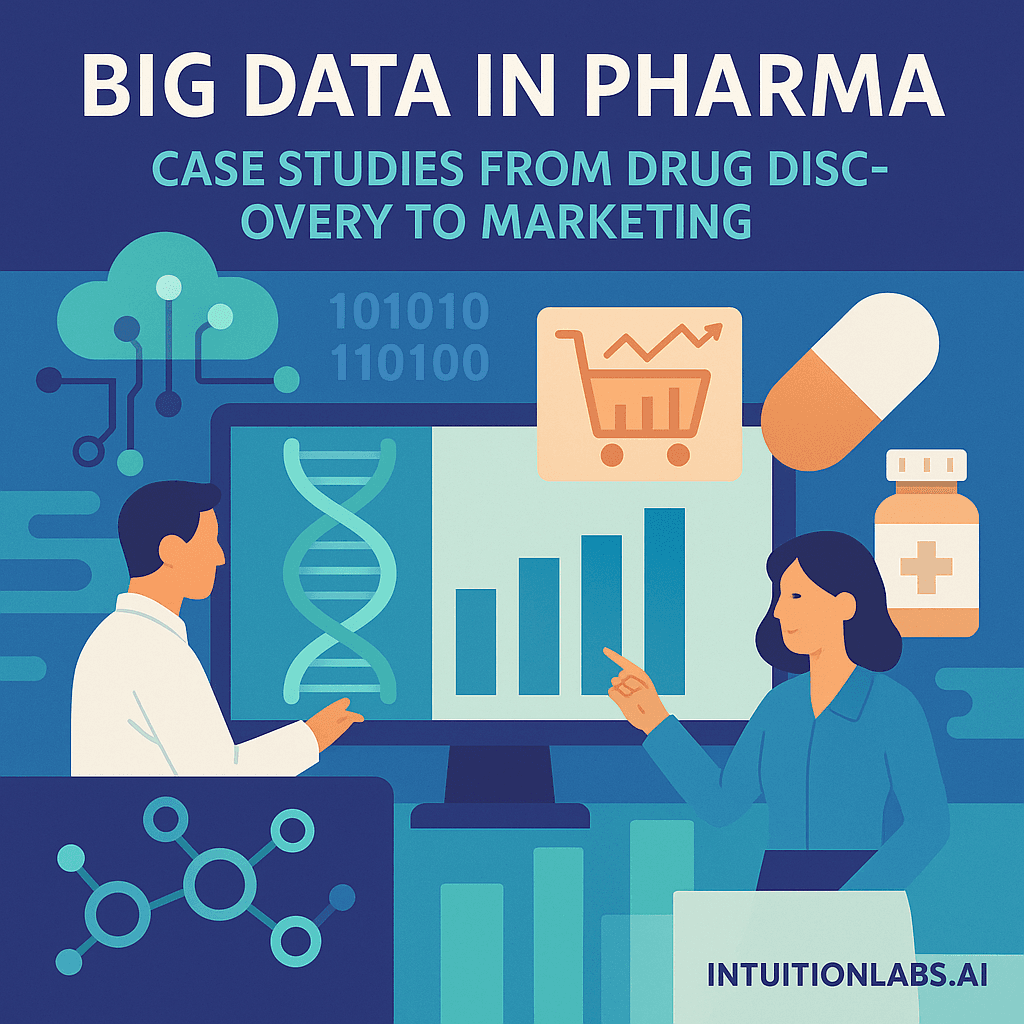
Big Data in Pharma: Case Studies from Drug Discovery to Marketing
Big data is revolutionizing the pharmaceutical industry, enabling companies to glean insights from massive datasets across the drug lifecycle. In recent years, pharma organizations have leveraged cloud computing, AI, and advanced analytics to accelerate drug discovery, optimize clinical trials, enhance pharmacovigilance, streamline manufacturing, and refine marketing strategies. Below, we explore in-depth case studies in each of these domains, highlighting the company involved, the big data technologies used, the specific application and objective, the outcomes achieved, and any challenges encountered.
Drug Discovery and Early Research
Pharmaceutical R&D generates enormous volumes of data from sources like scientific literature, genomic databases, and past experiments. Big data technologies now help researchers sift through these troves to identify new drug candidates or novel uses for existing drugs. One striking example is BenevolentAI, a biotechnology company that applied AI-driven big data analysis to find a treatment for COVID-19. BenevolentAI uses a knowledge graph containing millions of biomedical entities and hundreds of millions of relationships, mined from literature and other sources on the AWS cloud (BenevolentAI Case Study). In early 2020, as the pandemic emerged, the company pivoted to search its data for approved drugs that could be repurposed against the novel coronavirus. By running machine-learning models at scale on AWS, BenevolentAI's platform identified the rheumatoid arthritis drug baricitinib as a potential COVID-19 therapy in a matter of days (BenevolentAI Case Study). Impressively, the AI system sifted through vast datasets and found this candidate with only ~90 minutes of cloud computing time and under three days of human analysis (BenevolentAI Case Study). Within one month, a clinical trial of baricitinib for COVID-19 began, and the drug later proved effective enough to earn emergency use authorization in the U.S. This case showcases how big data and AI dramatically accelerated drug repurposing, providing a viable treatment option much faster than traditional methods. The challenge faced was the urgent need to scour enormous data for a quick answer during a global crisis – a task made feasible by scalable cloud infrastructure and data analytics. BenevolentAI's success highlights the growing role of big data in shortening drug discovery timelines and responding rapidly to emerging health threats (BenevolentAI Case Study).
Clinical Trials and Development
Clinical trials are data-intensive and notoriously time-consuming. Integrating and analyzing data across trials can reveal patterns that improve trial design and speed up development. GlaxoSmithKline (GSK) offers a powerful case study of leveraging big data to make clinical research more efficient. GSK, a 300-year-old pharma company, found itself with over 8 petabytes of trial data spread across 2,100 silos, largely untapped for broader insights (GSK accelerates data analytics for clinical trials - CIO). To break down these silos, GSK built a unified Big Data platform on a Cloudera Hadoop data lake, with pipelines to ingest and harmonize data from thousands of operational systems (GSK accelerates data analytics for clinical trials - CIO). They employed tools like StreamSets (for data ingestion bots) and Trifacta (for cleaning messy data), as well as machine learning tools such as Tamr (for mapping data to standard ontologies) and even Google TensorFlow for advanced analytics (GSK accelerates data analytics for clinical trials - CIO). This homegrown platform enabled researchers to analyze cross-trial data at unprecedented speed. For example, querying clinical trial datasets for a correlation that once took nearly one year now takes about 30 minutes (GSK accelerates data analytics for clinical trials - CIO). Such a dramatic reduction in data processing time had a "huge impact on researcher productivity" according to GSK's Chief Data Officer (GSK accelerates data analytics for clinical trials - CIO). GSK has used the platform for initiatives like analyzing genetic data from 500,000 UK Biobank participants to find new drug targets (GSK accelerates data analytics for clinical trials - CIO). The ultimate objective is to accelerate drug development; GSK hopes that by simulating trials and mining data, it can shrink the typical drug discovery timeline from 5–7 years down to roughly 2 years (GSK accelerates data analytics for clinical trials - CIO). A key challenge was cultural and technical – consolidating decades of legacy trial data and overcoming organizational silos. By investing in a robust data infrastructure and tools, GSK turned its fragmented data into a strategic asset, improving trial efficiency and informing discovery in ways previously impossible without big data technology (GSK accelerates data analytics for clinical trials - CIO) (GSK accelerates data analytics for clinical trials - CIO).
Pharmacovigilance (Drug Safety)
After drugs reach the market, pharmaceutical companies must continuously monitor safety data to detect adverse events and ensure patient safety. Pharmacovigilance generates big data from sources like adverse event reports, electronic health records, and social media. A notable case study is a top 10 global pharmaceutical company that transformed its drug safety monitoring by digitizing adverse event (AE) collection. Traditionally, AE reporting was a slow, manual process: a single safety case could pass through many hands (doctor, call center, data entry, etc.), causing delays and potential information loss. In 2012, this pharma company recognized that its legacy AE collection process was not fast or agile enough and sought a better solution (Case Study: Solving the Adverse Event Communication Bottlenecks with Vigilance Collect - IQVIA). Partnering with IQVIA (a healthcare data firm), the company implemented the IQVIA Vigilance Platform – specifically a module called Vigilance Collect – to capture adverse events directly from the source in real time. This cloud-based system uses web and mobile portals to allow healthcare professionals or patients to submit AE reports directly, bypassing the old multi-step transcription process (Case Study: Solving the Adverse Event Communication Bottlenecks with Vigilance Collect - IQVIA). The big data tech here includes a centralized safety data platform that can intake high volumes of reports and integrate them for analysis. The results have been impressive: as of 2021, the company was processing over 120,000 adverse event cases per year through the new system – more than 15% of all its global case intake (Case Study: Solving the Adverse Event Communication Bottlenecks with Vigilance Collect - IQVIA). Automating and streamlining data capture led to "substantial cost savings and superior pharmacovigilance outcomes," according to IQVIA (Case Study: Solving the Adverse Event Communication Bottlenecks with Vigilance Collect - IQVIA). Safety teams can now detect potential safety signals faster and focus on analysis rather than paperwork. The challenge of integrating this solution involved changing entrenched workflows and ensuring data quality from direct reporter inputs. Nonetheless, this case demonstrates how big data platforms in pharmacovigilance improve compliance and patient safety by speeding up adverse event reporting and analysis (Case Study: Solving the Adverse Event Communication Bottlenecks with Vigilance Collect - IQVIA) (Case Study: Solving the Adverse Event Communication Bottlenecks with Vigilance Collect - IQVIA).
Manufacturing and Supply Chain
In pharmaceutical manufacturing, maximizing quality and uptime is critical – especially for new drugs and clinical trial supplies. Here, big data and IoT (Internet of Things) sensors can drive smarter, more efficient production. Pfizer provides a real-world example through its collaboration with Amazon Web Services (AWS). In 2021 Pfizer and AWS launched the Pfizer-Amazon Collaboration Team (PACT) to apply cloud analytics and machine learning across Pfizer's product development and clinical manufacturing efforts (AWS Helps Pfizer Accelerate Drug Development And Clinical Manufacturing - Pfizer). One focus area was continuous manufacturing of oral solid dose drugs for clinical trials. Pfizer equipped equipment like centrifuges, coating machines, and other production gear with sensors, and AWS helped deploy a predictive maintenance solution to analyze this streaming equipment data (AWS Helps Pfizer Accelerate Drug Development And Clinical Manufacturing - Pfizer). The technology stack included AWS's big data and AI services: Amazon SageMaker for building and deploying machine learning models, Amazon Lookout for Equipment for detecting anomalous machine behavior from sensor readings, Amazon Lookout for Metrics for anomaly detection in process metrics, and Amazon QuickSight for data visualization (AWS Helps Pfizer Accelerate Drug Development And Clinical Manufacturing - Pfizer). By training ML models on historical sensor data, Pfizer's team developed a system that provides early warnings of equipment issues with minimal false alarms (AWS Helps Pfizer Accelerate Drug Development And Clinical Manufacturing - Pfizer). In practice, this means the system can flag subtle changes in vibration, temperature, or pressure that might precede a machine failure. As a result, Pfizer can proactively service equipment before a breakdown occurs, thus reducing unplanned downtime in production (AWS Helps Pfizer Accelerate Drug Development And Clinical Manufacturing - Pfizer). Ensuring high equipment uptime is especially vital when producing drugs for clinical trials or launch, where delays can set back R&D timelines. The outcome of this big data initiative is a more reliable manufacturing process that can produce new drugs faster and more reliably for testing (AWS Helps Pfizer Accelerate Drug Development And Clinical Manufacturing - Pfizer) (AWS Helps Pfizer Accelerate Drug Development And Clinical Manufacturing - Pfizer). One challenge in such projects is integrating diverse legacy equipment and sensor data into a unified platform, as well as retraining staff to trust and use AI-driven maintenance alerts. Pfizer's case illustrates how embracing industrial big data analytics in pharma manufacturing can improve operational efficiency and ultimately get treatments to patients sooner.
Marketing and Commercial Strategy
Pharmaceutical companies also use big data analytics to refine their marketing and sales strategies. By analyzing large datasets on prescriber habits, patient demographics, and market trends, companies can tailor their marketing efforts for greater impact. In one case study, a leading global pharma company worked with consultants to implement an AI-driven "Next Best Action" (NBX) system for its sales and marketing teams. The challenge was to boost prescription rates for its product in a highly competitive market – essentially, to ensure that marketing and sales efforts were as effective as possible in persuading healthcare providers to prescribe their drug. The solution involved integrating a wide variety of data: internal data (sales figures, call logs from sales reps, physician profiles, etc.), external data (market share, claims data, rival product info), and even qualitative research (surveys and interviews with doctors and sales reps). This big dataset was then mined using machine learning to identify which sequences of interactions tend to lead to increased prescriptions. In other words, the company analyzed thousands of past marketing "touchpoints" to find an optimal engagement strategy for each customer. From this analysis, the team derived a data-driven NBX model that would recommend the best next action for each sales representative – for example, whether to send a physician an email with clinical data, invite them to a webinar, or schedule a face-to-face meeting, depending on what approach proved most effective in similar situations. The results were compelling: in a pilot across several markets, clinics that were managed using the NBX recommendations saw 30% higher product sales growth compared to those that weren't (Case study: Uplifting sales in pharma with AI insights - PwC Switzerland). Moreover, sales reps who followed the AI-driven suggestions achieved sales about 1.5× higher than their peers who did not (Case study: Uplifting sales in pharma with AI insights - PwC Switzerland). These improvements translate into significantly increased revenue and better return on marketing investment. The main challenge here was the "last mile" problem of making analytics actionable – i.e. ensuring the insights reached reps in a convenient, timely way and that the reps trusted the recommendations. By integrating the NBX system into the reps' workflow (for instance, as suggestions in their CRM software) and demonstrating clear gains, the company overcame user skepticism. This case underscores how big data and AI can personalize marketing at scale in pharma – identifying the right message, time, and channel for each healthcare provider to maximize engagement and ultimately improve sales.
Comparative Summary of Case Studies
To summarize, the table below compares the key aspects of each case study across the pharma value chain:
Domain | Company/Org | Big Data Tech Used | Application & Objective | Outcome | Challenges |
---|---|---|---|---|---|
Drug Discovery | BenevolentAI (UK startup; used by Eli Lilly) | AWS cloud; AI/ML with a large biomedical knowledge graph (BenevolentAI Case Study) | AI-driven drug repurposing – identify existing drugs for new disease (COVID-19) | Found baricitinib as a COVID-19 treatment in days, using 90 min compute + 3 days analysis; clinical trial started within 1 month (BenevolentAI Case Study) | Urgent need to analyze vast scientific data rapidly (pandemic); required scalable compute and data integration |
Clinical Trials | GlaxoSmithKline (GSK) | Data lake (Cloudera Hadoop) with integration & analytics tools (StreamSets, Trifacta, Tamr, TensorFlow, etc.) (GSK accelerates data analytics for clinical trials - CIO) | Unified clinical trial data platform to enable cross-trial analysis and faster trial design (supporting drug discovery) | Data queries that once took ~1 year now run in ~30 minutes (GSK accelerates data analytics for clinical trials - CIO), greatly improving R&D productivity; aiming to cut drug discovery timeline from 5–7 years to ~2 years (GSK accelerates data analytics for clinical trials - CIO) | Siloed legacy data (~2,100 separate repositories) (GSK accelerates data analytics for clinical trials - CIO); required cultural shift and new data governance to treat data as a shared asset |
Pharmacovigilance (Safety) | Unnamed Top-10 Pharma (via IQVIA) | IQVIA Vigilance Collect platform (cloud-based portals & database) (Case Study: Solving the Adverse Event Communication Bottlenecks with Vigilance Collect - IQVIA) | Digital AE reporting – collect adverse event data directly from patients and HCPs to speed up safety surveillance | >120,000 adverse event cases/year (≈15% of all reports) now captured through the digital system (Case Study: Solving the Adverse Event Communication Bottlenecks with Vigilance Collect - IQVIA); yielded cost savings and improved safety oversight (Case Study: Solving the Adverse Event Communication Bottlenecks with Vigilance Collect - IQVIA) | Re-engineering legacy reporting processes; integrating multiple stakeholders (patients, doctors, call centers) into a unified digital workflow; ensuring data quality and compliance |
Manufacturing | Pfizer (PACT with AWS) | Industrial IoT sensors + AWS ML services (Amazon SageMaker, Lookout for Equipment/Metrics, QuickSight) (AWS Helps Pfizer Accelerate Drug Development And Clinical Manufacturing - Pfizer) | Predictive maintenance for drug production equipment to maximize uptime and ensure reliable supply for trials | Developed ML models giving early warning of equipment issues (with few false positives) (AWS Helps Pfizer Accelerate Drug Development And Clinical Manufacturing - Pfizer); enabled Pfizer to detect anomalies in real time and schedule maintenance, reducing unplanned downtime (AWS Helps Pfizer Accelerate Drug Development And Clinical Manufacturing - Pfizer) | Handling huge volumes of sensor data from manufacturing; needed to integrate legacy equipment with cloud analytics; change management for adopting AI-driven operations |
Marketing & Sales | Unnamed Global Pharma (via PwC case) | Integrated analytics platform combining internal & external data; machine learning for pattern mining (Next-Best-Action system) | Next Best Action recommendations – analyze big datasets on HCP behavior to optimize sales rep outreach and marketing tactics | Pilot saw +30% sales growth in targeted institutions vs. others, and reps using the AI suggestions achieved 1.5× higher sales than peers (Case study: Uplifting sales in pharma with AI insights - PwC Switzerland) | "Last mile" adoption by users – had to integrate AI insights seamlessly into reps' workflow and overcome distrust; also required merging very diverse data sources (sales, CRM, medical claims, etc.) for the AI model |
Conclusion
These case studies demonstrate the transformative impact of big data in pharma, from R&D to commercialization. By investing in modern data platforms, cloud computing, and AI analytics, pharmaceutical organizations have achieved tangible benefits: faster drug discovery, more efficient clinical trials, stronger safety surveillance, leaner manufacturing, and smarter marketing. Each example also highlights challenges – whether technical (integrating siloed or real-time data) or human (gaining user trust, changing legacy processes) – that IT professionals must navigate. For pharma IT leaders in the U.S. and globally, the success of these initiatives underscores the importance of treating data as a strategic asset. Big data technologies, when applied with clear objectives and executive support, are helping pharma companies bring therapies to market faster, operate more efficiently, and ultimately improve patient outcomes (GSK accelerates data analytics for clinical trials - CIO) (Case Study: Solving the Adverse Event Communication Bottlenecks with Vigilance Collect - IQVIA). As the industry continues to generate ever-growing data volumes (from genomics, health records, wearables, etc.), the ability to harness big data will remain a key competitive differentiator in delivering innovative medicines to patients. Each of the real-world cases above serves as an inspiring blueprint for leveraging data-driven insights in the pharmaceutical domain.
DISCLAIMER
The information contained in this document is provided for educational and informational purposes only. We make no representations or warranties of any kind, express or implied, about the completeness, accuracy, reliability, suitability, or availability of the information contained herein. Any reliance you place on such information is strictly at your own risk. In no event will IntuitionLabs.ai or its representatives be liable for any loss or damage including without limitation, indirect or consequential loss or damage, or any loss or damage whatsoever arising from the use of information presented in this document. This document may contain content generated with the assistance of artificial intelligence technologies. AI-generated content may contain errors, omissions, or inaccuracies. Readers are advised to independently verify any critical information before acting upon it. All product names, logos, brands, trademarks, and registered trademarks mentioned in this document are the property of their respective owners. All company, product, and service names used in this document are for identification purposes only. Use of these names, logos, trademarks, and brands does not imply endorsement by the respective trademark holders. IntuitionLabs.ai is an AI software development company specializing in helping life-science companies implement and leverage artificial intelligence solutions. Founded in 2023 by Adrien Laurent and based in San Jose, California. This document does not constitute professional or legal advice. For specific guidance related to your business needs, please consult with appropriate qualified professionals.