clinician-patient connection - The Official Microsoft Blog), Suki press release (RUSH Deepens its AI Efforts By Teaming Up with Suki to Tackle Clinician Burnout - Suki AI), McKinsey (Performance in clinical development with AI & ML-McKinsey), Medidata white paper (AI in Clinical Data Management: Coding & Reconciliation), FDA statements (FDA Issues Draft Guidance on the Role of Artificial Intelligence in Supporting Regulatory Decisions).)
Each of these tools addresses specific needs, and often they are used in combination. For instance, a hospital might use Nuance DAX for documentation, Epic’s built-in model for sepsis alerts, and a custom model on Google Cloud for predicting which patients will miss appointments. Or a pharma company might use Medidata for trial data capture and Flatiron for real-world oncology data analysis. Interoperability between AI tools is improving – many vendors provide open APIs or integration partnerships (for example, Nuance and Epic collaborate to embed DAX, and Medidata and Flatiron have data linkages for hybrid trial/RWE studies).
It’s also worth noting the distinction between general AI platforms and healthcare-specific vendors. The big tech companies (Microsoft, Google, Amazon, IBM) provide powerful AI infrastructure and some healthcare solutions (IBM’s Watson Health, now Merative, attempted broad healthcare AI including clinical decision support, though with mixed success). Meanwhile, specialized vendors deeply understand clinical workflows and data standards, which often leads to better user adoption in practice. Thus, collaborations are common – e.g. Microsoft provides cloud and AI backbone while Epic/Nuance provide domain-specific interfaces.
As of 2025, the AI in healthcare market is booming – estimated to reach $188 billion globally by 2030 (AI for EHR: A Transformation in the Making), with the U.S. as the leading segment. In the clinical data management niche, nearly every major health IT supplier is infusing AI into their products. For IT and pharmaceutical professionals, keeping track of these tools’ capabilities and maturity is crucial. Pilot testing different solutions, and measuring their impact (on efficiency, data quality, etc.), will inform which vendors truly add value versus hype. Fortunately, many vendors publish performance metrics and case studies, as cited above, which can guide decision-makers. The competition among vendors is also spurring rapid improvements – for example, the accuracy of NLP in 2025 is notably higher than just a few years ago, thanks to advances like transformer models and the race between offerings from Google, Amazon, and others.
Conclusion
Artificial Intelligence is no longer a futuristic concept in U.S. clinical data management – it is here and now, delivering practical improvements in how we handle healthcare and research data. From a doctor’s daily note-writing to a pharmaceutical company’s massive clinical trial, AI is helping to streamline workflows, enhance data accuracy, uncover insights, and maintain compliance. This comprehensive look at AI’s impact shows tremendous benefits: faster documentation, quicker trials, more robust real-world evidence, and predictive capabilities that can save lives. At the same time, it highlights the critical considerations needed to implement AI responsibly. Data privacy must be fiercely protected, biases must be checked, and humans must remain vigilant overseers of their AI partners.
For IT and pharmaceutical professionals, the path forward involves blending domain expertise with these AI tools – leveraging machines for what they do best (handling volume and complexity) while guiding them with human judgment and ethical frameworks. U.S. regulators like the FDA and HHS are actively engaging with AI’s rise, which is reassuring; they are updating guidelines to ensure innovation continues safely and effectively (FDA Issues Draft Guidance on the Role of Artificial Intelligence in Supporting Regulatory Decisions). Early adopters have shared valuable lessons: start with targeted use cases that have clear ROI, involve end-users (clinicians, data managers) in design and training, and establish an AI governance structure from day one.
In 2025, a hospital or pharma company not utilizing AI in some form of data management is increasingly the exception. The competitive and clinical pressures to harness data are too great. Yet, adopting AI is not a one-time project but a journey of continuous improvement – models will need retraining, and new use cases will emerge as technology evolves (for example, the advent of generative AI like GPT is opening new possibilities in summarizing research or even writing draft reports as we saw). One can envision a near future where much of the “heavy lifting” in managing clinical data is handled by intelligent systems in the background, allowing healthcare professionals to focus on decision-making and patient interactions.
In conclusion, AI is proving to be a transformative force in clinical data management in the U.S., driving efficiency, accuracy, and innovation. With proper stewardship, it will play a pivotal role in advancing healthcare quality and accelerating medical breakthroughs, all while upholding the trust and safety that patients and regulators expect. The organizations that successfully integrate AI into their data DNA will be well-positioned to lead in this new era of healthcare intelligence.
DISCLAIMER
The information contained in this document is provided for educational and informational purposes only. We make no representations or warranties of any kind, express or implied, about the completeness, accuracy, reliability, suitability, or availability of the information contained herein. Any reliance you place on such information is strictly at your own risk. In no event will IntuitionLabs.ai or its representatives be liable for any loss or damage including without limitation, indirect or consequential loss or damage, or any loss or damage whatsoever arising from the use of information presented in this document. This document may contain content generated with the assistance of artificial intelligence technologies. AI-generated content may contain errors, omissions, or inaccuracies. Readers are advised to independently verify any critical information before acting upon it. All product names, logos, brands, trademarks, and registered trademarks mentioned in this document are the property of their respective owners. All company, product, and service names used in this document are for identification purposes only. Use of these names, logos, trademarks, and brands does not imply endorsement by the respective trademark holders. IntuitionLabs.ai is an AI software development company specializing in helping life-science companies implement and leverage artificial intelligence solutions. Founded in 2023 by Adrien Laurent and based in San Jose, California. This document does not constitute professional or legal advice. For specific guidance related to your business needs, please consult with appropriate qualified professionals.