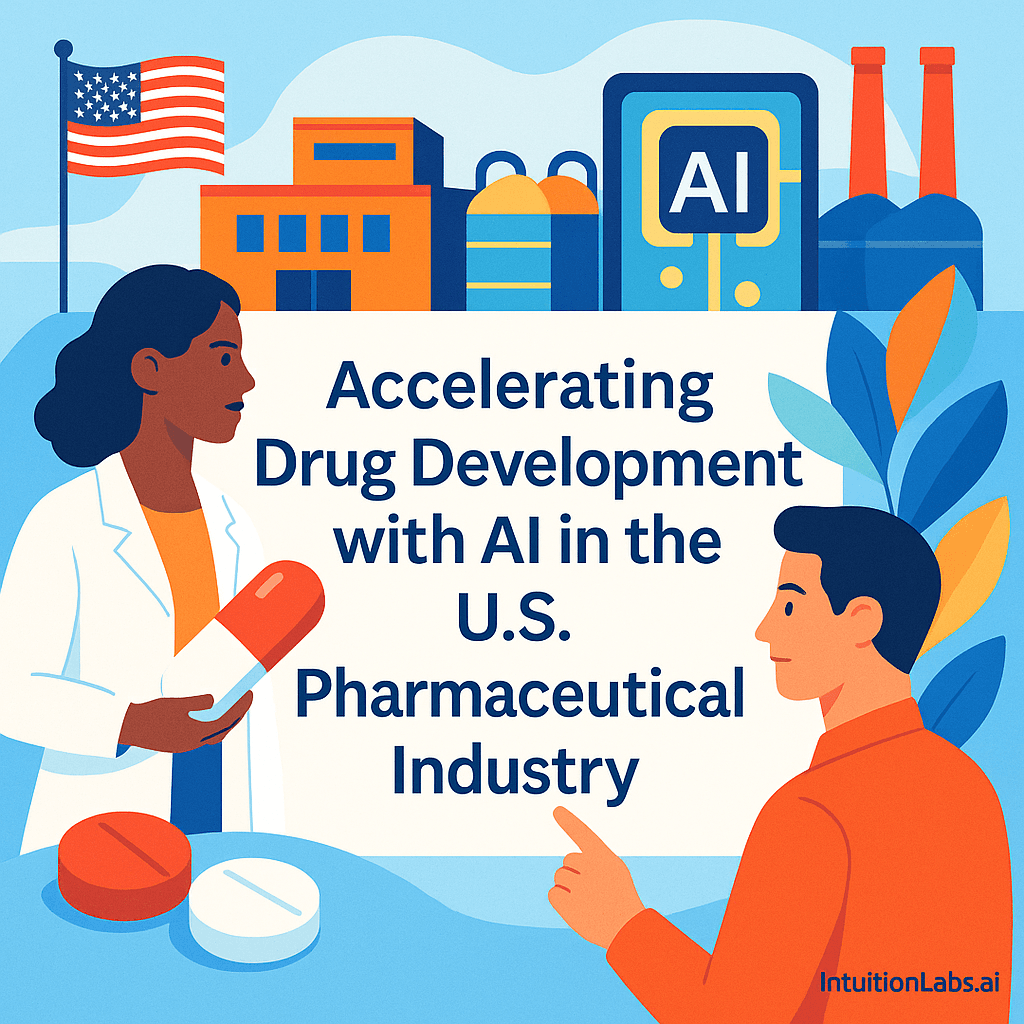
Accelerating Drug Development with AI in the U.S. Pharmaceutical Industry
Introduction
Bringing a new drug to market is a notoriously lengthy and expensive endeavor. The end-to-end process from initial discovery through FDA approval traditionally takes on the order of a decade or more – often 15 to 16 years from discovery to delivery (AI Can Transform Drug Development, New ITIF Report Finds-ITIF) – and costs billions of dollars. Moreover, the success rate is dismally low: only about 8% of early-stage drug candidates ultimately make it to market, with average R&D expenses per approved drug exceeding $2.8 billion (AI Can Transform Drug Development, New ITIF Report Finds-ITIF). This protracted timeline delays patient access to life-saving therapies and drives up healthcare costs. There is growing optimism that artificial intelligence (AI) can help reverse these trends by streamlining each phase of the pharmaceutical R&D pipeline. In fact, early evidence suggests AI could slash development times by roughly half (AI Can Transform Drug Development, New ITIF Report Finds-ITIF), by enhancing productivity from drug discovery and preclinical testing to clinical trials, regulatory review, and even manufacturing.
This report provides a comprehensive look at how AI technologies are reducing drug development timelines in the United States, focusing on impacts at each stage of the pipeline. We will explore the applications of AI in drug discovery, preclinical development, clinical trials, and regulatory processes, backed by up-to-date statistics and case studies. Notably, we highlight real-world examples where AI has accelerated development, such as AI-designed molecules reaching trials in record time and AI-optimized clinical trials. We also address the limitations, risks, and regulatory considerations that come with deploying AI in pharma R&D. The goal is to give IT professionals and pharma stakeholders a clear, technically-informed view of how AI is reshaping drug development in the U.S. and what that means for time-to-market. All assertions are supported by recent data and reputable sources, including scientific publications and guidance from U.S. regulatory bodies (e.g. the FDA).
The Traditional Drug Development Timeline
Developing a new therapeutic is traditionally a multi-phase process that spans many years. At a high level, the pipeline consists of: Drug Discovery (identifying a promising molecule or biologic), Preclinical testing (lab and animal studies to assess safety and mechanism), Clinical trials (Phases I–III in humans to establish safety and efficacy), and Regulatory review (FDA evaluation and approval). Figure 1 summarizes typical durations and success rates for these stages under conventional approaches:
Stage | Conventional Duration & Success | Key Activities |
---|---|---|
Discovery & Lead Identification | ~3–6 years to identify viable drug leads (target discovery, compound screening, hit-to-lead). Very high attrition; thousands of compounds may yield 1 candidate (Exscientia claims world first as AI-created drug enters clinic-pharmaphorum). | Basic research, target validation, high-throughput screening of chemical libraries, lead optimization. |
Preclinical Development | ~1–2 years of in vitro and animal studies. Only ~1 in 10 compounds entering preclinical will reach clinical stage (AI Can Transform Drug Development, New ITIF Report Finds-ITIF). | In vitro assays (cellular models), in vivo animal testing for toxicity and pharmacokinetics, manufacturing scale-up for trials. |
Clinical Trials (Phase I–III) | ~6–8 years on average for human trials (Phase I ~2 years, II ~3 years, III ~3 years) (What's the average time to bring a drug to market in 2022? - N-SIDE). Cumulative success probability ~10% (Phase I to approval) (AI-Generated Drug Candidates Are Here, But Clinical Trial Wins Need More Time - MedCity News). | Phase I: safety in 20–100 healthy volunteers; Phase II: efficacy in 100–300 patients; Phase III: large-scale (1000+ patients) efficacy and safety. |
Regulatory Review & Approval | ~0.5–2 years for FDA review, advisory committees, and final approval (if successful). | New Drug Application (NDA) or Biologics License Application (BLA) preparation and submission; FDA evaluation of all data for safety/efficacy; manufacturing quality inspections. |
Figure 1. Conventional drug development phases, with typical time frames and success rates. It often takes 10–15 years (or more) and countless experiments to progress from a lab discovery to an FDA-approved drug (AI Can Transform Drug Development, New ITIF Report Finds-ITIF) (AI-Generated Drug Candidates Are Here, But Clinical Trial Wins Need More Time - MedCity News). The lengthy clinical trial stages are the primary bottleneck, consuming over half of the total timeline (How long do clinical trial phases take? - Antidote.me). Each step is fraught with risk – for every 10 compounds that start human trials, roughly 9 will fail at some point (AI-Generated Drug Candidates Are Here, But Clinical Trial Wins Need More Time - MedCity News). Given this lengthy and risky process, there is intense interest in any technology that can compress timelines or improve success rates. Artificial intelligence has emerged as a leading candidate, with applications across all stages of development. Below, we examine how AI is being applied at each phase to accelerate the journey of a drug to market.
AI in Drug Discovery and Early Development
The application of AI in the drug discovery stage has demonstrated some of the most dramatic time savings to date. Traditionally, discovering a new drug candidate is a laborious iteration of hypothesis-driven research and trial-and-error testing. Scientists might screen thousands to millions of compounds to find a few “hits”, then optimize those to a lead candidate – a process that often takes 4–6 years of chemistry and biology effort. AI-driven approaches are fundamentally changing this by using algorithms to search chemical and genomic space far more efficiently:
- Target Identification: AI models (including machine learning on genomics data and knowledge graphs) can analyze vast biomedical datasets to uncover novel drug targets or disease pathways. For example, AI can sift through omics data to identify genes or proteins likely involved in a disease, which might be missed by manual analysis. This can shorten the time needed to decide “what to target” in the first place.
- Virtual Screening and Molecule Design: Instead of physically testing every compound, in silico screening uses AI algorithms (e.g. deep learning or predictive models) to evaluate millions of chemical structures for likely activity against a target. This narrows down candidate molecules much faster. Advanced AI techniques like generative models can even propose novel molecular structures with desired properties (a process known as de novo drug design).
Evidence of AI’s impact in discovery is compelling. Notably, the first AI-designed drug candidates have reached human trials in a fraction of the typical time. One landmark example is the molecule DSP-1181, developed by the startup Exscientia in partnership with Sumitomo Dainippon Pharma. Using an AI-driven platform to design and optimize the compound, they brought DSP-1181 (an OCD therapy) from project start to clinical trial in only 12 months – compared to about 5 years normally (Exscientia claims world first as AI-created drug enters clinic-pharmaphorum). The AI system dramatically reduced the number of compounds that had to be synthesized and tested, finding a promising candidate after exploring only 350 compounds (versus ~2,500 compounds typically) (Exscientia claims world first as AI-created drug enters clinic-pharmaphorum). This efficiency gain (an 85% reduction in compounds needed) saved enormous time and cost. Similarly, Insilico Medicine reported using AI to identify a novel drug target and design a lead molecule for idiopathic pulmonary fibrosis, advancing it through preclinical testing to Phase I readiness in under 18 months, at roughly 10% of the cost of traditional programs (Insilico: linking target discovery and generative chemistry AI platforms for a drug discovery breakthrough) (Insilico: linking target discovery and generative chemistry AI platforms for a drug discovery breakthrough). That molecule (later known as ISM001-055) successfully entered human trials in 2021 (Insilico Medicine provides benchmark timelines from its 22 AI-designed drug candidates) and by 2024 had shown positive Phase IIa results in patients (Insilico Medicine provides benchmark timelines from its 22 AI-designed drug candidates) – a pace virtually unheard of in pulmonary fibrosis research.
AI can also assist in drug repurposing, which is identifying existing approved drugs that might treat other diseases. A striking case occurred during the COVID-19 pandemic: in January 2020, AI models at BenevolentAI identified the rheumatoid arthritis drug baricitinib as a potential treatment for COVID-19 in just 48 hours (FDA Converts Emergency Approval Of Baricitinib — First Identified As A COVID Treatment By BenevolentAI — To A Full Approval-BenevolentAI (AMS: BAI)). This AI-driven hypothesis (suggesting baricitinib could inhibit the virus’s entry and tame the inflammatory “cytokine storm”) was published in The Lancet in February 2020 (FDA Converts Emergency Approval Of Baricitinib — First Identified As A COVID Treatment By BenevolentAI — To A Full Approval-BenevolentAI (AMS: BAI)). Clinical trials quickly confirmed the benefit, and baricitinib obtained an FDA Emergency Use Authorization by November 2020 for hospitalized COVID-19 patients, followed by full approval in 2022 (FDA Converts Emergency Approval Of Baricitinib — First Identified As A COVID Treatment By BenevolentAI — To A Full Approval-BenevolentAI (AMS: BAI)) (FDA Converts Emergency Approval Of Baricitinib — First Identified As A COVID Treatment By BenevolentAI — To A Full Approval-BenevolentAI (AMS: BAI)). This repurposing success – going from idea to authorized therapy in under a year – showcased how AI’s speed in discovery can translate to real-world impact in a crisis.
Beyond finding molecules faster, AI is improving the quality of early drug candidates, which can prevent costly failures later. By learning from massive chemical and biological data, AI models can predict properties like a compound’s toxicity, bioavailability, or potency before it’s ever made in the lab. This means researchers can prioritize higher-probability candidates and drop problematic ones sooner. Indeed, early statistics suggest that AI-discovered compounds have higher success rates in initial trials. As of late 2023, 24 AI-designed molecules had entered Phase I trials and 21 successfully passed Phase I (an ~85–88% success rate) (The convergence of AI technologies and human expertise in pharma R&D). This is nearly double the historical Phase I success rate of ~40–65% (The convergence of AI technologies and human expertise in pharma R&D). While the sample is still small, it hints that AI’s ability to select better drug candidates (e.g. by avoiding toxic or ineffective compounds) could translate into more efficient development with fewer failures.
Overall, AI in the discovery stage is shrinking timelines from years to months for certain steps. It’s achieving this by automating the search through chemical space, generating and evaluating ideas much faster than humans alone, and leveraging patterns in big data that humans might overlook. From target identification to lead generation, AI acts as a force-multiplier for researchers. In practical terms, this means a drug discovery project that might have taken five years in the past could potentially reach a viable lead in a year or two with AI – as demonstrated by real case studies (Exscientia claims world first as AI-created drug enters clinic-pharmaphorum) (Insilico Medicine provides benchmark timelines from its 22 AI-designed drug candidates). These time savings at the front of the pipeline then feed into a faster start for preclinical and clinical development.
AI in Preclinical Development
After a lead compound is identified, it must undergo rigorous preclinical testing before human trials can begin. This phase typically involves refining the compound’s properties and assessing its safety in laboratory and animal studies – a process that can take several years. AI is accelerating preclinical development through a combination of predictive modeling, automation, and even replacement of certain traditional experiments:
- In Silico Toxicology and Pharmacology: One of the most time-consuming aspects of preclinical work is evaluating a compound’s safety (toxicity, carcinogenicity, etc.) and pharmacokinetics (absorption, distribution, metabolism, excretion). AI models trained on large datasets of chemical structures and biological assay results can predict these properties with increasing accuracy. For example, machine learning models can flag molecules likely to have off-target toxic effects or poor metabolic profiles before they go through months of animal testing. By filtering out unsafe or nonviable candidates early, AI helps avoid dead-ends and focuses resources on the most promising compounds. This translates to time saved by not running unnecessary experiments on doomed candidates.
- Automated Experimentation (“Lab-in-the-Loop”): AI is also used to guide laboratory experiments in an iterative loop, sometimes called “robot scientists” or closed-loop optimization. A notable example is Genentech’s AI-driven “lab-in-the-loop” platform, which continuously refines its predictive models based on real-time experimental results (AI Can Transform Drug Development, New ITIF Report Finds-ITIF). The system proposes new compound variants to test, the experiments are run (often using robotics for high-throughput synthesis and screening), results are fed back into the AI model, and the model updates its predictions for the next round. This cycle can rapidly hone in on an optimized drug candidate. Genentech reports that this approach has significantly cut down discovery and lead optimization timelines (AI Can Transform Drug Development, New ITIF Report Finds-ITIF) by reducing the number of cycles needed to arrive at a good candidate. Essentially, AI is making the preclinical research process more iterative and data-driven rather than purely trial-and-error.
- Reducing Animal Testing: In vivo animal studies are a classic bottleneck – they are slow, expensive, and ethically sensitive. Advances in AI, combined with new in vitro systems, are enabling virtual or alternative preclinical models. Recognizing this potential, U.S. regulators have begun to support non-animal approaches. The FDA Modernization Act 2.0 (2022) for the first time authorized the use of alternatives to animal testing for drug development, paving the way for greater use of AI-driven methods (FDA to phase out animal testing with AI and lab-based models - Clinical Trials Arena) (FDA to phase out animal testing with AI and lab-based models - Clinical Trials Arena). In 2025, the FDA announced plans to phase out certain animal testing in favor of “more effective, human-relevant methods,” explicitly including AI-based models and lab-on-a-chip systems (FDA to phase out animal testing with AI and lab-based models - Clinical Trials Arena). This is a significant policy shift expected to accelerate preclinical timelines. For instance, instead of breeding animal models and observing them for weeks or months, researchers might use AI to predict a drug’s toxic dose or likely side effects in minutes using computer simulations of human biology. Similarly, AI can analyze data from microphysiological systems (organ-on-chip devices) to predict clinical outcomes faster than animal studies. By complementing or even replacing some animal tests, AI not only saves time but can yield more human-relevant insights earlier.
Another area where AI contributes is formulation and process development – figuring out how to manufacture the drug compound at scale and in a form suitable for patients (pills, injections, etc.). Machine learning can optimize formulation parameters or manufacturing processes (like choosing the best synthesis route) more quickly by learning from past production data. Companies like Asimov are applying AI to enhance biologics manufacturing; for example, Asimov’s AI tools in gene therapy manufacturing have improved scalability and quality (AI Can Transform Drug Development, New ITIF Report Finds-ITIF), which can shorten the timeline to ramp up production for trials. While manufacturing comes later in the pipeline, early consideration of manufacturability via AI can prevent delays down the road.
Overall, AI in preclinical development acts as an accelerator by shrinking the design-test-analyze cycle times. A fully AI-integrated preclinical program was able to deliver a development candidate and complete IND-enabling studies in under 18 months (as seen with Insilico’s IPF project) (Insilico: linking target discovery and generative chemistry AI platforms for a drug discovery breakthrough) (Insilico Medicine provides benchmark timelines from its 22 AI-designed drug candidates), whereas conventionally this might take 3–5 years. It’s important to note that even with AI, some preclinical steps (e.g. certain toxicity tests) cannot be skipped entirely due to regulatory requirements. However, AI can augment these steps – for example, guiding dose selection for animal studies so that fewer iterations are needed, or identifying biomarkers that allow shorter studies. With supportive regulatory changes, like the FDA’s openness to AI and in vitro alternatives, the preclinical phase is poised to become much more efficient. This means drugs can move into human testing faster, shaving off years from the timeline to market.
AI in Clinical Trials
The clinical trial phase is often the longest and most expensive segment of drug development, typically spanning multiple years and hundreds to thousands of patients. Here, AI has enormous potential to streamline operations, reduce delays, and even improve success probabilities. Key applications of AI in clinical development include patient recruitment optimization, adaptive trial design, real-time data analysis, and predictive analytics for decision-making. We examine each and present current evidence of their impact:
-
Patient Recruitment and Enrollment: One of the biggest causes of trial delays is slow patient enrollment – finding eligible participants who meet the trial criteria. AI can dramatically accelerate this process by mining large health data sources (electronic health records, medical claims, patient registries, etc.) to identify candidates. Machine learning models can match trial inclusion/exclusion criteria against millions of patient records in minutes, flagging likely eligible patients for outreach. Pharmaceutical companies are using AI to scan databases and even social media to locate patients with rare diseases or specific genetic profiles for precision trials. This can cut recruitment time from many months to just weeks in some cases. In fact, AI-driven patient recruitment is already improving metrics: for example, in oncology trials (where finding the right patients is notoriously challenging), AI tools have been shown to improve enrollment rates and reduce screen failure rates (Global AI In Clinical Trials Market Trends Current Partnerships) (Global AI In Clinical Trials Market Trends Current Partnerships). An IQVIA case study cited by one industry report showed that using AI/ML to streamline site selection and feasibility surveys led to a 90% reduction in the time required for those startup activities (Global AI In Clinical Trials Market Trends Current Partnerships). Faster site activation and enrollment means trials reach their target sample size sooner, directly shortening the overall trial timeline.
-
Adaptive Trial Design and Analysis: AI enables more adaptive and efficient trial designs that can save time compared to traditional fixed designs. For instance, AI can simulate trial outcomes under various parameters (dosages, endpoints, patient subgroups) to help designers choose an optimal trial setup that might reach conclusions faster. During a trial, AI-based analytics can process incoming data in real-time and suggest modifications. Modern “adaptive trials” allow modifications such as dynamic patient randomization, dropping ineffective arms early, or adjusting dosage based on interim results, all under pre-specified statistical rules. AI algorithms are ideal for monitoring data and making these complex decisions swiftly. A tangible example was reported where a biopharma company shortened a trial’s duration by 15–30% by using AI to select better endpoints (Global AI In Clinical Trials Market Trends Current Partnerships). In that case (a rare disease trial), the AI identified an endpoint measurable via more frequent or easily obtained data (like a blood biomarker) instead of a traditional clinical outcome that would have taken much longer to observe (Global AI In Clinical Trials Market Trends Current Partnerships). By focusing on a faster-readout endpoint that still correlates with patient benefit, the trial could conclude sooner without sacrificing scientific validity. Similarly, AstraZeneca’s partnership with Immunai to apply AI in an immuno-oncology trial helped optimize dose selection and biomarker use, reportedly reducing trial duration by up to 25% in those cancer trials (Global AI In Clinical Trials Market Trends Current Partnerships). These examples show that AI’s ability to crunch data and model scenarios can directly translate into months or even years saved in clinical testing.
-
Data Management and Monitoring: Clinical trials generate massive amounts of data (labs, imaging, patient-reported outcomes, etc.). AI can automate and enhance the processing of this data, leading to faster insights and decisions. Machine learning models can detect safety signals or efficacy trends earlier by analyzing interim data for patterns that human monitors might miss. For example, AI might identify subtle correlations indicating an adverse effect long before it becomes obvious, allowing a sponsor to address the issue or adjust the trial. On the positive side, if a treatment is performing exceedingly well, AI might recognize a clear efficacy signal early, which could justify stopping the trial early for success (ethical reasons) or moving into an accelerated approval pathway. Additionally, AI-powered natural language processing (NLP) can automate the cleaning and structuring of data from diverse sources, reducing the lag between data collection and analysis. Overall, these efficiencies speed up the time to data lock and analysis at trial end. By cutting down the manual labor in data management, AI frees researchers to focus on interpreting results and making faster go/no-go decisions for the next phase.
-
Improving Success Rates with AI: One of the more profound potential impacts of AI in trials is improving the likelihood that trials succeed, which indirectly accelerates time to market by avoiding failures and repeat trials. AI can help ensure trials are designed with the right patient population – for instance, using predictive models to find patients most likely to respond to the therapy, which can increase the measured efficacy. AI can also enhance patient stratification, identifying subgroups based on biomarkers who benefit the most, thereby yielding clearer positive results. Major pharma companies are leveraging this; for example, Johnson & Johnson deployed AI tools to improve clinical trial inclusivity and diversity (AI Can Transform Drug Development, New ITIF Report Finds-ITIF), ensuring broader representation. A more inclusive trial that reflects the real-world patient population can both speed up recruitment and produce results that regulators consider more robust (potentially streamlining the approval decision). Additionally, success rates might improve simply because AI-selected drug candidates entering trials are of higher quality (as discussed earlier). Early data suggests that AI-originated drugs are faring well in trials so far, with significantly higher Phase I success (The convergence of AI technologies and human expertise in pharma R&D). If these trends continue through Phase II and III, it could mean fewer trial failures and faster development overall.
The cumulative effect of these AI applications is substantial. Industry analysts project that by 2030, AI will be integrated into 60–70% of clinical trials, resulting in dramatically faster timelines and cost savings (Global AI In Clinical Trials Market Trends Current Partnerships). One estimate is an annual saving of $20–30 billion for the industry through efficiency gains and shorter trial durations (Global AI In Clinical Trials Market Trends Current Partnerships). In practical terms, trial phases that each took several years might be shortened on the order of 20–50%. Indeed, a 2020 analysis by the ITIF found that AI could cut the length of clinical phases by half or more by automating tasks and improving trial design (Fact of the Week: Artificial Intelligence Can Save Pharmaceutical Companies Almost $54 Billion in R&D Costs Each Year-ITIF). We are already seeing signals of this future: decentralized trials with AI-based remote monitoring showed the potential to reduce overall Phase II–III timelines and delivered an additional ~$20 million value per drug, according to a Tufts CSDD study (The convergence of AI technologies and human expertise in pharma R&D).
It’s worth noting that as of 2025, no completely “AI-developed” drug has finished all trial phases and been approved yet – the first wave of AI-discovered drugs are mostly in Phase I or II. There have been some setbacks too, reminding us that AI is not magic. For example, in 2023 a few much-touted AI-designed compounds did not meet endpoints in trials for atopic dermatitis, schizophrenia, and cancer, causing those programs to be reevaluated (AI-Generated Drug Candidates Are Here, But Clinical Trial Wins Need More Time - MedCity News). These failures underscore that while AI can improve odds, it cannot eliminate the inherent risk of drug development. Nonetheless, the broad trend is that AI is reshaping clinical development: making trials faster, more adaptive, and data-driven. As more trials incorporate AI for various functions, the expectation is that the clinical stage will cease to be the intractable bottleneck it once was, thereby bringing effective drugs to patients sooner.
AI in Regulatory Review and Approval
Even after successful trials, a drug must navigate the regulatory process – in the U.S., this means convincing the Food and Drug Administration that the drug is safe and effective for its intended use. Regulatory review involves the submission of extensive documentation (sometimes 100,000+ pages of data), analysis by FDA scientists, and often iterative Q&A between the agency and the sponsor. AI’s role in this stage is emerging on two fronts: supporting the industry in preparing stronger, faster submissions and assisting regulators in efficiently reviewing data. Additionally, regulators themselves are actively developing guidelines to govern AI’s use in drug development, which can indirectly expedite approvals by clarifying the rules of the game.
On the industry side, companies are exploring AI tools to compile and organize submission data more efficiently. For instance, NLP algorithms can help auto-generate parts of the regulatory submission by collating results from multiple trial reports, ensuring consistency in how data is presented. AI can cross-check for errors or omissions in the vast datasets before submission (a form of quality control to avoid regulatory delays). Some sponsors use AI to predict what questions regulators are likely to ask, allowing them to proactively address those in the filing. All of this can reduce the back-and-forth once an application is filed. Moreover, AI simulations (sometimes called in silico trials) might be used to supplement clinical data – for example, to provide additional evidence of how the drug might work in subpopulations not extensively tested. While regulatory acceptance of simulation results is still cautious, the FDA has shown openness to modeling and simulation in areas like dose selection and extrapolating pediatric data, which are essentially forms of AI-driven analysis in the approval package. Faster and more thorough submissions can shorten the review clock by minimizing the need for clarification or additional analyses post-submission.
The FDA itself is gearing up to leverage AI and accommodate AI-driven submissions. In January 2025, the FDA issued its first draft guidance on the use of AI in supporting drug regulatory decisions (FDA Issues Draft Guidance on the Role of Artificial Intelligence in Supporting Regulatory Decisions). This guidance provides a risk-based framework for sponsors to establish the credibility of AI models used in drug development (FDA Issues Draft Guidance on the Role of Artificial Intelligence in Supporting Regulatory Decisions). Essentially, the FDA is saying: if you want to use AI-generated evidence (e.g. an AI model’s prediction of toxicity or efficacy) to support your drug application, you need to demonstrate the model is credible and explainable to an appropriate degree. The guidance outlines expectations depending on the AI’s context of use – for example, using AI to analyze clinical trial data or choose trial participants is considered a high-impact use and thus requires thorough documentation of the model’s training data, performance, and potential biases (AI Drug Development: FDA Releases Draft Guidance-Foley & Lardner LLP) (AI Drug Development: FDA Releases Draft Guidance-Foley & Lardner LLP). FDA Commissioner Robert Califf highlighted that with proper safeguards, AI has “transformative potential to advance clinical research and accelerate medical product development” (FDA Issues Draft Guidance on the Role of Artificial Intelligence in Supporting Regulatory Decisions), emphasizing the agency’s support for innovation provided that standards are met. This regulatory clarity is crucial: it gives companies a roadmap to follow so that using AI won’t become a roadblock at the approval stage. As long as they validate their models and provide transparency per FDA guidelines, AI outputs can be integrated into the evidence package.
Another area is post-market surveillance and pharmacovigilance, which, while after initial approval, is part of the extended lifecycle. AI can monitor real-world data (like electronic health records, insurance claims, social media) for adverse events or safety signals once a drug is on the market. Early detection of issues can lead to quicker regulatory actions (label changes, warnings) that protect patients and maintain trust in new drugs. The FDA has interest in these AI tools to complement its own surveillance systems. Additionally, in manufacturing (CMC review), companies using AI to control manufacturing processes can ensure consistent product quality, which might reduce the time needed for regulators to evaluate manufacturing changes or scale-ups. For example, if an AI-driven control system in manufacturing catches deviations in real-time, it can prevent batch failures and expedite supply availability once the drug is approved.
It should be noted that regulatory timelines (FDA review periods) are to some extent fixed by guidelines (e.g., a standard NDA review is ~10 months). AI cannot magically force the FDA to approve a drug faster than mandated, but it can increase the probability of first-cycle approval (i.e. approval without additional data requests or re-submissions). By submitting a more complete and well-analyzed application – potentially with AI insights strengthening the case – a sponsor might avoid the dreaded “complete response letter” that asks for more information and can add years of delay. In that sense, AI’s impact at the regulatory stage is about quality and confidence: better evidence, better understood. We are already seeing regulators proactively prepare: the multi-center involvement in FDA’s 2025 AI guidance (including the Office of New Drugs, Oncology Center, etc.) (FDA Issues Draft Guidance on the Role of Artificial Intelligence in Supporting Regulatory Decisions) indicates broad buy-in. The FDA is also recruiting data scientists and creating infrastructure to handle AI-derived data. All of this bodes well for the U.S. market, as regulatory acceptance is a key enabler for AI-driven pharma innovation.
In summary, while the direct time saved in the regulatory review might be subtle (e.g., shaving weeks off an analysis here or there), the indirect effects are significant. Clear FDA guidance reduces uncertainty (so companies can use AI without fear of regulatory rejection), and AI can ensure a smoother approval process by reducing errors and strengthening evidence. The result should be fewer delays between finishing clinical trials and obtaining FDA approval, meaning patients get new treatments sooner. The FDA’s stance can be summed up by Califf’s comment: with an agile, risk-based framework and appropriate safeguards, AI can indeed accelerate development to improve patient care (FDA Issues Draft Guidance on the Role of Artificial Intelligence in Supporting Regulatory Decisions). Both industry and regulators in the U.S. are now actively aligning to harness AI for faster drug development while upholding high standards of safety and efficacy.
Case Studies: AI-Accelerated Drug Development in Action
To concretize the impact of AI, this section highlights several real-world case studies where AI methods have successfully accelerated the drug development timeline. These examples, summarized in Table 2, span different stages of the pipeline – from discovery to clinical testing – and illustrate the diverse ways AI is shortening time to market:
Drug / Project | Organization(s) | AI Application | Outcome / Time Acceleration |
---|---|---|---|
ISM001-055 (IPF fibrotic drug) | Insilico Medicine (USA/HK) | De novo AI-led drug discovery (target identification + generative chemistry) | From hypothesis to IND candidate in ~18 months (Insilico Medicine provides benchmark timelines from its 22 AI-designed drug candidates), at ~10% the cost of traditional discovery (Insilico: linking target discovery and generative chemistry AI platforms for a drug discovery breakthrough). Entered Phase I in 2021 and showed positive Phase IIa results by late 2023 (Insilico Medicine provides benchmark timelines from its 22 AI-designed drug candidates), demonstrating a much faster preclinical-to-clinic transition than usual (benchmark ~4–5 years). |
DSP-1181 (OCD drug) | Exscientia & Sumitomo Dainippon (UK/Japan) | AI-designed small molecule (centrally acting agent for OCD) | Identified clinical candidate in just 12 months (Exscientia claims world first as AI-created drug enters clinic-pharmaphorum) using AI-driven design (vs ~5 years normally). Required synthesizing only ~350 compounds to find a potent lead (Exscientia claims world first as AI-created drug enters clinic-pharmaphorum) (vs thousands traditionally), accelerating project start. First AI-created drug to enter Phase I trials (2020) (Exscientia claims world first as AI-created drug enters clinic-pharmaphorum), heralded as a milestone in speed. |
Baricitinib for COVID-19 (repurposing) | BenevolentAI & Eli Lilly (USA) | AI knowledge graph for drug repurposing | Discovered in 48 hours that baricitinib (an existing arthritis drug) could treat COVID-19 (FDA Converts Emergency Approval Of Baricitinib — First Identified As A COVID Treatment By BenevolentAI — To A Full Approval-BenevolentAI (AMS: BAI)). Prediction published Feb 2020; by Nov 2020 the drug received FDA Emergency Use Authorization (FDA Converts Emergency Approval Of Baricitinib — First Identified As A COVID Treatment By BenevolentAI — To A Full Approval-BenevolentAI (AMS: BAI)) – compressing what could have been a multi-year development into months. First AI-driven repurposed therapy widely used in a pandemic setting. |
Adaptive Oncology Trials (Immunotherapy) | AstraZeneca & Immunai (USA) | AI-guided clinical trial optimization (dose finding, biomarker-based patient stratification) | Implemented AI in immuno-oncology trial design; achieved ~25% reduction in trial duration (Global AI In Clinical Trials Market Trends Current Partnerships) by identifying optimal dose and patient subgroups faster. Demonstrated that AI can cut down Phase I/II timelines in cancer trials (which often take years) by enabling smarter, data-adaptive protocols. |
Table 2. Representative case studies of AI accelerating drug development. These examples underscore tangible time savings: an AI-originated molecule reaching human trials in 1–2 years (vs 5+ years), an existing drug repurposed for a new disease in mere months, and an ongoing trial sped up by double-digit percentage through AI-driven efficiencies. Notably, the Insilico and Exscientia cases are pioneering instances of end-to-end AI-assisted discovery for new chemical entities, while the BenevolentAI case highlights AI’s agility in emergent situations (epidemics). The AstraZeneca example shows that even in later-stage development, AI can shave off significant time within a trial’s conduct.
Beyond these cases, the industry-wide adoption of AI is accelerating. As of early 2024, at least 31 AI-discovered drug candidates were in human trials across leading AI-driven biotech companies (How successful are AI-discovered drugs in clinical trials? A first…), and this number is growing rapidly. A report by Deloitte noted an “exponential increase” in AI-discovered drugs advancing in the pipeline, with dozens now in Phase II or III by 2023, whereas there were virtually none a few years prior (AI drug discovery: assessing the first AI-designed drug candidates for humans-CAS). Pharma companies large and small are racing to integrate AI into R&D – partnerships between traditional pharma and AI tech firms increased ~30% from 2022 to 2024 (Global AI In Clinical Trials Market Trends Current Partnerships). Examples include Pfizer partnering with AI startups for immuno-oncology, Sanofi investing $180M in an AI collaboration (with Owkin) to identify cancer biomarkers (Global AI In Clinical Trials Market Trends Current Partnerships), and Merck working with DeepMind on protein-folding AI to aid drug design (Global AI In Clinical Trials Market Trends Current Partnerships). Table 2’s AstraZeneca case is one of many such partnerships (AZ also works with tech companies like Tempus for AI in trials). This momentum indicates a broad validation: AI is moving beyond pilot projects to becoming a mainstream component of drug development strategy. The U.S. market, in particular, has seen heavy investment – collectively, 800+ AI-driven pharma startups had raised over $59 billion by 2022 to fuel this innovation (Global AI In Clinical Trials Market Trends Current Partnerships).
The case studies also illustrate that AI’s benefits are not confined to one disease area or modality – successes span small molecule drugs, biologics (gene therapy), and drug repurposing, across diseases from fibrosis to COVID-19 to cancer. Each positive result builds confidence and know-how for the next. Importantly, these faster timelines did not cut corners on safety or rigor; rather, they reflect doing the same necessary work (finding a molecule, testing it, running trials) but far more efficiently, guided by AI at critical junctures.
Limitations and Challenges of AI in Pharma R&D
While the promise of AI in accelerating drug development is great, it is equally important to acknowledge the limitations, challenges, and risks that come with its deployment. Successful case studies often make headlines, but behind the scenes, integrating AI into the highly regulated, complex process of drug R&D is not trivial. Below we discuss some key challenges and considerations:
-
Data Quality and Bias: AI models are only as good as the data they are trained on. Drug development data (whether biological assays, clinical data, or real-world health records) can be noisy, heterogeneous, and biased. If an AI system learns from historical data that under-represents certain populations (e.g. minorities in clinical trials) or is skewed towards past “successes,” it may carry forward those biases. This could lead to AI models that inadvertently exacerbate health inequalities or overlook drug targets relevant to underrepresented groups (Navigating The Pitfalls Of AI In Clinical Drug Development) (Navigating The Pitfalls Of AI In Clinical Drug Development). For example, an AI trained mostly on data from male patients might be less accurate for female patients. Ensuring diverse, high-quality training data and applying techniques to detect and mitigate bias are crucial. The industry is becoming aware of this – bias in medical AI is a well-acknowledged issue, and researchers emphasize the need for representative datasets and careful validation (Navigating The Pitfalls Of AI In Clinical Drug Development). In sum, garbage in, garbage out: without robust data governance, AI could make wrong recommendations, leading to wasted time or even unsafe outcomes if not caught.
-
The “Black Box” Problem (Explainability): Many AI algorithms, particularly deep learning models, operate as complex black boxes that don’t readily explain why they made a given prediction. In drug development, this lack of transparency can be problematic. Scientists and regulators are understandably cautious about trusting a decision if they don’t understand the rationale – for instance, why an AI chose a particular molecular structure as a candidate. This opacity of AI models can lead to trust issues and regulatory skepticism (Navigating The Pitfalls Of AI In Clinical Drug Development). If an AI suggests a compound that’s chemically novel, humans need to rationalize it with known science to be comfortable moving it forward. Furthermore, a lack of explainability can mask errors – an AI might latch onto spurious correlations in data that happen to work for the training set but are not truly causal (leading to failures later). This “black box dilemma” means AI recommendations require human oversight and validation at each step. Regulators like the FDA, in their guidance, stress that sponsors should provide details on AI model design and performance (AI Drug Development: FDA Releases Draft Guidance-Foley & Lardner LLP) (AI Drug Development: FDA Releases Draft Guidance-Foley & Lardner LLP), essentially forcing a degree of transparency. Research is ongoing into more interpretable AI models or methods to extract explanations (e.g. identifying which molecular features led the AI to score a compound highly). Until AI can clearly explain itself, it will be used as a supportive tool rather than an autonomous decision-maker in critical R&D decisions.
-
Over-Reliance and Automation vs. Human Expertise: AI is powerful, but it cannot replace human scientists and clinicians. A potential pitfall is over-reliance on AI automation without adequate human judgment – this could result in missed errors or ethical lapses. As one commentary put it, you need a “Human-in-the-Loop” approach to balance automation with expert insight (Navigating The Pitfalls Of AI In Clinical Drug Development). Human oversight is essential to catch AI’s mistakes (e.g., a data anomaly that confuses the model) and to provide context that algorithms might lack. For instance, AI might suggest a trial design that is statistically efficient but clinically impractical or not patient-friendly – humans must moderate such suggestions. Additionally, certain aspects of drug development require intuition and creativity beyond current AI capabilities, such as hypothesizing novel biological mechanisms or empathizing with patient needs. The best outcomes likely arise from human-AI collaboration, not AI working in isolation. Organizations implementing AI are advised to maintain strong governance and oversight frameworks. As the ThoughtSphere authors noted, completely hands-off automation can lead to “missed safety issues and diminished data quality” (Navigating The Pitfalls Of AI In Clinical Drug Development). The solution is to clearly define where AI aids decision-making and where humans must take the reins (especially in final safety evaluations or ethical judgments).
-
Validation and Regulatory Hurdles: Introducing AI models into a regulated process means extra work to validate those models. Companies must demonstrate that an AI tool is “fit for purpose” – for example, if an AI is used to select trial patients, the sponsor should show evidence that this improves trial outcomes and doesn’t inadvertently exclude important patient groups. The FDA’s draft guidance in 2025 lays out expectations for documentation of AI model development, performance metrics, and ongoing monitoring (AI Drug Development: FDA Releases Draft Guidance-Foley & Lardner LLP) (AI Drug Development: FDA Releases Draft Guidance-Foley & Lardner LLP). Meeting these requirements is non-trivial; it requires new skill sets (data science, software validation) and can slow things down in the short term as processes mature. There is a risk that if an AI model is not well validated, regulators could discount any data associated with it, negating the time advantage. Thus, rigorous verification and validation (V&V) of AI algorithms is now a necessary part of the timeline when using AI – and this must be planned and resourced accordingly. Companies also face the challenge of protecting their intellectual property: they might consider an AI algorithm proprietary, but to get a drug approved they may have to disclose significant details about it to regulators (AI Drug Development: FDA Releases Draft Guidance-Foley & Lardner LLP) (AI Drug Development: FDA Releases Draft Guidance-Foley & Lardner LLP), which could tip off competitors or compromise trade secrets. Balancing transparency for credibility with IP protection is a new dilemma in the AI era.
-
False Discoveries and “Hallucinations”: Particularly with generative AI (like algorithms that invent new molecules or hypothesize new targets), there is a phenomenon known as AI “hallucination” – producing outputs that look plausible but are not grounded in reality. A generative model might propose a molecular structure that is predicted to bind a target well in silico, but in reality that molecule might be impossible to synthesize or chemically unstable. Or an AI might find a pattern in patient data suggesting a drug works for a subgroup, when in truth it’s a random fluke. Without careful vetting, these AI-proposed mirages could send researchers down unproductive paths, costing time. The industry has to incorporate steps to experimentally confirm or sanity-check AI outputs at intermediate stages. In other words, AI suggestions must be stress-tested with real-world science. This is part of ensuring that speed doesn’t come at the expense of rigor. The worst-case scenario would be an AI-recommended drug that advanced quickly into trials but later was found to have a dire safety issue that earlier human scrutiny might have caught. Thus caution and tiered validation at every milestone remain essential, even if AI made the earlier steps faster.
-
Cultural and Workforce Challenges: Pharma R&D has traditionally been the domain of bench scientists, clinicians, and statisticians. Integrating AI requires cross-disciplinary collaboration with data scientists, software engineers, and AI ethicists. Companies may struggle with internal resistance or lack of expertise to implement AI solutions. Upskilling the workforce and fostering a culture that is both data-driven and innovation-friendly is a work in progress. The human change management aspect can be as challenging as the technical integration. The IT professionals in pharma (likely the readers of this report) often play a crucial role as bridges between the new AI systems and the end-user scientists, ensuring the tools are user-friendly and actually solve the problems at hand.
-
Ethical and Privacy Concerns: AI often relies on large datasets, some of which include sensitive patient information. Ensuring patient privacy (compliance with HIPAA and other regulations) when using AI on electronic health records or genomic data is paramount. Techniques like data anonymization and federated learning (where AI models learn from data without that data leaving secure repositories) are being explored to mitigate privacy risks. Ethically, there is also the question of how to handle AI-driven findings – for instance, if an AI finds that a drug only works for a certain genetic subgroup, how do we ensure fairness in drug development so that other groups aren’t neglected? The FDA and industry are aware of these concerns, and guidelines are being shaped to address them (for example, ensuring data-sharing for AI is done with privacy-enhancing techniques (AI Can Transform Drug Development, New ITIF Report Finds-ITIF) as recommended by ITIF).
In light of these challenges, it is clear that AI is not a panacea or “magic wand” that instantly fixes drug development timelines. Rather, it is a powerful set of tools that, if used wisely, can yield substantial improvements – but it must be integrated carefully and responsibly into an already complex process. Many experts emphasize a balanced view: AI can augment human expertise but not replace it, and initial hype must be tempered with scientific rigor. As one venture capitalist noted, it was unrealistic to think AI would give 100% success rates in trials; standard pipeline risks and failures will still occur (AI-Generated Drug Candidates Are Here, But Clinical Trial Wins Need More Time - MedCity News). The goal should be to use AI to reduce those risks and speed up learning cycles, not to assume we can avoid all failures. In practice, even an AI-accelerated program might fail in Phase III due to unforeseen issues – but if AI helped identify that failure risk earlier (say in Phase II) and the program stops sooner, that is still a form of acceleration (failing fast can save years of chasing a doomed project).
Finally, there is a need for continued evaluation and evidence collection on AI’s impact. The industry is starting to gather statistics on how AI-aided projects compare to traditional ones. Thus far, the signs (like the Phase I success rates, faster discovery times, etc.) are encouraging (The convergence of AI technologies and human expertise in pharma R&D) (Exscientia claims world first as AI-created drug enters clinic-pharmaphorum). However, skeptics rightly point out that many AI-success stories are isolated or early-stage – the true test will be when an AI-designed drug not only reaches market, but does so demonstrably faster than it would have under a traditional paradigm, and benefits patients. We expect those proofs to emerge in the coming years, but until then, a cautious optimism is warranted.
Conclusion and Future Outlook
Artificial intelligence is ushering in a new era for pharmaceutical development – one where data-driven algorithms work hand-in-hand with human expertise to dramatically speed up the journey from lab discovery to market launch. In this report, we examined how AI is impacting each stage of the drug development pipeline in the United States, bringing forth measurable reductions in time-to-market: from AI systems that identify drug candidates in months rather than years (Exscientia claims world first as AI-created drug enters clinic-pharmaphorum), to machine learning that streamlines clinical trials and cuts their duration by double-digit percentages (Global AI In Clinical Trials Market Trends Current Partnerships), to AI-assisted regulatory processes aimed at expediting approvals (FDA Issues Draft Guidance on the Role of Artificial Intelligence in Supporting Regulatory Decisions). For an industry where a single year’s delay can mean a patent lifetime lost or patients left waiting for treatments, these gains are game-changing.
Key takeaways include: AI-driven discovery has produced clinical candidates in ~50% less time than traditional methods in certain cases (AI Can Transform Drug Development, New ITIF Report Finds-ITIF) (Exscientia claims world first as AI-created drug enters clinic-pharmaphorum); AI-enabled trials are becoming leaner and more efficient, with projections of widespread adoption by 2030 leading to billions in savings and faster results (Global AI In Clinical Trials Market Trends Current Partnerships); and regulators like the FDA are actively encouraging responsible use of AI to maintain rigor while reaping innovation’s benefits (FDA Issues Draft Guidance on the Role of Artificial Intelligence in Supporting Regulatory Decisions). Notably, the U.S. regulatory environment – through initiatives like the FDA’s 2025 draft guidance and the 2022 Modernization Act – is adapting to facilitate AI’s integration, which is crucial for the U.S. pharma industry to fully leverage these tools (FDA to phase out animal testing with AI and lab-based models - Clinical Trials Arena) (FDA Issues Draft Guidance on the Role of Artificial Intelligence in Supporting Regulatory Decisions). The alignment of technological capability with regulatory acceptance suggests that the American market will see more AI-enhanced drug programs moving forward under clearer guidelines.
However, the future is not without caveats. We have highlighted that AI is a powerful accelerator, but not a guarantee. Challenges around data, transparency, and trust must be managed. It is encouraging that stakeholders are addressing these: companies are investing in data quality and model validation, and regulators are seeking a balance between innovation and safety oversight. In essence, the industry is learning how to move fast without breaking things – speeding up science, but with eyes open to the risks. Ethical and equitable deployment of AI will remain a focal point, ensuring that faster drug development also means better drug development for all patients.
Looking ahead, what might drug development in the U.S. look like in, say, 5–10 years if current trends continue? We can envision a scenario where AI is deeply ingrained in R&D workflows: every project starts with AI mining of disease data to propose targets; chemists use generative AI to design candidate molecules, which are optimized in autonomous labs; preclinical testing relies heavily on in silico models and AI-analyzed organ-on-chip studies; clinical trials are smaller, adaptive, and enriched with the “right” patients thanks to AI screening, and perhaps even partly augmented with digital twin simulations to reduce the number of human subjects needed. By the time a drug reaches submission, a lot of the risk and uncertainty has been wrung out by AI-driven analysis, making FDA review smoother – maybe even supplemented by AI that helps FDA reviewers check the massive data sets. This is the vision of a more efficient, data-centric drug development paradigm.
Some of this is already happening in bits and pieces; the coming decade will be about scaling it up and knitting it together. For IT professionals in pharma, this means their roles will be ever more critical: managing big data infrastructure, ensuring cybersecurity for sensitive health data, implementing AI platforms, and bridging communication between AI technologists and pharma scientists. A technically literate, IT-savvy pharma workforce will be a linchpin in realizing AI’s potential.
In conclusion, AI has demonstrated the ability to accelerate the timeline of bringing a drug to market in the U.S. through enhancements in discovery, development, and delivery. The case for optimism is strong – life-saving therapies can reach patients faster than previously thought possible, as long as we continue to innovate carefully and intelligently. As one expert noted, AI won’t make drug development 100% successful or instantaneous, but it will “undoubtedly have a positive impact on patients’ lives” by unlocking faster and smarter ways to innovate (AI-Generated Drug Candidates Are Here, But Clinical Trial Wins Need More Time - MedCity News). The convergence of AI technology with human scientific acumen is poised to transform pharmaceutical R&D from a slow marathon into a more streamlined, agile process. The U.S. pharmaceutical industry stands at the forefront of this transformation, and the coming years will likely solidify AI’s role as an indispensable catalyst in delivering new medicines to the world.
Sources: All information in this report is drawn from reputable sources, including peer-reviewed journals, industry reports, and guidance from regulatory authorities. Key references include the Information Technology and Innovation Foundation’s 2024 report on AI in drug development (AI Can Transform Drug Development, New ITIF Report Finds-ITIF) (AI Can Transform Drug Development, New ITIF Report Finds-ITIF), FDA announcements and guidances on AI in drug development (FDA Issues Draft Guidance on the Role of Artificial Intelligence in Supporting Regulatory Decisions) (FDA Issues Draft Guidance on the Role of Artificial Intelligence in Supporting Regulatory Decisions), recent case studies from FierceBiotech and Nature Biotechnology on AI-discovered drugs (Insilico Medicine provides benchmark timelines from its 22 AI-designed drug candidates) (Insilico Medicine provides benchmark timelines from its 22 AI-designed drug candidates), and up-to-date industry analyses on AI’s impact on clinical trials and success rates (Global AI In Clinical Trials Market Trends Current Partnerships) (The convergence of AI technologies and human expertise in pharma R&D), among others cited throughout. These demonstrate the current state of AI in pharma (as of 2024–2025) and provide the evidence base for the trends discussed.
DISCLAIMER
The information contained in this document is provided for educational and informational purposes only. We make no representations or warranties of any kind, express or implied, about the completeness, accuracy, reliability, suitability, or availability of the information contained herein. Any reliance you place on such information is strictly at your own risk. In no event will IntuitionLabs.ai or its representatives be liable for any loss or damage including without limitation, indirect or consequential loss or damage, or any loss or damage whatsoever arising from the use of information presented in this document. This document may contain content generated with the assistance of artificial intelligence technologies. AI-generated content may contain errors, omissions, or inaccuracies. Readers are advised to independently verify any critical information before acting upon it. All product names, logos, brands, trademarks, and registered trademarks mentioned in this document are the property of their respective owners. All company, product, and service names used in this document are for identification purposes only. Use of these names, logos, trademarks, and brands does not imply endorsement by the respective trademark holders. IntuitionLabs.ai is an AI software development company specializing in helping life-science companies implement and leverage artificial intelligence solutions. Founded in 2023 by Adrien Laurent and based in San Jose, California. This document does not constitute professional or legal advice. For specific guidance related to your business needs, please consult with appropriate qualified professionals.